Content from Python Scripts and Modules
Last updated on 2024-04-16 | Edit this page
Estimated time: 22 minutes
Overview
Questions
- What is a ‘module’ in Python? How does this differ from a ‘script’?
- What are the benefits of modularising our Python code?
Objectives
- Introduce the example library used in this lesson.
- Understand the limitations of scripts.
- Understand what happens when we
import
something. - Learn how to convert a simple script into a reusable module.
Python as a Scripting Language
Python is frequently used as a scripting language by scientists and engineers due to its expressiveness, simplicity, and its rich ecosystem of third-party libraries. There isn’t a hard line between a scripting language and a non-scripting language, but some differences include:
- Scripts are not compiled to an executable or library object (such as
.so
or.dll
) before use, and are instead interpreted directly from the source code. A non-scripting language, such as C++, must be compiled to machine code before it can be used. - Scripts must be run using some other compiled program, such as
python
orbash
. - Scripts are typically short programs that leverage the power of complex libraries to accomplish some task. They focus more on gluing together existing tools than performing their own low-level computations.
Python is a very general-purpose language, and it meets the criteria of both a scripting language and a non-scripting language depending on how we choose to use it:
- Python can be run as an interactive session simply by calling
python
at the command line. This is typical of a scripting language. - It is possible to write a linear series of Python expressions in a file, and run this using the Python interpretter –again, like a scripting language.
- Python code can be bundled in modules and packages
which can then be
import
-ed into other Python programs/scripts. These are typically pre-compiled into ‘bytecode’ to improve performance. This is closer to the behaviour of a fully compiled language.
Typically, a Python project will begin as something that is inarguably a ‘script’, but as it develops it will take on the character of a ‘program’. A single file containing a linear series of commands may develop into a module of reusable functions, and this may develop further into a package of related modules. Developing our software in this way allows our programs to grow in complexity in a much more sustainable manner, and grants much more flexibility to how users can interact with our code.
Throughout this course, we’ll develop an example library that might be used for epidemiology modelling, though it isn’t necessary to understand how this works in order to follow the course. We’ll begin where many new Python projects start: with a simple script. This one solves a SIR model, which models the number of Susceptible, Infected, and Recovered individuals as a pathogen spreads through a population. The general pattern of the code – set up inputs, solve a problem, plot the results – should be familiar to those working in a data-oriented field. It uses the popular plotting library Matplotlib to generate a figure.
It isn’t necessary to dwell on the details of the script. It’s only being used as an example of the sort of script the students might be familiar with.
PYTHON
import matplotlib.pyplot as plt
# Inputs
# Total number of susceptible people at the start of an outbreak
pop_size = 8000000
# Average no. of new infections per infected person per day
beta = 0.5
# Inverse of the average number of days taken to recover
gamma = 0.1
# Number of days to run the simulation for
days = 150
# Number of infected people at the start of the simulation
I_0 = 10
# Initialise data
S = [] # Number of susceptible people each day
I = [] # Number of infected people each day
R = [] # Number of recovered people each day
S.append(pop_size - I_0)
I.append(I_0)
R.append(0)
# Solve model
for i in range(days):
# Get rate of change of S, I, and R
dS = - beta * S[i] * I[i] / pop_size
dR = gamma * I[i]
dI = -(dS + dR)
# Get values on next day
S.append(S[i] + dS)
I.append(I[i] + dI)
R.append(R[i] + dR)
# Plot results
plt.plot(range(len(S)), S, label="S")
plt.plot(range(len(I)), I, label="I")
plt.plot(range(len(R)), R, label="R")
plt.xlabel("Time /days")
plt.ylabel("Population")
plt.legend()
plt.show()
If we save this script to the file SIR_model_script.py
,
it can then be run from the command line as follows:
If everything works as expected, it should produce the following result:
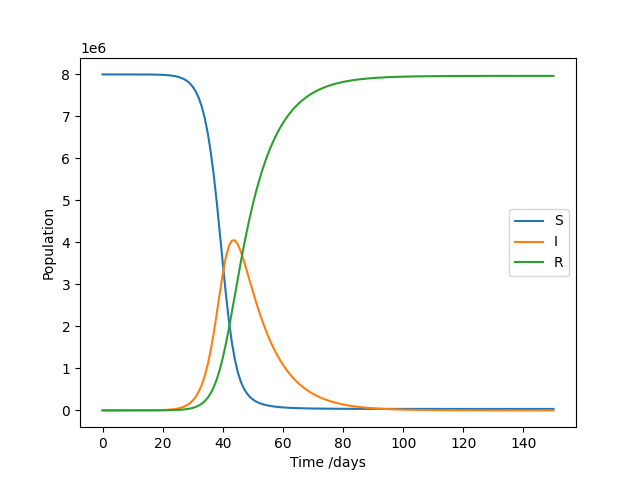
So far so good, but there are some limitations to this coding style.
There are many possible answers to this. Here are some examples:
- If the user wants to change the model inputs, they have to open the script file and modify some lines of code before running again. This is difficult to automate and it’s possible that the user might overwrite something they didn’t intend to.
- If we wish to expand on this functionality or do something different with the output data, we must either overwrite our original script, or copy the code across to a new scipt. If we choose to copy the script, it would take a lot of effort to update all versions every time we wish to adjust the core routine, and this is likely to introduce bugs.
- The user must know where the script is stored on their system, navigate to that directory, and call it from there. This makes it challenging to string scripts together to make more complex programs.
It’s important to note that there’s nothing strictly wrong
with writing scripts in this way, and it’s often a good starting point
for a new project. However, as we develop our project further, we might
find it useful to bundle up the reusable bits of our code into a
module that we can import
from other Python
programs, just as we import
-ed Matplotlib in our own
script.
What is a ‘Module’?
A module is simply any file containing Python statements,
so, technically, the script we defined above is already a module. The
name of a module is the same as the file name, only without the
.py
extension. If we were to open a new Python interpretter
session and call the following:
then the script would run as if we’d run it from the command line.
However, the variables we defined in the script will be accessible
within the namespace SIR_model_script
:
The current namespace includes all of the objects defined in our
interpretter session, plus all built-in Python functions and types. When
we import
our script, we add the name of the script to the
current namespace, but not the variables defined within it; those are
defined within the scope of SIR_model_script
, and
are only accessible using the dot operator. If we wanted to bring
everything within the script to the current namespace, but not the
module itself, we can instead call:
Alternatively, if we only want a few objects brought into the current namespace, we can use:
The dangers offrom module import *
Using from module import *
is considered harmful by many
developers, as objects can be unintentionally overwritten if there are
any name clashes between modules. It can also make it difficult for
people reading you code to tell which modules your functions and classes
have come from. In general, it’s better to be explicit about what you’re
import
-ing.
It is also possible to assign an alias to a module name using the
as
keyword:
Something to note about import
is that it runs the code
found within the module only the first time it is imported, so if we
were to import our script multiple times, it would only create a plot
once.
While we’ve shown that our script is importable, so far it doesn’t seem to provide many advantages over simply running it on the command line. The next section will explain what features can make a module more versatile and useful.
The result is 15
, as the second import does not run the
code in my_module.py
, and therefore
my_module.x
is not reset back to 10
.
Making a Reusable Module
A good Python module will tend to do the following:
- Define functions, classes and constants that the user might want to use.
- Not rely on global variables (those defined within the module but not inside of functions/classes), except in a few scenarios where it only makes sense for a single instance of the an object to exist.
- Avoid performing runtime calculations at the
import
stage.
Most Python modules shouldn’t do anything if we try to run them from
the command line, and instead they should provide
import
-able tools that can be used in the Python
interpretter, by other Python modules, or by dedicated scripts. We’ll
see later how to write a reusable Python module that doubles as a
runnable script.
Most scripts can be converted into reusable modules using the following steps:
- Identify each major stage of our data processing. Examples may include reading in data, running a model, processing results, creating plots, etc.
- For each stage, identify what data is an input, and what is an output.
- Bundle each processing stage into a function that takes the input data as arguments and returns the output data.
For example, the script SIR_model_script
has two stages
that can be bundled into functions. The first stage runs the SIR model,
and it takes the following input parameters:
Parameter | Type | Description |
---|---|---|
pop_size |
int |
Total number of susceptible people at the start of an outbreak |
beta |
float |
Average no. of new infections per infected person per day |
gamma |
float |
Inverse of the average number of days taken to recover |
days |
int |
Number of days to run the simulation for |
I_0 |
int |
Number of infected people at the start of the simulation |
The output data from this stage is:
Parameter | Type | Description |
---|---|---|
S |
List[float] |
Number of susceptible people each day |
I |
List[float] |
Number of infected people each day |
R |
List[float] |
Number of recovered people each day |
We can therefore bundle this portion of the script into the function
SIR_model
:
PYTHON
def SIR_model(pop_size, beta, gamma, days, I_0):
"""
Solves a SIR model numerically using a simple integration scheme.
Parameters
----------
pop_size: int
Total number of susceptible people at the start of an outbreak.
beta: float
Average number of new infections per infected person per day.
gamma: float
Inverse of the average number of days taken to recover.
days: int
Number of days to run the simulation for.
I_0: int
Number of infected people at the start of the simulation.
Returns
-------
S: List[float]
Number of susceptible people on each day.
I: List[float]
Number of infected people on each day.
R: List[float]
Number of recovered people on each day.
"""
# Initialise data
S = [] # Number of susceptible people each day
I = [] # Number of infected people each day
R = [] # Number of recovered people each day
S.append(pop_size - I_0)
I.append(I_0)
R.append(0)
# Solve model
for i in range(days):
# Get rate of change of S, I, and R
dS = - beta * S[i] * I[i] / pop_size
dR = gamma * I[i]
dI = -(dS + dR)
# Get values on next day
S.append(S[i] + dS)
I.append(I[i] + dI)
R.append(R[i] + dR)
return S, I, R
Note that we’ve provided a nice docstring, so that users of our function can understand how to use it. The second stage of our script takes the results of the SIR model as input data, and produces a plot. We can therefore bundle the plotting parts of the script as follows:
PYTHON
# Note: imports should go at the top of the file
import matplotlib.pyplot as plt
def plot_SIR_model(S, I, R):
"""
Plot the results of a SIR simulation.
Parameters
----------
S: List[float]
Number of susceptible people on each day.
I: List[float]
Number of infected people on each day.
R: List[float]
Number of recovered people on each day.
Returns
-------
None
"""
plt.plot(range(len(S)), S, label="S")
plt.plot(range(len(I)), I, label="I")
plt.plot(range(len(R)), R, label="R")
plt.xlabel("Time /days")
plt.ylabel("Population")
plt.legend()
plt.show()
If we save the two code blocks above to a file called
SIR_model.py
, we can then open up a Python interpreter and
run the following:
PYTHON
>>> from SIR_model import SIR_model, plot_SIR_model
>>> S, I, R = SIR_model(
pop_size=8000000, beta=0.5, gamma=0.1, days=150, I_0=10
)
>>> plot_SIR_model(S, I, R)
This should give us the same results as when we ran the script
earlier, and we can run the SIR_model
function with
different inputs without needing to change the input parameters in the
file itself. The functions in our script are now therefore ‘reusable’,
and can be integrated in other workflows. The nice docstrings we added
can be viewed using the built-in help()
function:
However, the script-like behaviour has been lost:
In the next section, we’ll show how to maintain script-like behaviour, and write reusable modules in the same file.
Maintaining Script-Like Functionality
If we wish, we can also maintain the script-like behaviour using the
if __name__ == "__main__".py
idiom at the bottom of the
file SIR_model.py
. Here, we create a special
if
block at the bottom of our module, and within this we
call each of our functions in turn, using the outputs of one as inputs
to the next:
PYTHON
# file: SIR_model.py
# Add this after our function definitions
if __name__ == "__main__":
S, I, R = SIR_model(
pop_size=8000000, beta=0.5, gamma=0.1, days=150, I_0=10
)
plot_SIR_model(S, I, R)
With this is place, we can still run the module as if it were a script:
However, this section will not run if we import
the
file. Let’s break down how this works:
- Each Python module is automatically assigned a variable
__name__
, and this is usually set to the name of the file without the.py
extension.
OUTPUT
"SIR_model"
- The exception to the rule is when we run a Python module as a
script. In this case, the top-level file instead has its
__name__
variable set to"__main__"
.
Therefore, the code written under
if __name__ == "__main__"
will run if we use the module as
a script, but not if we import
the file.
It prints "__main__"
It prints "name_test"
As we’ll see later, it can also be handy to bundle the contents of
our if __name__ == "__main__"
block into a function, as
then we can import
that function and access our script-like
behaviour in another way. This function can take any name, but is often
called main
:
PYTHON
# file: SIR_model.py
def main():
S, I, R = SIR_model(
pop_size=8000000, beta=0.5, gamma=0.1, days=150, I_0=10
)
plot_SIR_model(S, I, R)
if __name__ == "__main__":
main()
We’ll use this format later when discussing ways to make runnable packages.
Managing PYTHONPATH
Our script is now import
-able, so the
SIR_model
function can be used from any other Python
script, module, or interpretter session. However, we still need to know
where the module is stored on our file system, which can make it
difficult to reuse the functions in a separate project. A simple
solution is to set the PYTHONPATH
environment variable on
our system. On Linux machines, this can be achieved using:
If you want this to be set every time you open a terminal, you can
add the line to the file ~/.bashrc
.
However, this is not recommended as a long-term solution, as a custom
PYTHONPATH
can cause dependency conflicts between different
packages on our system and can be difficult to debug. In a later
chapter, we will show how to install our own modules using the
pip
package manager, which gives us much more control over
how we integrate our modules into our Python environments (which may be
managed using tools such as venv
or conda
),
and also allows us to install packages from a remote repository. We’ll
also show how to upload our own packages to the remote repository PyPI,
and therefore allow others to download and install our code from the
command line!
Extra: Better Automation with Matplotlib
Earlier, we converted the plotting section of our script into a function that the user can call. There are two issues with the implementation as it stands:
- The use of
plt.show()
interrupts the flow of the program and requires the user to manually save the figure or discard it. This makes it difficult to automate the production of figures. - As it calls
plt.plot()
without first creating a new figure, it may interfere with our user’s Matplotlib code.
We can improve the function with a few changes:
- Rather than using Matplotlib’s ‘implicit’ API (such as by using
plt.plot()
), which manages global Matplotlib objects, use the ‘explicit’ API, sometimes called the ‘object-oriented’ API. This requires handlingFigure
andAxes
objects directly. - Optionally take in an
Axes
object. This way, the user can choose to set up their ownFigure
andAxes
, and our function can write to it. - Return the
Axes
object that we worked on, so that the user can make further changes if they wish. - Only use
plt.show()
if the user requests it. Also provide an option to save the figure.
Here is an example of an improved function:
PYTHON
import matplotlib.pyplot as plt
def plot_SIR_model(S, I, R, ax=None, save_to=None, show=False):
"""
Plot the results of a SIR simulation.
Parameters
----------
S: List[float]
Number of susceptible people on each day.
I: List[float]
Number of infected people on each day.
R: List[float]
Number of recovered people on each day.
ax: Optional[plt.Axes], default None
Axes object on which to create the plot.
A new Axes is created if this is None.
save_to: Optional[str], default None
Name of the file to save the plot to.
Does not save if None.
show: bool, default False
If True, call plt.show() on completion.
Returns
-------
plt.Axes
The axes object the results have been plotted to.
"""
# Use plt.subplots to create Figure and Axes objects
# if one is not provided.
if ax is None:
fig, ax = plt.subplots()
# Create plot
ax.plot(range(len(S)), S, label="S")
ax.plot(range(len(I)), I, label="I")
ax.plot(range(len(R)), R, label="R")
ax.set_xlabel("Time /days")
ax.set_ylabel("Population")
ax.legend()
# Optionally save the figure
if save_to is not None:
fig = ax.get_figure()
fig.savefig(save_to)
# Optionally show the figure
if show:
plt.show()
return ax
This gives our users much more control over how to create plots, and it allows our function to be used as part of a larger automated pipeline that runs without needing human input. There are many further ways we could improve this function and allow our users to finely control what’s plotted, such as by allowing the user to overwrite axis/line labels or interact with legend placement, but we’ll move on from this topic for now.
Extra: Better scripting with argparse
We showed earlier how to maintain script-like functionality in our modules. We’ll update that code to include our updated plotting function, so we’ll automatically save to a file if the user runs our script:
PYTHON
# file: SIR_model.py
def main():
S, I, R = SIR_model(
pop_size=8000000, beta=0.5, gamma=0.1, days=150, I_0=10
)
plot_SIR_model(S, I, R, save_to="SIR_model.png")
if __name__ == "__main__":
main()
An issue with our example is that it still requires the user to
manually edit the file if they wish to change the input or outputs. This
problem can be solved by instead taking arguments from the command line.
A simple interface can be created using sys.argv
, which is
a list of command line arguments in the form of strings:
PYTHON
# file: SIR_model.py
import sys
def main():
# Note: sys.argv[0] is the name of our program!
pop_size = int(sys.argv[1])
beta = float(sys.argv[2])
gamma = float(sys.argv[3])
days = int(sys.argv[4])
I_0 = int(sys.argv[5])
output = sys.argv[6]
S, I, R = SIR_model(
pop_size=pop_size,
beta=beta,
gamma=gamma,
days=days,
I_0=I_0,
)
plot_SIR_model(S, I, R, save_to=output)
if __name__ == "__main__":
main()
However, this requires the user to provide every argument in order,
and doesn’t allow default arguments. We can achieve a better interface
using the built-in argparse
library. The comments in the
code below explain how this works:
PYTHON
# file: SIR_model.py
from argparse import ArgumentParser
def main():
# Create an argument parser object. We can provide
# some info about our program here.
parser = ArgumentParser(
prog="SIR_model",
description="Solves SIR model and creates a plot",
)
# Add each argument to the parser. We can specify
# the types of each argument. Optional arguments
# should have single character names with a hypen,
# or longer names with a double dash.
parser.add_argument(
"-p", "--pop_size", type=int, default=10000000,
help="Total population size",
)
parser.add_argument(
"-b", "--beta", type=float, default=0.5,
help="Average no. of new infections per infected person per day",
)
parser.add_argument(
"-g", "--gamma", type=float, default=0.1,
help="Inverse of average number of days taken to recover",
)
parser.add_argument(
"-d", "--days", type=int, default=150,
help="Number of days to run the simulation",
)
parser.add_argument(
"-i", "--i0", type=int, default=10,
help="Number of infected people at the start of the simulation",
)
parser.add_argument(
"-o", "--output", default="SIR_model.png",
help="Output file to save plot to",
)
# Get each argument from the command line
args = parser.parse_args()
# Run our code
S, I, R = SIR_model(
pop_size=args.pop_size,
beta=args.beta,
gamma=args.gamma,
days=args.days,
I_0=args.i0,
)
plot_SIR_model(S, I, R, save_to=args.output)
if __name__ == "__main__":
main()
We can now run our script using inputs from the command line:
We defined each option with a default, so we don’t need to provide
all of them if we don’t want to. Each option has a long form with two
dashes (--output
, --beta
), and a short form
with one dash (-o
, -b
), which are
interchangeable (if we wish, we could provide only the short form or the
long form). Note that we can use either an equals =
or a
space to separate the option name from its value.
If we had provided options without preceeding dashes, they would
become ‘positional’ arguments, and would be required. The order
positional arguments should be supplied is given by the order in which
they are added to the parser. For example, if we had added
beta
and gamma
as follows:
PYTHON
parser.add_argument(
"beta", type=float,
help="Average no. of new infections per infected person per day",
)
parser.add_argument(
"gamma", type=float,
help="Inverse of average number of days taken to recover",
)
We would need to supply these arguments in order when running our code. Positional arguments are not specified by name on the command line:
If we forget how our script is supposed to be run,
argparse
automatically provides a nice help message if we
run it with -h
or --help
:
usage: SIR_model [-h] [-p POP_SIZE] [-b BETA] [-g GAMMA] [-d DAYS] [-i I0] [-o OUTPUT]
Solves SIR model and creates a plot
optional arguments:
-h, --help show this help message and exit
-p POP_SIZE, --pop_size POP_SIZE
Total population size
-b BETA, --beta BETA Average no. of new infections per infected person per day
-g GAMMA, --gamma GAMMA
Inverse of average number of days taken to recover
-d DAYS, --days DAYS Number of days to run the simulation
-i I0, --i0 I0 Number of infected people at the start of the simulation
-o OUTPUT, --output OUTPUT
Output file to save plot to
There are many ways to control our command line interface in more
detail, such as constraining the possible user input choices, parsing
lists of inputs, and using ‘sub-parsers’ to split functionality across a
number of sub-commands, much like how pip
handles its many
utilities:
Here, install
and list
are sub-commands,
which each handle a different set of their own args. We’ll show how to
achieve this in the next lesson, where we’ll expand our code from a
single module to a collection of modules known as a ‘package’.
Key Points
- Any Python file can be considered a ‘module’, and can be
import
-ed. This just runs the code in the file, and makes the names of any objects in the module accessible using dot-notation. - If we bundle our Python scripts into a collection of functions, we can reuse those functions in other modules or in the Python interpretter.
- After turning our scripts into reusable modules, we can maintain
script-like behaviour using the idiom
if __name__ == "__main__"
. -
argparse
can be used to create sophisticated command line interfaces.
Content from From Modules to Packages
Last updated on 2024-04-16 | Edit this page
Estimated time: 20 minutes
Overview
Questions
- What is a ‘package’ in Python?
- Why should we organise our code into packages?
Objectives
- Explain how to group multiple modules into a package.
- Understand the purpose of an
__init__.py
file. - Understand how
__all__
works. - Understand the purpose of a
__main__.py
file.
Packages
In the previous lesson, we showed how to convert a simple Python script into a reusable module by bundling up different parts of the script into functions. However, as our project grows, we may find it beneficial to spread the functionality of our project over multiple files. Separating logically distinct units of code into their own files will help others to understand how our project works, and it allows us to control which features of our code are exposed to users at the top level.
The primary tool for organising a project across multiple files is the package. Packages are very similar to modules, and they are defined by the directory structure we use to organise our files.
To demonstrate how to create a package, we’ll convert our SIR model
module from the last lesson into a package. Let’s say we have it stored
within a directory epi_models
– short for epidemiology
models:
📁 epi_models
|
|____📜 SIR.py
To convert this to a package, we’ll simply create a new directory –
also called epi_models
– and move our Python module to
it:
We’ll also add a new empty file called __init__.py
:
Our directory structure should now be:
📁 epi_models
|
|____📦 epi_models
|
|____📜 __init__.py
|____📜 SIR.py
That’s all we need for a basic package! But why did we need to create
a new directory with the same name, and what is __init__.py
for?
- The top-level directory can be thought of as the project directory, while the directory contained within is the package directory. Later, we will add new files to the project directory containing descriptive metadata for our package, and this will allow us to build, install, and publish the package. It is important for these files to be kept outside of the package itself.
- The choice to give the package directory the same name as the project directory is simply a common convention for Python projects, and it’ll make it easier for to build, install and publish our package later. We’ll cover alternative directory layouts in our lesson on building and installing packages.
- The presence of an
__init__.py
file marks a directory as a package, and the contents of this file are run when the package or any modules within are imported. We’ll explain how to use this file to set up and control our package in a later section.
Namespace Packages
Since Python 3.3, if you omit __init__.py
, you may find
that the following code snippets continue to work. This is because
directories without __init__.py
act as namespace
packages, which can be used to build a package using multiple
‘portions’ spread across your system. The specification for namespace
packages can be found in PEP
420.
Unless you’re intending to create a namespace package, it is good
practice to include __init__.py
, even if you choose to
leave it empty, as this makes your intentions for the package
clearer.
Now that we’ve created a basic package, it is straightforward to expand it to contain additional modules. For example, we may choose to add new epidemiology models, such as the SEIR model which introduces a new population category of those who are Exposed to a pathogen, but not yet infectious themselves (this models diseases such as COVID-19 fairly well). We could also add a SIS model, which is similar to the SIR model, but Recovered individuals do not gain immunity to the pathogen, and instead return to the Susceptible population (this can apply to the common cold and some types of flu). These new modules should be added to the package directory:
📁 epi_models
|
|____📦 epi_models
|
|____📜 __init__.py
|____📜 SIR.py
|____📜
SEIR.py
|____📜 SIS.py
These new modules might contain similar functions to
SIR.py
. For example, SEIR.py
may contain:
PYTHON
# file: SEIR.py
def SEIR_model(pop_size, alpha, beta, gamma, days, I_0):
# Function to solve the SEIR model
# Returns lists S, E, I, R
pass
def plot_SEIR_model(S, E, I, R):
# Function to plot S, E, I, R over time
pass
We won’t worry about how these functions should be implemented for
now. SIS.py
should contain similar functions.
To import our functions, we can now enter the top-level
epi_models
directory and call the following in an
interactive session:
PYTHON
>>> import epi_models.SIR
>>> S, I, R = epi_models.SIR.SIR_model(
pop_size=8000000, beta=0.5, gamma=0.1, days=150, I_0=10
)
Having to type the package name and the module name every time can be
inconvenient, so it’s a good idea to use the alternative
import
styles we discussed in the previous lesson:
PYTHON
>>> # Assign an alias to the import
>>> import epi_models.SEIR as SEIR
>>> S, E, I, R = SEIR.SEIR_model(
pop_size=8000000, alpha=0.2, beta=0.5, gamma=0.1, days=150, I_0=10
)
>>> # Use 'from ... import ...' to get the function directly
>>> from epi_models.SEIR import SEIR_model
>>> S, E, I, R = SEIR_model(
pop_size=8000000, alpha=0.2, beta=0.5, gamma=0.1, days=150, I_0=10
)
>>> # Import everything into the current namespace (not recommended!)
>>> from epi_models.SIS import *
>>> S, E, I, R = SEIR_model(
pop_size=8000000, alpha=0.2, beta=0.5, gamma=0.1, days=150, I_0=10
)
As our project develops, we may decide that we need a further level of organisation. For example, if our modelling tools and our plotting tools become sufficently complex, we may decide to move these into their own directories. To do this, we can define sub-packages within our top-level package:
📁 epi_models
|
|____📦 epi_models
|
|____📜 __init__.py
|
|____📁 models
| |
| |____📜 __init__.py
| |____📜
SIR.py
| |____📜 SEIR.py
| |____📜 SIS.py
|
|____📁 plotting
|
|____📜
__init__.py
|____📜 plot_SIR.py
|____📜
plot_SEIR.py
|____📜 plot_SIS.py
Note that each sub-directory, and hence sub-package, requires its own
__init__.py
. With our project organised in this way, we can
now import each function using:
Relative Imports
One advantage of organising our code into packages and sub-packages
is that it allows us to import other modules using relative
imports. To show how these work, we’ll add an extra file
utils.py
inside the models
subpackage.
📁 epi_models
|
|____📦 epi_models
|
|____📜 __init__.py
|
|____📁 models
| |
| |____📜 __init__.py
| |____📜
SIR.py
| |____📜 SEIR.py
| |____📜 SIS.py
| |____📜 utils.py
|
|____📁 plotting
|
|____📜 __init__.py
|____📜
plot_SIR.py
|____📜 plot_SEIR.py
|____📜
plot_SIS.py
This could contain utility functions that may be useful within
SIR.py
, SEIR.py
, and SIS.py
. In
each of these files, we could import the function some_func
from utils.py
using the following syntax:
The dot preceding the module name instructs Python to search inside the current (sub-)package to find a module with a matching name.
We may also find it useful for our plotting tools to have access to
the modelling functions. In that case, we can add the following to
plot_SIR.py
:
Here, the double dots indicate that we should look one package higher than the current sub-package.
Note that relative imports only work within packages, so they should be avoided in our plain scripts.
The __init__.py
file
__init__.py
files do not need to contain any code to
mark a directory as a Python package, but if we wish, we can use them to
control what is or isn’t visible at package-level scope, or to perform
any additional setup.
Consider the __init__.py
file in the models
directory. Let’s add the following lines:
PYTHON
# file: epi_models/models/__init__.py
from .SIR import SIR_model
from .SEIR import SEIR_model
from .SIS import SIS_model
When SIR_model
, SEIR_model
and
SIS_model
are brought into the local namespace in
models/__init__.py
, they are brought into the namespace of
epi_models.models
too:
PYTHON
>>> from epi_models.models import SIR_model
>>> # Equivalent to:
>>> from epi_models.models.SIR import SIR_model
If we wish to expose these functions to the user at the top level
package, we can also add the following to
epi_models/__init__.py
Note that we can import these names directly from the
models
sub-package instead of going to each of the modules
in turn. It is now much easier for users to access these functions:
When writing __init__.py
files, it is important to
consider what we don’t import. Note that we did not import any
functions from utils.py
, as these are only intended for use
within the models
sub-package, and there’s no need to
expose these implementation details to the user. A well crafted
__init__.py
allows us to define a public API,
which is the portion of our code that we expect our users to interact
with. The following section introduces the __all__
variable, which allows us to more rigorously define a public API.
As the contents of __init__.py
is run whenever the
package or any sub-packages/modules are imported, we can also use
__init__.py
to perform any additional package-level setup.
For instance, it might be used to set up connection to a database used
throughout the package. In this way, __init__.py
performs a
similar role for a package as an __init__
function does for
a class.
Using __all__
to control
from module import *
__all__
is an optional variable that we may set in our
modules and packages (to assign it to a package, it should be defined in
__init__.py
). It should be a list of strings matching the
names of all objects – including functions, classes, constants and
variables – that we wish to be considered ‘public’ features that the
user may wish to use.
__all__
also changes the behaviour of
from module import *
. By default, this would import all
objects within the namespace of module
, and bring them into
the current namespace. This may not be desirable, as this may bring
utility functions/classes/variables into the current scope, including
any objects we explicitly mark as private using a preceeding underscore,
and even anything we’ve imported. If we set __all__
, only
those objects with names matching the strings contained within
__all__
will be loaded. For example, if we wrote the
following:
PYTHON
# file: epi_models/__init__.py
from .models import SIR_model, SEIR_model, SIS_model
__all__ = ["SIR_model", "SEIR_model"] # Note SIS_model is missing!
Calling the following in an interactive session would work just fine:
OUTPUT
<function SIR_model at 0x7f0290dc9150>
However, the following would fail:
OUTPUT
Traceback (most recent call last):
File "<stdin>", line 1, in <module>
NameError: name 'SIS_model' is not defined
‘Private’ variables in Python
It is common for Python programmers to mark objects as private by
prefixing their names with an underscore. For example,
_private_variable
or _PrivateClass
. For an
added layer of protection, variables set in class instances with two
underscores, such as self.__x
, will have their names
mangled when viewed from outside the class, but they will still be
locatable and modifiable by a determined individual.
Python programmers will also sometimes use a trailing underscore, and this is commonly used to avoid a name clash with a built-in object:
A combination of the private naming convention, well chosen
__all__
variables, and thorough documentation is more than
sufficient to specify a public API.
Script-like Packages and the __main__.py
file
In the previous lesson, we introduced the
if __name__ == "__main__"
idiom, and discussed how to use
this to maintain script-like behaviour in our reusable modules. The good
news is that this is still possible when we upgrade our modules to
packages. For instance, let’s say that the file
epi_models/plotting/plot_SIR
contains the following, which
excludes the enhancements added in the ‘extra’ section last lesson:
PYTHON
# file: plot_SIR.py
import matplotlib.pyplot as plt
def plot_SIR_model(S, I, R):
"""
Plot the results of a SIR simulation.
Parameters
----------
S: List[float]
Number of susceptible people on each day.
I: List[float]
Number of infected people on each day.
R: List[float]
Number of recovered people on each day.
Returns
-------
None
"""
plt.plot(range(len(S)), S, label="S")
plt.plot(range(len(I)), I, label="I")
plt.plot(range(len(R)), R, label="R")
plt.xlabel("Time /days")
plt.ylabel("Population")
plt.legend()
plt.show()
def main():
S, I, R = SIR_model(
pop_size=8000000, beta=0.5, gamma=0.1, days=150, I_0=10
)
plot_SIR_model(S, I, R)
if __name__ == "__main__":
main()
If we jump into the directory epi_models/plotting
, and
call the following, we get an error:
OUTPUT
Traceback (most recent call last):
File "plot_SIR.py", line 2, in <module>
from ..models.SIR import SIR_model
ImportError: attempted relative import with no known parent package
When running a single file as if its a script, Python will not
consider it to be part of a wider package, and hence relative imports
will fail. To solve this, we should run our script from outside the
package using the -m
flag:
Note that we use dots rather than slashes to separate the names of
each sub-package/module, and we don’t include .py
at the
end.
We can also add script-like behaviour to packages by adding a
__main__.py
file:
📁 epi_models
|
|____📦 epi_models
|
|____📜 __init__.py
|____📜 __main__.py
|
|____📁 models
| |
| |____📜
__init__.py
| |____📜 SIR.py
| |____📜
SEIR.py
| |____📜 SIS.py
| |____📜 utils.py
|
|____📁 plotting
|
|____📜
__init__.py
|____📜 plot_SIR.py
|____📜
plot_SEIR.py
|____📜 plot_SIS.py
As the module name of this file is already __main__
,
there’s no need to use the if __name__ == "__main__"
idiom,
and we may write this file as if it were a simple script. If we wish to
run the scripting interface from plot_SIR.py
, we can do so
simply by importing and calling the function main()
:
If we add a different scripting interface for each model type, we can
use sys.argv
to read the user’s model choice from the
command line:
PYTHON
# file: __main__.py
import sys
from plotting.plot_SIR import main as SIR_main
from plotting.plot_SEIR import main as SEIR_main
from plotting.plot_SIS import main as SIS_main
# Read model type from the command line
model = sys.argv[1]
# Run the chosen model
if model == "SIR":
SIR_main()
elif model == "SEIR":
SEIR_main()
elif model == "SIS":
SIS_main()
else:
raise RuntimeError(
"First cmd line arg should be 'SIR', 'SEIR', or 'SIS'"
)
If you do intend to add scripting behaviour to your packages, it is
highly recommended to read the ‘extra’ sections in this lesson and the
previous one, as argparse
allows you to build much more
sophisticated command line interfaces.
Extra: Advanced argparse
in
__main__.py
Let’s return to plot_SIR.py
, and apply the enhancements
discussed in the ‘extra’ sections last lesson:
PYTHON
import matplotlib.pyplot as plt
from argparse import ArgumentParser
from ..models.SIR import SIR_model
def plot_SIR_model(S, I, R, ax=None, save_to=None, show=False):
"""
Plot the results of a SIR simulation.
Parameters
----------
S: List[float]
Number of susceptible people on each day.
I: List[float]
Number of infected people on each day.
R: List[float]
Number of recovered people on each day.
ax: Optional[plt.Axes], default None
Axes object on which to create the plot.
A new Axes is created if this is None.
save_to: Optional[str], default None
Name of the file to save the plot to.
Does not save if None.
show: bool, default False
If True, call plt.show() on completion.
Returns
-------
plt.Axes
The axes object the results have been plotted to.
"""
# Use plt.subplots to create Figure and Axes objects
# if one is not provided.
if ax is None:
fig, ax = plt.subplots()
# Create plot
ax.plot(range(len(S)), S, label="S")
ax.plot(range(len(I)), I, label="I")
ax.plot(range(len(R)), R, label="R")
ax.set_xlabel("Time /days")
ax.set_ylabel("Population")
ax.legend()
# Optionally save the figure
if save_to is not None:
fig = ax.get_figure()
fig.savefig(save_to)
# Optionally show the figure
if show:
plt.show()
return ax
def main():
# Create an argument parser object. We can provide
# some info about our program here.
parser = ArgumentParser(
prog="SIR_model",
description="Solves SIR model and creates a plot",
)
# Add each argument to the parser. We can specify
# the types of each argument. Optional arguments
# should have single character names with a hypen,
# or longer names with a double dash.
parser.add_argument(
"-p", "--pop_size", type=int, default=10000000,
help="Total population size",
)
parser.add_argument(
"-b", "--beta", type=float, default=0.5,
help="Average no. of new infections per infected person per day",
)
parser.add_argument(
"-g", "--gamma", type=float, default=0.1,
help="Inverse of average number of days taken to recover",
)
parser.add_argument(
"-d", "--days", type=int, default=150,
help="Number of days to run the simulation",
)
parser.add_argument(
"-i", "--i0", type=int, default=10,
help="Number of infected people at the start of the simulation",
)
parser.add_argument(
"-o", "--output", default="SIR_model.png",
help="Output file to save plot to",
)
# Get each argument from the command line
args = parser.parse_args()
# Run our code
S, I, R = SIR_model(
pop_size=args.pop_size,
beta=args.beta,
gamma=args.gamma,
days=args.days,
I_0=args.i0,
)
plot_SIR_model(S, I, R, save_to=args.output)
if __name__ == "__main__":
main()
This will work if we run
python3 -m epi_models.plotting.plot_SIR
, but it won’t work
if we try to run it using python3 -m epi_models SIR
, as we
need to read from the command line in __main__.py
in order
to decide which model to run, but plot_SIR.py
will also try
to read from the command line to determine its own setup. Inevitably,
each scripting interface will expect a different set of arguments. The
solution comes in the form of subparsers
. We’ll show how
these work using the following simple example:
PYTHON
# file: subparsers.py
from argparse import ArgumentParser
parser = ArgumentParser(description="A test program")
# set up subparsers, make them required
subparsers = parser.add_subparsers(required=True)
# add two subcommands: cmd1 and cmd2
parser1 = subparsers.add_parser("cmd1")
parser2 = subparsers.add_parser("cmd2")
# add some args to each
parser1.add_argument("foo")
parser1.add_argument("bar")
parser2.add_argument("baz")
# parse args and print what we get
args = parser.parse_args()
print(args)
If we try running this with no arguments, it breaks! If we check how
it should work using --help
:
usage: subparsers.py [-h] {cmd1,cmd2} ...
A test program
positional arguments:
{cmd1,cmd2}
optional arguments:
-h, --help show this help message and exit
We therefore need to supply either cmd1
or
cmd2
as the first argument. We can then get further info on
each subcommand:
usage: subparsers.py cmd1 [-h] foo bar
positional arguments:
foo
bar
optional arguments:
-h, --help show this help message and exit
So what if we provide these arguments?
Namespace(bar='2', foo='1')
We see that foo
and bar
are defined within
args
, but not baz
. Similarly:
Namespace(baz='3')
We can therefore use subparsers to set up multiple independent
command line interfaces using the same root command. If we wished, we
could even create further subparsers from our subparsers, leading to a
nested command line interface! Something to note is that information
about which command was chosen is not contained within args;
we’ll show a workaround for this later using
set_defaults()
.
Let’s apply subparsers to our epi_models
package. First,
our main()
function should be split into a function that
assigns arguments to an ArgumentParser
, and a function that
runs the code using args
:
PYTHON
# file: plot_SIR.py
def _add_arguments(parser):
parser.add_argument(
"-p", "--pop_size", type=int, default=10000000,
help="Total population size",
)
parser.add_argument(
"-b", "--beta", type=float, default=0.5,
help="Average no. of new infections per infected person per day",
)
parser.add_argument(
"-g", "--gamma", type=float, default=0.1,
help="Inverse of average number of days taken to recover",
)
parser.add_argument(
"-d", "--days", type=int, default=150,
help="Number of days to run the simulation",
)
parser.add_argument(
"-i", "--i0", type=int, default=10,
help="Number of infected people at the start of the simulation",
)
parser.add_argument(
"-o", "--output", default="SIR_model.png",
help="Output file to save plot to",
)
def main(args):
# Run our code
S, I, R = SIR_model(
pop_size=args.pop_size,
beta=args.beta,
gamma=args.gamma,
days=args.days,
I_0=args.i0,
)
plot_SIR_model(S, I, R, save_to=args.output)
The if __name__ == "__main__"
section should be tasked
with creating an ArgumentParser
and coordinating
everything:
PYTHON
# file: plot_SIR.py
if __name__ == "__main__":
parser = ArgumentParser(
prog="SIR_model",
description="Solves SIR model and creates a plot",
)
_add_arguments(parser)
args = parser.parse_args()
main(args)
As the _add_arguments(parser)
function doesn’t care
whether it’s supplied with a parser or a subparser, this will work just
fine if we run this file directly using
python3 -m epi_models.plotting.plot_SIR
.
A similar refactor should be applied for every other model. There is
no need for each model to require the same command line arguments, but
they should each have a function equivalent to
_add_arguments(parser)
and main(args)
.
We can then switch between each model in __main__.py
using subparsers. The file below shows how to achieve a rich command
line interface for epi_models
:
PYTHON
# file: __main__.py
from argparse import ArgumentParser
# Import each _add_arguments and main, giving each an alias
from .plotting.plot_SIR import main as SIR_main
from .plotting.plot_SEIR import main as SEIR_main
from .plotting.plot_SIS import main as SIS_main
from .plotting.plot_SIR import _add_arguments as add_SIR_arguments
from .plotting.plot_SIR import _add_arguments as add_SEIR_arguments
from .plotting.plot_SIR import _add_arguments as add_SIS_arguments
# Create top-level ArgumentParser
parser = ArgumentParser(
prog="epi_models",
description="Tool for solving epidemiology models",
)
# Set up subparsers, adding a new one for each model
subparsers = parser.add_subparsers(required=True)
SIR_parser = subparsers.add_parser("SIR")
SEIR_parser = subparsers.add_parser("SEIR")
SIS_parser = subparsers.add_parser("SIS")
# Setup each subparser using each model's _add_arguments
add_SIR_arguments(SIR_parser)
add_SEIR_arguments(SEIR_parser)
add_SIS_arguments(SIS_parser)
# Ensure each parser knows which function to call.
# set_defaults can be used to set a new arg which
# isn't set on the command line.
SIR_parser.set_defaults(main=SIR_main)
SEIR_parser.set_defaults(main=SEIR_main)
SIS_parser.set_defaults(main=SIS_main)
# Extract command line arguments and run
args = parser.parse_args()
args.main(args)
Here, we used set_defaults()
to assign each model’s
main()
function to the variable main
within
args
. This will be set depending on which subcommand the
user provides. Each model’s _add_arguments
can be used as a
‘black box’, and each can be developed independently of the others.
Following this, we can use epi_models
to run several
different models, each with their own command line interface!
BASH
$ python3 -m epi_models SIR --pop_size=300 --output="SIR.png"
$ python3 -m epi_models SEIR --alpha=1
Key Points
- Packages can be used to better organise our code as it becomes more complex
- Packages are defined by a directory structure and the presence of
__init__.py
- By controlling what is or isn’t imported in
__init__.py
, we can define a public API for our project. - A package can be run as a script using the
-m
flag, provided it contains a file__main__.py
. - Through clever use of
argparse
, we can provide a rich scripting interface to a whole package.
Content from Building and Installing Packages using setuptools
Last updated on 2024-04-16 | Edit this page
Estimated time: 20 minutes
Overview
Questions
- How can we manage our Python environment?
- How can we install our own packages?
Objectives
- Use
venv
to manage Python environments - Understand what happens when we install a package
- Use
setuptools
to install packages to our local environment
Introduction
In the first lesson, we showed how to use the PYTHONPATH
environment variable to enable us to import our modules and packages
from anywhere on our system. There are a few disadvantages to this
method:
- If we have two different versions of a package on our system at
once, it can be tedious to manually update
PYTHONPATH
whenever we want to switch between them. - If we have multiple Python environments on our system (using tools
such as
venv
orconda
), settingPYTHONPATH
will affect all of them. This can lead to unexpected dependency conflicts that can be very hard to debug. - If we share our software with others and require them to update
their own
PYTHONPATH
, they will need to install any requirements for our package separately, which can be error prone.
It would be preferable if we could install our package using
pip
, the same way that we would normally install external
Python packages. However, if we enter the top level directory of our
project and try the following:
We get the following error:
OUTPUT
ERROR: Directory '.' is not installable. Neither 'setup.py' nor 'pyproject.toml' found.
In order to make our project installable, we need to add the either
the file pyproject.toml
or setup.py
to our
project. For modern Python projects, it is recommended to write only
pyproject.toml
. This was introduced by PEP 517, PEP 518 and PEP 621 as a standard way
to define a Python project, and all tools that build, install, and
publish Python packages are expected to use it.
What issetup.py
?
setup.py
serves a similar role to
pyproject.toml
, but it is no longer recommended for use.
The lesson on the history of
build tools explains how it works and why the community has moved
away from it.
By making our project pip
-installable, we’ll also make
it very easy to publish our packages on public repositories – this will
be covered in our lesson on package
publishing. After publishing our work, our users will be able to
download and install our package using pip
from any machine
of their chocie!
To begin, we’ll introduce the concept of a ‘Python environment’, and how these can help us manage our workflows.
Managing Python Environments
When working with Python, it can sometimes be beneficial to install packages to an isolated environment instead of installing them globally. Usually, this is done to manage competing dependencies:
- Project B might depend upon Project A, but may have been written to use version 1.0.
- Project C might also depend upon Project A, but may instead only work with version 2.0.
- If we install Project A globally and choose version 2.0, then Project B will not work. Similarly, if we choose version 1.0, Project C will not work.
A good way to handle these sorts of conflicts is to instead use
virtual environments for each project. A number of tools have
been developed to manage virtual environments, such as
venv
, which is a standard built-in Python tool, and
conda
, which is a powerful third-party tool. We’ll focus on
venv
here, but both tools work similarly.
If we’re using Linux, we can find which Python environment we’re using by calling:
If we’re using the default system environment, the result is something like the following:
OUTPUT
/usr/bin/python3
To create a new virtual environment using venv
, we can
call:
This will create a new directory at the location
/path/to/my/env
. Note that this can be a relative path, so
just calling python3 -m venv myenv
will create the virtual
environment in the directory ./myenv
. We can then
‘activate’ the virtual environment using:
Checking which Python we’re running should now give a different result:
OUTPUT
/path/to/my/env/bin/python3
If we now install a new package, it will be installed within our new virtual environment instead of being installed to the system libraries. For example:
We should now find NumPy installed at the following location (note that the Python version may not match yours):
site-packages
is a standard location to store installed
Python packages. We can see this by analysing Python’s import path:
['', '/usr/lib/python38.zip', '/usr/lib/python3.8', '/usr/lib/python3.8/lib-dynload', '/path/to/my/env/lib/python3.8/site-packages']
If we no longer wish to use this virtual environment, we can return to the system environment by calling:
Virtual environments are very useful when we’re testing our code, as they allow us to create a fresh Python environment without any of the installed packages we normally use in our work. This will be important later when we add dependencies to our package, as this allows us to test whether our users will be able to install and run our code properly using a fresh environment.
An Overview of TOML files
pyproject.toml
is a TOML file, which stands for ‘Tom’s
Obvious Minimal Langauge’ (named for its developer, Thomas
Preston-Werner, who cofounded GitHub). There are many configuration file
formats in common usage, such as YAML, JSON, and INI, but the Python
community chose TOML as it provides some benefits over the
competition:
- Designed to be human writable and human readable.
- Can map unambiguously to a hash table (a
dict
in Python). - It has a formal specification, so has an unambiguous set of rules.
A TOML file contains a series of key = value
pairs,
which may be grouped into sections using a header enclosed in square
brackets, such as [section name]
. The values are typed,
unlike some other formats where all values are strings. The available
types are strings, integers, floats, booleans, and dates. It is possible
to store lists of values in arrays, or store a series of key-value pairs
in tables. For example:
TOML
# file: mytoml.toml
int_val = 5
float_val = 0.5
string_val = "hello world"
bool_val = true
date_val = 2023-01-01T08:00:00
array = [1, 2, 3]
inline_table = {key = "value"}
# Section headings allow us to define tables over
# multiple lines
[header_table]
name = "John"
dob = 2002-03-05
# We can define subtables using dot notation
[header_table.subtable]
foo = "bar"
We can read this using the toml
library in Python:
PYTHON
>>> import toml
>>> with open("mytoml.toml", "r") as f:
... data = toml.load(f)
>>> print(data)
The result is a dictionary object, with TOML types converted to their corresponding Python types:
{
'int_val': 5,
'float_val': 0.5,
'string_val': 'hello world',
'bool_val': True,
'date_val': datetime.datetime(2023, 1, 1, 8, 0),
'array': [1, 2, 3],
'inline_table': {'key': 'value'},
'header_table': {
'name': 'John',
'dob': datetime.date(2002, 3, 5),
'subtable': {
'foo': 'bar'
}
}
}
Installing our package with pyproject.toml
First, we will show how to write a relatively minimal
pyproject.toml
file so that we can install our projects
using pip
. We will then cover some additional tricks that
can be achieved with this file:
- Use alternative directory structures
- Include any data files needed by our code
- Generate an executable so that our scripts can be run directly from the command line
- Configure our development tools.
To make our package pip
-installable, we should add the
file pyproject.toml
to the top-level
epi_models
directory:
📁 epi_models
|
|____📜 pyproject.toml
|____📦
epi_models
|
|____📜 __init__.py
|____📜
__main__.py
|
|____📁 models
| |
| |____📜 __init__.py
| |____📜 SIR.py
| |____📜 SEIR.py
| |____📜 SIS.py
| |____📜 utils.py
|
|____📁 plotting
|
|____📜 __init__.py
|____📜
plot_SIR.py
|____📜 plot_SEIR.py
|____📜
plot_SIS.py
The first section in our pyproject.toml
file should
specify which build system we wish to use, and additionally specify any
version requirements for packages used to build our code. This is
necessary to avoid a circular dependecy problem that occurred with
earlier Python build systems, in which the user had to run an install
program to determine the project’s dependencies, but needed to already
have the correct build tool installed to run the install program – see
the lesson on historical build
tools for more detail. We will choose to use
setuptools
, which requires the following:
TOML
# file: pyproject.toml
[build-system]
requires = [
"setuptools >= 65",
"wheel >= 0.38,
]
build-backend = "setuptools.build_meta"
-
requires
is set to a list of strings, each of which names a dependency of the build system and (optionally) its minimum version. This uses the same version syntax aspip
. -
build-backend
is set to a sub-module ofsetuptools
which implements the PEP 517 build interface.
With our build system determined, we can add some metadata that defines our project. At a minimum, we should specify the name of the package, its version, and our dependencies:
TOML
# file: pyproject.toml
[build-system]
requires = [
"setuptools >= 65",
"wheel >= 0.38,
]
build-backend = "setuptools.build_meta"
[project]
name = "epi_models"
version = "0.1.0"
dependencies = [
"matplotlib",
]
That’s all we need! We’ll discuss versioning in our lesson on publishing. With this done, we can install our package using:
This will automatically download and install our dependencies, and our package will be importable regardless of which directory we’re in.
The installed package can be found in the directory
/path/to/my/env/lib/python3.8/site-packages/epi_models
along with a new directory, epi_models-0.1.0.dist-info
,
which simply contains metadata describing our project. If we look inside
our installed package, we’ll see that our files have been copied, and
there is also a __pycache__
directory:
__init__.py __main__.py models plotting __pycache__
The __pycache__
directory contains Python bytecode,
which is a lower-level version of Python that is understood by the
Python Virtual Machine (PVM). All of our Python code is converted to
bytecode when it is run or imported, and by pre-compiling our package it
can be imported much faster. If we look into the directories
models
and plotting
, we’ll see those have been
compiled to bytecode too.
If we wish to uninstall, we may call:
We can also create an ‘editable install’, in which any changes we make to our code are instantly recognised by any codes importing it – this mode can be very useful when developing our code, especially when working on documentation or tests.
Callout
The ability to create editable installs from a
pyproject.toml
-only build was standardised in PEP 660, and only recently
implemented in pip
. You may need to upgrade to use this
feature:
There are many other options we can add to our
pyproject.toml
to better describe our project. PEP 621 defines a minimum
list of possible metadata that all build tools should support, so we’ll
stick to that list. Each build tool will also define synonyms for some
metadata entries, and additional tool-specific metadata. Some of the
recommended core metadata keys are described below:
TOML
# file: pyproject.toml
[project]
# name: String, REQUIRED
name = "my_project"
# version: String, REQUIRED
# Should follow PEP 440 rules
# Can be provided dynamically, see the lesson on publishing
version = "1.2.3"
# description: String
# A simple summary of the project
description = "My wonderful Python package"
# readme: String
# Full description of the project.
# Should be the path to your README file, relative to pyproject.toml
readme = "README.md"
# requires-python: String
# The Python version required by the project
requires-python = ">=3.8"
# license: Table
# The license of your project.
# Can be provided as a file or a text description.
# Discussed in the lesson on publishing
license = {file = "LICENSE.md"}
# or...
license = {text = "BDS 3-Clause License"}
# authors: Array of Tables
# Can also be called 'maintainers'.
# Each entry can have a name and/or an email
authors = [
{name = "My Name", email = "my.email@email.net"},
{name = "My Friend", email = "their.email@email.net"},
]
# urls: Table
# Should describe where to find useful info for your project
urls = {source = "github.com/MyProfile/my_project", documentation = "my_project.readthedocs.io/en/latest"}
# dependencies: Array of Strings
# A list of requirements for our package
dependencies = [
"numpy >= 1.20",
"pyyaml",
]
Note that some of the longer tables in our TOML file can be written using non-inline tables if it improved readability:
Alternative Directory Structures
setuptools
provides some additional tools to help us
install our package if they use a different layout to the ‘flat’ layout
we covered so far. A popular alternative layout is the
src
-layout:
📁 epi_models
|
|____📜 pyproject.toml
|____📁
src
|
|____📦 epi_models
|
|____📜 __init__.py
|____📜 __main__.py
|____📁 models
|____📁 plotting
The main benefit of this choice is that setuptools
won’t
accidentally bundle any utility modules stored in the top-level
directory with our package. It can also be neater when one project
contains multiple packages. Note that directories and files with special
names are excluded by default regardless of which layout we choose, such
as test/
, docs/
, and
setup.py
.
We can also disable automatic package discovery and explicitly list the packages we wish to install:
Note that this is not part of the PEP 621 standard, and
therefore instead of being listed under the [project]
header, it is a method specific to setuptools
. Finally, we
may set up custom package discovery:
TOML
# file: pyproject.toml
[tool.setuptools.packages.find]
where = ["my_directory"]
include = ["my_package", "my_other_package"]
exclude = ["my_package.tests*"]
However, for ease of use, it is recommended to stick to either the
flat layout or the src
layout.
Package Data
Sometimes our code requires some non-.py
files in order
to function properly, but these would not be picked up by automatic
package discovery. For example, the project may store default input data
in .json
files. These could be included with your package
by adding the following to pyproject.toml
:
Note that this would grab only .json
files in the
top-level directory of our project. To include data files from all
packages and sub-packages, we should instead write:
Installing Scripts
If our package contains any scripts and/or a __main__.py
file, we can run those from anywhere on our system after
installation:
With a little extra work, we can also install a simplified interface
that doesn’t require python3 -m
in front. This is how tools
like pip
can be invoked using two possible methods:
This can be achieved by adding a table scripts
under the
[project]
header:
TOML
# file: pyproject.toml
[project]
scripts = {epi_models = "epi_models.__main__:main"}
# Alternative form:
[project.scripts]
epi_models = "epi_models.__main__:main"
This syntax means that we should create a console script
epi_models
, and that running it should call the function
main()
from the file epi_models/__main__.py
.
This will require a slight modification to our __main__.py
file. All that’s necessary is to move everything from the script into a
function main()
that takes no arguments, and then to call
main()
at the bottom of the script:
This will allow us to run our package as a script directly from the command line
Note that we’ll still be able to run our code using the longer form:
If we have multiple scripts in our package, these can all be given invidual console scripts. However, these will also need to have a function name as an entry point:
TOML
# file: pyproject.toml
[project.scripts]
epi_models = "epi_models.__main__:main"
epi_models_sir = "epi_models.plotting.plot_SIR:main"
So how do these scripts work? When we activate a virtual environment,
a new entry is added to our PATH
environment variable
linking to /path/to/my/env/bin/
:
After installing our console scripts, we can find a new file in this
directory with the name we assigned to it. For example,
/path/to/my/env/bin/epi_models
:
PYTHON
#!/path/to/my/env/bin/python3
# -*- coding: utf-8 -*-
import re
import sys
from epi_models.__main__ import main
if __name__ == '__main__':
sys.argv[0] = re.sub(r'(-script\.pyw|\.exe)?$', '', sys.argv[0])
sys.exit(main())
Installing our project has automatically created a new Python file
that can be run as a command line script due to the hash-bang
(#!
) on the top line, and all it does it import our main
function and run it. As it’s contained with the bin/
directory of our Python environment, it’s available for use as long
we’re using that environment, but as soon as we call
deactivate
, it is removed from our PATH
.
Setting Dependency Versions
Earlier, when setting dependencies
in our
pyproject.toml
, we chose to specify a minimum requirement
for numpy
, but not for pyyaml
:
This indicates that pip
should install any version of
numpy
greater than 1.20, but that any version of
pyyaml
will do. If our installed numpy
version
is less than 1.20, or if it isn’t installed at all, pip
will upgrade to the latest version that’s compatible with the rest of
our installed packages and our Python version. We’ll cover software
versioning in more detail in the lesson on
publishing, but now we’ll simply cover some ways to specify which
software versions we need:
TOML
"numpy >= 1.20" # Must be at least 1.20
"numpy > 1.20" # Must be greater than 1.20
"numpy == 1.20" # Must be exactly 1.20
"numpy <= 1.20" # Must be 1.20 at most
"numpy < 1.20" # Must be less than 1.20
"numpy == 1.*" # Must be any version 1
If we separate our clauses with commas, we can combine these requirements:
A useful shorthand is the ‘compatible release’ clause:
This is equivalent to:
That is, we require anything which is version 1, provided it’s greater than 1.20. This would include version 1.25, but exlude version 2.0. We’ll come back to this later when we discuss publishing.
Optional Dependencies
Sometimes we might have dependencies that only make sense for certain
kind of user. For example, a developer of our library might need any
libraries we use to run unit tests or build documentation, but an end
user would not. These can be added as
optional-dependencies
:
TOML
# file: pyproject.toml
[project.optional-dependencies]
test = [
"pytest >= 5.0.0",
]
doc = [
"sphinx",
]
These dependencies can be installed by adding the name of each
optional dependency group in square brackets after telling
pip
what we want to install:
Additional Tools
Some additional tools unrelated to package building also make use of
pyproject.toml
. Their settings are typically set using
[tool.*]
headings. For example, the tool black
which is
used to auto-format Python code can be configured here:
Not all popular Python tools have adopted pyproject.toml
yet. Notably, the linter flake8
cannot be configured this way, and users will instead need to use a
.flake8
file (or one of a few alternative config
files).
Content from Extra: A History of Python Build Tools
Last updated on 2024-04-16 | Edit this page
Estimated time: 30 minutes
Overview
Questions
- Why have the ‘best practices’ for building and installing Python packages changed so much of the years?
- What problems has each new iteration solved?
Objectives
- Understand the history of Python packaging
- Understand what each method does when we install a package
This lesson can be skipped entirely if you’re aiming for a purely practical lesson.
Introduction
In the previous lesson, we showed how to install Python packages
using the file pyproject.toml
. However, if you ever find
yourself looking for online help on a Python packaging topic, you’ll
likely find a large number of alternative methods being recommended.
Some say you should use a file setup.py
, while others
recommend a combination of setup.cfg
and
setup.py
– some even say to use both these and
pyproject.toml
!
The reason for this conflicting information is that the Python packaging ‘best practices’, as decided by the Python community, have changed a number of times over the years. This lesson is optional, but it will explain why some older tutorials recommend some methods over the ones we’ve covered here. The intention here is not to present alternative methods so that you can use them in new projects, but rather to provide some context to the often confusing information out there, and to help if you ever find yourself working on a project that hasn’t yet updated to the latest standards.
In the beginning, there was distutils
First introduced with Python 2.2, distutils
was a module
of Python’s standard library that allowed users to install and
distribute their own packages. However, it was deprecated in PEP 632, having been
superceded by setuptools
. The primary issue with
distutils
is that it is strongly coupled to the user’s
Python version, and the developers found they could not implement some
features or fixes without breaking inter-version compatibility.
Nowadays, it is recommended to use a combination of pip
and
setuptools
instead, but it will be useful to briefly cover
basic distutils
usage so that we can understand some of the
design choices that would follow.
To use distutils
to install a package, the user would
create a file setup.py
and (optionally)
requirements.txt
in the same directory as the top-level
package:
📁 epi_models
|
|____📜 setup.py
|____📜
requirements.txt
|____📦 epi_models
|
|____📜
__init__.py
|____📜 __main__.py
|____📁 models
|____📁 plotting
The file setup.py
should contain a script that contains
metadata about the package and its author(s). A very simple one might
look like this:
PYTHON
# file: setup.py
from distutils.core import setup
setup(
name="epi_models",
version="1.0",
description="Epidemiology modelling tools in Python",
author="Jordan Smith",
author_email="jsmith@email.net",
url="https://github.com/jsmith1234/epi_models",
packages=["epi_models", "epi_models.models", "epi_models.plotting"],
)
Note that each subpackage is explicitly listed, unlike modern systems that make assumptions about your directory structure and perform automatic package discovery.
To install, setup.py
could be run as a script with the
argument install
:
This creates a ‘source distribution’ in a new directory
./build
, and adds it to the current environment. We can see
how the package is added to our environmentby reading the output written
to the terminal:
creating /path/to/my/env/lib/python3.8/site-packages/epi_models
copying build/lib/epi_models/__init__.py -> /path/to/my/env/lib/python3.8/site-packages/epi_models
creating /path/to/my/env/lib/python3.8/site-packages/epi_models/models
copying build/lib/epi_models/models/utils.py -> /path/to/my/env/lib/python3.8/site-packages/epi_models/models
copying build/lib/epi_models/models/__init__.py -> /path/to/my/env/lib/python3.8/site-packages/epi_models/models
copying build/lib/epi_models/models/SEIR.py -> /path/to/my/env/lib/python3.8/site-packages/epi_models/models
copying build/lib/epi_models/models/SIR.py -> /path/to/my/env/lib/python3.8/site-packages/epi_models/models
copying build/lib/epi_models/models/SIS.py -> /path/to/my/env/lib/python3.8/site-packages/epi_models/models
creating /path/to/my/env/lib/python3.8/site-packages/epi_models/plotting
copying build/lib/epi_models/plotting/__init__.py -> /path/to/my/env/lib/python3.8/site-packages/epi_models/plotting
copying build/lib/epi_models/plotting/plot_SIS.py -> /path/to/my/env/lib/python3.8/site-packages/epi_models/plotting
copying build/lib/epi_models/plotting/plot_SEIR.py -> /path/to/my/env/lib/python3.8/site-packages/epi_models/plotting
copying build/lib/epi_models/plotting/plot_SIR.py -> /path/to/my/env/lib/python3.8/site-packages/epi_models/plotting
copying build/lib/epi_models/__main__.py -> /path/to/my/env/lib/python3.8/site-packages/epi_models
byte-compiling /path/to/my/env/lib/python3.8/site-packages/epi_models/__init__.py to __init__.cpython-38.pyc
byte-compiling /path/to/my/env/lib/python3.8/site-packages/epi_models/models/utils.py to utils.cpython-38.pyc
byte-compiling /path/to/my/env/lib/python3.8/site-packages/epi_models/models/__init__.py to __init__.cpython-38.pyc
byte-compiling /path/to/my/env/lib/python3.8/site-packages/epi_models/models/SEIR.py to SEIR.cpython-38.pyc
byte-compiling /path/to/my/env/lib/python3.8/site-packages/epi_models/models/SIR.py to SIR.cpython-38.pyc
byte-compiling /path/to/my/env/lib/python3.8/site-packages/epi_models/models/SIS.py to SIS.cpython-38.pyc
byte-compiling /path/to/my/env/lib/python3.8/site-packages/epi_models/plotting/__init__.py to __init__.cpython-38.pyc
byte-compiling /path/to/my/env/lib/python3.8/site-packages/epi_models/plotting/plot_SIS.py to plot_SIS.cpython-38.pyc
byte-compiling /path/to/my/env/lib/python3.8/site-packages/epi_models/plotting/plot_SEIR.py to plot_SEIR.cpython-38.pyc
byte-compiling /path/to/my/env/lib/python3.8/site-packages/epi_models/plotting/plot_SIR.py to plot_SIR.cpython-38.pyc
byte-compiling /path/to/my/env/lib/python3.8/site-packages/epi_models/__main__.py to __main__.cpython-38.pyc
There are three stages:
- First, create
./build
and make a source distribution there. - Create a new directory within
/path/to/my/env/lib/python3.8/site-packages/
, and copy the contents of./build/lib
. - Compile each file to Python bytecode so it can be run more quickly later.
The end result is therefore very similar to what happens when we
install using pyproject.toml
. However, a significant
downside of using distutils
and setup.py
is
that there is no way to safely uninstall a project once it has been
installed, and instead the user must manually remove the package from
their site-packages
folder. The creation of a
./build
directory also tends to clutter up our
workspace.
distutils
contains many other utilities, such as adding
language extensions written in C. However, it does not allow users to
specify dependencies, and these are instead expected to be listed in the
file requirements.txt
:
matplotlib>=3.6
The user can install the requirements by calling:
It is not recommended to use distutils
for new projects,
and it will be removed from standard Python distributions in version
3.12. Listing dependencies in requirements.txt
is also no
longer required.
setuptools
and egg
files
setuptools
is not part of the core Python library, but
it has become the de facto standard build tool. Originally, it
added extra functionality on top of distutils
using a
complicated collection of subclasses and monkeypatching, and it offered
better support across multiple Python versions. It has since superceded
distutils
entirely.
Using setuptools
, it is possible to use
setup.py
to define a package in much the same way as
distutils
:
PYTHON
# file: setup.py
from setuptools import setup
setup(
name="epi_models",
version="1.0",
description="Epidemiology modelling tools in Python",
author="Jordan Smith",
author_email="jsmith@email.net",
url="https://github.com/jsmith1234/epi_models",
packages=["epi_models", "epi_models.models", "epi_models.plotting"],
install_requires=["matplotlib>=3.6"],
)
Note the addition of an extra field, install_requires
.
This allows us to specify the dependencies without the extra file
requirements.txt
, and these libraries will be installed
alongside our package when we install it.
We can run this file just as we did with distutils
:
Again, this will create a new directory ./build
, but it
will also create a directory ./dist
. Much as before,
./build
contains a ‘source distribution’ of our code, while
./dist
contains a file with a name such as
epi_models-1.0-py3.8.egg
and a directory
epi_models.egg-info
containing metadata files describing
our project. The ‘egg’ file is a distributable package format used by
setuptools
, and is essentially just a .zip
file containing our package with a name specifying both the package
version and the version of Python it runs on. We can show this on Linux
systems using the unzip
command line utility:
OUTPUT
EGG-INFO epi_models
Inside the .egg
file, we find a copy of the
.egg-info
directory created earlier, and a copy of our
package. If we look inside this version of our package, we can also see
that each directory contains a __pycache__
, with Python
bytecode .pyc
files inside, meaning each file has been
pre-compiled. This is done to optimise the egg file, as they are
intended to be directly importable. However, it also means the egg file
is only compatible with some Python versions, so projects written to be
compatible with both Python 2 and Python 3 must have separate
.egg
files for each Python version. Egg files have been
superseded by ‘wheel’ files, which we’ll discuss in the next lesson.
So what happens when we install with setuptools
as
opposed to distutils
? A clue can be found in some of the
text outputted when we installed:
Adding epi-models 1.0 to easy-install.pth file
If we look in our site-packages
directory, we’ll see a
file easy-install.pth
. This is a path configuration file,
denoted by the extension .pth
, and if any are found within
site-packages
(or a number of other Python configuration
directories), any additional items listed in it will be added to
sys.path
whenever Python is loaded up. If we look into this
file, we find:
./epi_models-1.0-py3.8.egg
Therefore, rather than copying our source distribution into
site-packages
, setuptools
has instead copied
the egg file and added its location to sys.path
. This last
step is necessary, as while Python is capable of running/importing
zipped directories, it doesn’t automatically search zip files on the
import path for any modules contained within.
Although there is still no way to uninstall using
setup.py
, it is possible to remove a package installed this
way using pip:
Using pip
instead of running setup.py
The direct usage of setup.py
is now discouraged. After
installing a package using python3 setup.py install
, there
is no equivalent command to uninstall. It also tends to clutter the
user’s workspace by creating local ./build
and
./dist
directories. Both of these problems can be solved
using pip
, which also provides a number of further
benefits:
This will install the library to the current Python environment. It can then be uninstalled using:
When installing with pip
instead of running
setup.py
, no ./build
or ./dist
directories are created, nor are any .egg
files. Two lines
which are printed to screen during the install process explain what is
happening instead:
Created wheel for epi-models: filename=epi_models-1.0-py3-none-any.whl size=4968 sha256=f876a8ce10a3b6a6d0261f4d739b1d78904153b4feaf2b37f83de60e4b9c2d36
Stored in directory: /tmp/pip-ephem-wheel-cache-_og4ywxd/wheels/d9/4d/ac/bbc1437fd83635787dd0fb8c3df8da61fc7b57f6eaa2b0d17b
Instead of creating an egg file, a ‘wheel’ is created instead. We’ll cover wheel files in more detail in the next lesson, but to summarise, a wheel is very similar to an egg file except for two major differences:
- The code within is not compiled to Python bytecode.
- The name of the file contains more information regarding its compatibility.
The wheel is created in a temporary directory (/tmp
on
most Linux systems) so that it doesn’t clutter up our workspace, and
when installing from a wheel, the file is unzipped within
site-packages
and the code within is compiled to bytecode.
This is in contrast to egg files, which remain zipped at all times and
come with their code pre-compiled.
To aid code development, we can also create editable installs, in which the user’s changes to the code are automatically picked up and there is no need to reinstall:
This works simply by adding the path of our working directory into
easy-install.pth
within the site-packages
directory. It will also install any dependencies of the project as
normal.
The usage of setup.py
gained further criticism, even
when used alonside pip
. As library writers were able to add
arbitrary code to this file, setup scripts often became very long and
difficult for users to understand. They could also contain potentially
dangerous (or even malicious) code that may not be apparent at first
glance. distutils
had also supported an alternative method
of specifying package metadata using an additional file
setup.cfg
, and in time this became the preferred
method.
setup.cfg
as a ‘Declarative Config’
Instead of using setup.py
to define our package
metadata, it is preferable to instead use the file
setup.cfg
. Instead of being a runnable Python module, this
file is simply a config file that can be read using Python’s built-in
configparser
tool. Its format is very similar to INI files
commonly used on Windows systems. It should be included at the very top
of a project, just like setup.py
:
📁 epi_models
|
|____📜 setup.cfg
|____📜
setup.py
|____📦 epi_models
|
|____📜
__init__.py
|____📜 __main__.py
|____📁 models
|____📁 plotting
setup.py
is still present, but it can be reduced to a
very simple form:
The setup.py
files we used earlier can be easily adapted
to a corresponding setup.cfg
:
INI
; file: setup.cfg
[metadata]
name = epi_models
version = 1.0
description = Epidemiology modelling tools in Python
author = Jordan Smith
author_email = jsmith@email.net
url = "https://github.com/jsmith1234/epi_models"
[options]
packages = find:
install_requires =
matplotlib >= 3.6
Note that the values on the right hand side of each equals sign are
all intepretted as strings by default, and double quotes are only needed
to escape certain special characters. Values can stretch over a line
break by indenting the next line down. Comment lines start with either
;
or #
, and inline comments are not
allowed.
The benefit of using this file over setup.py
is that the
number of possible operations is restricted. This prevents users from
writing overly complex setup.py
files that include
arbitrary and potentially dangerous computations. This is also
beneficial from an automation standpoint, as it is easy for tools
besides setuptools
to read setup.cfg
and
deduce useful information about a package.
Note that in converting our setup.py
to a
setup.cfg
, we are no longer listing each package and
subpackage, and instead we are using the line:
This instructs setuptools
to detect packages itself. We
could instead specify this manually using:
A common alternative project layout is to place the top-level package
in a directory src
:
📁 epi_models
|
|____📜 setup.py
|____📜
setup.cfg
|____📁 src
|
|____📦 epi_models
|
|____📜 __init__.py
|____📜
__main__.py
|____📁 models
|____📁
plotting
Our setup.cfg
file should be rewritten as follows:
We can also continue using packages = find:
by adding an
extra heading to our config file:
There are many additional ways to organise a project, and it is
possible to set package names to something other than the corresponding
directory name, but it is recommended to keep the directory structure of
your projects as close as possible to that of the Python package. See
the documentation
for setuptools
keywords for more information.
pyproject.toml
and Circular Build Requirements
We saw in the previous sections that setuptools
allows
library writers to specify the requirements of their packages and allow
users to automatically download and install dependencies. However, there
is always one requirement missing from this list:
setuptools
itself! It would be useful if we could add
setuptools
to our install_requires
, but the
user already needs to have setuptools
installed in order to
parse this field! In the era of distutils
, this was not a
problem for Python developers, as distutils
was shipped as
part of Python’s standard library.
The resolution to this problem came in PEP 517 and PEP 518, which specifies
how to set build system requires within a new config file,
pyproject.toml
. setup.cfg
is understood by
setuptools
(and possibly distutils
), but
pyproject.toml
is intended to be understood by any
Python build tool, current or future. This includes pip
and
alternatives such as flit
or poetry
.
To specify our build system following PEP 517/518,
pyproject.toml
should be added to our project at the same
level as setup.py
and setup.cfg
:
📁 epi_models
|
|____📜 pyproject.toml
|____📜
setup.py
|____📜 setup.cfg
|____📦 epi_models
|
|____📜 __init__.py
|____📜 __main__.py
|____📁
models
|____📁 plotting
At a minimum, it should contain the following:
Following this, we may install our package using pip
without first having setuptools
installed. If we have an
older version of setuptools
installed, pip
will now fetch the latest version in an isolated virtual environment
prior to building our project.
If we try installing however, we may find the following warnings:
WARNING: Missing build requirements in pyproject.toml for file:///my/file/path.
WARNING: The project does not specify a build backend, and pip cannot fall back to setuptools without 'wheel'.
This means that pip
is falling back on default
behaviour, as we have not fully specified our build system. By default,
pip
will use setuptools
(more specifically,
the build backend setuptools.build_meta:__legacy__
), and it
requires the additional package wheel
(which we’ll discuss
further in the next lesson). To explicitly set our build system, we must
also provide a build-backend
:
Moving to pyproject.toml
-only builds
Following PEP 517/518, it was realised that
pyproject.toml
and setup.cfg
were both solving
similar problems, although pyproject.toml
was to be a
standardised config file, while setup.cfg
was
setuptools
specific (although many other tools in the
Python ecosystem had also adopted the usage of setup.cfg
).
Altenative build tools such as poetry
and flit
opted to use pyproject.toml
alone to define Python
packages, and this was eventually codified as a requirement for all
build tools in PEP
621.
The aim of PEP 621 is
to standardise a set of ‘core’ metadata that any build tool should
recognise, such as name
, version
,
description
, authors
, etc. Tools may choose to
use their own synonyms for this metadata, and they may provide many more
options than the core set, but to be standards compliant they must
recognise the metadata of PEP 621 at a minimum.
The end of setup.py
With PEP 621 removing
the need for setup.cfg
, one might expect that the older
setup.py
could be removed too. However,
setuptools
still required this file for some features to
work, including editable installs. With PEP 660, the Python
community standardised a way to use wheel files to create editable
installs, and therefore the use of setup.py
is no longer
required. However, this feature was only recently implemented in
pip
, so users may need to upgrade if they wish to use this
feature:
Key Points
- The ‘best practices’ for Python packaging has changed a number of times, and there are now many competing tools for accomplishing the same task.
- Be wary of information on this topic in online tutorials – not all guides have been updated to use the most recent methods, and some advice may no longer be relevant.
Content from Publishing our Python Packages
Last updated on 2024-04-16 | Edit this page
Estimated time: 30 minutes
Overview
Questions
- What services can we use to publish our software?
- What steps must we take to accomplish this?
Objectives
- Learn how to version our software and create releases.
- Learn how to publish our software on PyPi, the Python Packaging Index.
Publishing our Python Packages
Following our lesson on building and installing
Python packages, we should now have a package that can be installed
using pip
. With just a little more work, we can now publish
our software on a few free online services: GitHub for source/version
control, and PyPI (the Python Packaging Index) for package hosting. The
latter is the default repository used by pip
, so by hosting
our software there, other users will be able to install our software to
any machine of their choosing, and will be able to add it as a
dependency to their own projects.
Before discussing how to use these services, we’ll briefly cover ‘semantic versioning’: a method for adding meaningful versions to our software containing compatibility information, and we’ll look at some of the preparatory steps we should take before publishing our code.
Versions and Releases
Users of our software will expect it to stay consistent over time. If we were to suddenly rename a function, or change what arguments it takes, this could break our user’s code, which in turn could break another developer’s code, etc.
A good solution to this problem is to use ‘semantic versioning’, in which the version number contains compatibility information in a widely agreed-upon format. Using semanic versioning, we will periodically make a new ‘release’ of our code, each time updating its version number, and the way we change the number informs our users of whether the changes to our code will break compatibility. In its most basic form, semantic versioning requires 3 numbers, separated by periods, such as 1.2.3. These numbers stand for MAJOR.MINOR.PATCH, and are typically updated right-to-left. The meaning of each number is:
- PATCH: We increment the patch number whenever we make an update that doesn’t add or remove functionality. It can include things such as backwards-compatible bug fixes, internal code restructuring, and performance improvements.
- MINOR: The minor version should be incremented whenever we add new backwards-compatible features to our code. This can include the addition of new functions and classes. Whenever we increment the minor version, the patch version is reset to zero.
- MAJOR: The major version should be incremented whenever we make a change that breaks backwards compatibility. This could include changing a function name or signature, or removing something from the public API. It can also include changing a function’s behaviour in a significant way. Whenever we increment the major version, both the minor version and patch version should be reset to zero.
Challenge
Our contains the following function which gives the nth value of the Fibonacci sequence:
A user points out that this function causes an infinite loop if provided with a negative number, so we change it to:
PYTHON
def fibonacci(n):
if n < 0:
raise ValueError("n must be greater than or equal to 0")
elif n in [0, 1]:
return n
else:
return fibonacci(n-1) + fibonacci(n-2)
If our software was version 2.3.4, and after this change we make a new release, what should the new version number be?
2.3.5. This change is backwards compatible, and doesn’t add any new features. Instead, it simply fixes a bug.
Challenge
Our algorithm isn’t very efficient, so we convert it to an iterative algorithm:
PYTHON
def fibonacci(n):
if n < 0:
raise ValueError("n must be greater than or equal to 0")
elif n in [0, 1]:
return n
else:
last_two = [0, 1]
while n > 1:
val = sum(last_two)
last_two[0] = last_two[1]
last_two[1] = val
n -= 1
return val
If the previous version was 2.4.7, what should the new version be?
2.4.8. This change is backwards compatible, and doesn’t add any new features. Instead, it just improves the performance of an existing function.
Challenge
We decide to add a new function to our code that gives the user the full list of Fibonnaci numbers up to n:
PYTHON
def fibonacci_list(n):
if n < 0:
raise ValueError("n must be greater than or equal to 0")
elif n == 0:
return [0]
else:
result = [0, 1]
while n > 1:
result.append(result[-1] + result[-2])
n -= 1
return result
If the previous version was 3.2.1, what should the new version be?
3.3.0. We’ve added new features to our software, but we haven’t changed the existing API. Therefore the minor version should be incremented, and the patch number should be reset to zero.
Challenge
Our users seem to prefer the fibonacci_list
function, so
we decide to streamline our software by removing the original function
and renaming the new one fibonacci
.
PYTHON
def fibonacci(n):
if n < 0:
raise ValueError("n must be greater than or equal to 0")
elif n == 0:
return [0]
else:
result = [0, 1]
while n > 1:
result.append(result[-1] + result[-2])
n -= 1
return result
If the previous version was 3.4.5, what should the new version be?
4.0.0. By removing a function and changing the behaviour of another, we have changed the public API in a manner which is not backwards-compatible, and thus this might break our user’s code. We therefore must increment the major number, and set both the minor and patch numbers to zero.
We can add a version to our code by adding a __version__
to our top-most __init__.py
file:
This should also be reflected in our pyproject.toml
:
To avoid having to keep these up-to-date manually, we’ll see later
how to automatically generate version info using git
tags.
Something to note about the major and minor version numbers is that they only need to be updated if we change the public API of our software. Therefore, if we change the behaviour of any functions or classes intended for internal use, and the public API is unchanged, we only need to update the patch number.
As discussed in our lesson on
packages, e can indicate that a function, class, or variable is only
intended for internal use by prepending their name with an underscore
(_myvar
, _myfunc
, _MyClass
),
excluding it from __all__
, and excluding it from the user
API in any published documentation (although it may be a good idea to
document private objects in a separate developer API).
When we update the version of our software and release it publically, we must not go back and change it, no matter how tempting the prospect may be! Any fixes to our software may be perfomed by further releases, usually via the patch number. If a vulnerability is found in an old version of our software, it is permissible to return to it and release a new patch. For example, the last version of Python 2 was version 2.7.18, released in April 2020 – over a decade after the release of Python 3.0. If we choose to stop supporting an old version of our software, and therefore leave any vulnerabilities intact, this should be clearly stated to our users, and they should be strongly advised to upgrade.
With this understanding of semantic versioning, we can now better
understand the behaviour of the ‘compatible release’ comparator
~=
that we can use when setting dependencies in
pyproject.toml
:
This means that we require a version of NumPy which has at least all
of the features of version 1.22.1, but maintains backwards compatibility
with it. pip
will aim to get the highest possible version
that matches, so if a patch is released, it will get version 1.22.2. If
a new minor version is released, it will install 1.23.0. This continues
until the release of 2.0.0, which pip
will not install.
This is equivalent to:
Unstable Versions
Software with a major version of 0 is usually understood to be ‘unstable’. This means that anything can change between minor versions, and that no promises can be made for backwards compatibility. This is common for software in early stages of development, as the public API may undergo many iterations before being finalised.
For your own software, it may be a good idea to keep the major number at zero while you’re hashing out the public API and building a userbase. Once you’ve settled on a stable API, or you’ve developed a sizable userbase that won’t appreciate having to regularly update their code to match your changing standards, you should consider setting your version to 1.0.0.
If one of the dependencies in your project is still in an unstable
state, it may be a good idea to fix the minor version number instead of
using the compatible release comparator ~=
:
This is because the ~= 0.6
will get the latest release
which is at least 0.6.0
but less than1.0.0
,
but in this case version 0.7
might break backwards
compatibility with 0.6
. Note that some unstable projects
may also break backwards compatibiliy in their patch releases. In these
cases, it may be better to fix even the patch number:
Deprecation
It is considered good manners to give our users plenty of warning if we plan to remove features that they might depend upon in our next major release. For this reason, many developers will implement deprecation warnings to alert users that the API is due to change in future, and that they should update their code accordingly. For example:
PYTHON
import warnings
def myfunc():
warnings.warn(
"myfunc is deprecated, and wil be removed in version 4",
DeprecationWarning,
)
return 42
When adding deprecations to our code, we should update the minor version, and include them in our changelog. When we follow through on our deprecations and remove features, this should also be clearly stated in the changelog of our major release.
Extra: Advanced Versioning
This section may be skipped.
There are many more fine-grained versioning techniques that may be
employed as our projects grow in complexity, although these are not
universally adopted and may only be applicable to very large projects
with a detailed review process. The full list of version types accepted
by tools such as pip
is listed in PEP 440, while the formal
specification for semantic versioning can be found at semver.org. For example, we may provide
pre-release specifiers:
- 1.1.0a0: The first alpha version of 1.1.0. This is intended for use by developers of the project and (perhaps) a small group of users, and may be subject to large changes. 1.1.0a0 comes after 1.0.N, but before 1.1.0. A second alpha version may be listed as 1.1.0a1.
- 1.1.0b0: The beta version of 1.1.0. This follows 1.1.0aN, and is intended for use by a wider pool of users for further testing and feedback. This is usually close to the finished product, but some large changes remain possible.
- 1.1.0rc0: The release-candiate version of 1.1. Sometimes called a ‘preview’ version, it is not expected that further significant changes will be made in version 1.1, but some small changes may be included. It comes after 1.1.0bN, but before 1.1.0.
The number following the pre-release specifier is optional, with no
number interpretted as 0 (for example, 1.0.0a == 1.0.0a0
).
A dot, hyphen, or underscore separator is also allowed, such as
1.0.0-a1
, 1.1.0.beta.3
, or
1.1.0-rc.2
. We may also make post-releases if we
wish to make minor edits after a release:
- 1.1.0post0: An update after a release that does not change the distributed software. For example, a change in documentation or release notes. It comes after 1.1.0 but before 1.1.1.
Similarly, post-release specifiers may have dot, hypen or underscore separators, and may exclude the trailing number. The use of ‘rev’ or ‘r’ in place of ‘post’ is also common. Note that a post-release is not the appropriate place for a quick bug fix after a full release – that should be an update to the patch number.
Finally, it is possible to create local versions in cases where a
developer has made progress on a new development but has not finalised
their work in a new release. If you use a tool such as Git to control
your versioning, it may automatically generate the local version. In
general, there is no ordering to local versions. A local version is
specified with a +
followed by a dot-separated list of
numbers, letters, and hyphens, for example 1.1.0+001
or
1.2.0-alpha-630-g60eca14
.
Preparing to Publish
README Files
Before sharing our software with the world, it is a good idea to let
others know what it is and how it works! A good README file should
explain what a project is for, how users can install it, and how to use
it. For example, we may use the following file README.md
,
written using markdown:
MARKDOWN
# epi_models
A collection of Python utilities for solving epidemiology problems.
## Installation
The project can be installed using \`pip\`:
```
$ pip install epi_models
```
To install from this repo:
```
$ git clone github.com/username/epi_models
$ cd epi_models
$ pip install .
```
## Usage
The project offers a command line interface, which can be used as
follows:
```
$ epi_models SIR input.yaml
```
`SIR` can be exchanged for a model of your choice, and `input.yaml`
is an input file with the following entries...
This should be included at the top level of our project:
📁 epi_models
|
|____📜 pyproject.toml
|____📜
README.md
|____📦 epi_models
|
|____📜
__init__.py
|____📜 __main__.py
|____📁 models
|____📁 plotting
It should be included in our package metadata by adding the following
line in our pyproject.toml
:
Licensing
It is important for our software to have a license so that any potential users know what their rights are with regards to usage and redistribution. This can also provide us with legal protections in some cases.
There are several open source licenses available, and they can be
applied to our project simply by adding a LICENSE
,
LICENSE.txt
, or LICENSE.md
file to the top
level of our project:
📁 epi_models
|
|____📜 pyproject.toml
|____📜
README.md
|____📜 LICENSE.md
|____📦 epi_models
|
|____📜 __init__.py
|____📜 __main__.py
|____📁
models
|____📁 plotting
One of the simplest and most widely used licenses is the MIT License, which is very permissive. It requires users of your software to retain its copyright notice if they redistribute or modify it (or ‘substantial portions’ of it), but otherwise allows users to do what they wish. It also ensures the author is not liable for anything the users do with their software:
MIT License
Copyright (c) [year] [fullname]
Permission is hereby granted, free of charge, to any person obtaining a copy
of this software and associated documentation files (the "Software"), to deal
in the Software without restriction, including without limitation the rights
to use, copy, modify, merge, publish, distribute, sublicense, and/or sell
copies of the Software, and to permit persons to whom the Software is
furnished to do so, subject to the following conditions:
The above copyright notice and this permission notice shall be included in all
copies or substantial portions of the Software.
THE SOFTWARE IS PROVIDED "AS IS", WITHOUT WARRANTY OF ANY KIND, EXPRESS OR
IMPLIED, INCLUDING BUT NOT LIMITED TO THE WARRANTIES OF MERCHANTABILITY,
FITNESS FOR A PARTICULAR PURPOSE AND NONINFRINGEMENT. IN NO EVENT SHALL THE
AUTHORS OR COPYRIGHT HOLDERS BE LIABLE FOR ANY CLAIM, DAMAGES OR OTHER
LIABILITY, WHETHER IN AN ACTION OF CONTRACT, TORT OR OTHERWISE, ARISING FROM,
OUT OF OR IN CONNECTION WITH THE SOFTWARE OR THE USE OR OTHER DEALINGS IN THE
SOFTWARE.
Information on alternative open source licenses can be found at choosealicense.com. Note that your choice of license may be determined by your dependencies. For example, if your code uses software licensed under the GNU GPLv3.0 license, it too should be licensed under GPLv3.0.
We should include our license in our pyproject.toml
file
as follows:
We can instead simply state the name of the license:
If you’re developing code on behalf of an organisation, it’s worth checking in with their legal team (or at the very least, your supervisor) before deciding on a license.
Publishing our Software on GitHub
git
is a powerful version control tool that allows us to
track changes to our source code over time. This lesson is not the place
for teaching how to use git
, but it is highly recommended
that you use it for managing your Python projects.
GitHub is an online service for hosting git
-based
software projects, and it is a great way to share our code and
collaborate with others.
Once our code is hosted on GitHub, we can create releases and assign them a tag. This tag should be the version number of the release. We will see later how to use GitHub Actions to automatically publish our code on other services whenever a new release is created.
Whenever we make new releases of our code, we should write a detailed changelog that describes all of the changes since the last version. This should include new features, bug fixes, removals, and deprecations. A good changelog document should include all code changes in reverse chronological order (so the latest changes should be first in the list), and should credit anybody who contributed to the code.
With our code hosted on GitHub, it is possible for others to install
it using pip
:
However, this will simply get the latest commit to the main branch,
which may be a work-in-progress and not a true release. We can specify a
particular release by providing the version number after an
@
sign:
Note that we can also supply a branch name or a commit hash here:
BASH
$ pip install "git+https://github.com/user/project@branch"
$ pip install "git+https://github.com/user/project@1a2b3c4"
Projects hosted on GitHub can also be added to dependencies in
pyproject.toml
as so:
However, it is relatively slow for pip
to install in
this way, as it must clone the whole repository. We can instead install
from a snapshot by pointing pip
towards an archived
.zip
:
However, this is still slower than installing from a dedicated Python
package repository such as PyPI, which hosts pre-built .whl
files, and it only allows our users to specify a single version of our
code. This means that they may miss out on crucial patch releases. In
general, it is a good idea to use GitHub to host our source code and to
manage its development, and to use a service like PyPI to host packages
that our users can install.
Extra: Consistent Versioning with setuptools-scm
An issue with using GitHub to create new releases is that the project version can easily become desynced. The version needs to be specified in three places:
-
git
tags, determined by releases on GitHub - The
version
field inpyproject.toml
-
__version__
in our__init__.py
It is possible to have all three determined by git
tags
using setuptools-scm
. This can be set as a requirement of
the build system:
TOML
# file: pyproject.toml
[build-system]
requires = [
"setuptools >= 65",
"setuptools_scm[toml]",
"wheel",
]
build-backend = "setuptools.build_meta"
With this, we no longer need to provide an entry for
project.version
, and instead should add:
TOML
# file: pyproject.toml
[project]
name = "epi_models"
# version = "1.2.3" # no longer needed!
dynamic = ["version"]
Following this, we should add the following section elsewhere in
pyproject.toml
:
Now, when we install or build the project,
setuptools-scm
will create a new file
_version.py
inside of our built package. If our git tag is
1.2.3
, this will contain:
PYTHON
# file generated by setuptools_scm
# don't change, don't track in version control
__version__ = version = '1.2.3'
__version_tuple__ = version_tuple = (1, 2, 3)
This can be retrieved at runtime by adding the following to
__init__.py
:
PYTHON
# file: __init__.py
from importlib.metadata import version, PackageNotFoundError
try:
__version__ = version("epi_models")
except PackageNotFoundError:
# If the package is not installed, don't add __version__
pass
Note that importlib.metadata
was added to the Python
standard library in version 3.8. Earlier versions will need to instead
load an external package importlib_metadata
, which works in
the same way. We can account for both using:
PYTHON
# file: __init__.py
try:
from importlib.metadata import version, PackageNotFoundError
except ImportError:
from importlib_metadata import version, PackageNotFoundError
We’ll also need to account for importlib_metadata
in our
pyproject.toml
as follows:
TOML
# file: pyproject.toml
[project]
dependencies = [
'importlib_metadata; python_version < "3.8"',
]
Now, our Git tags, __version__
, and
pyproject.toml
version
will automatically be
kept in sync.
PyPI, the Python Packaging Index
PyPI is the official package
repository for the Python community. It is equivalent to CRAN, used for
the R programming language. This is the repository that pip
uses when we install remote packages from the command line. It is
recommended to upload packages here if we want our projects to reach a
wider audience.
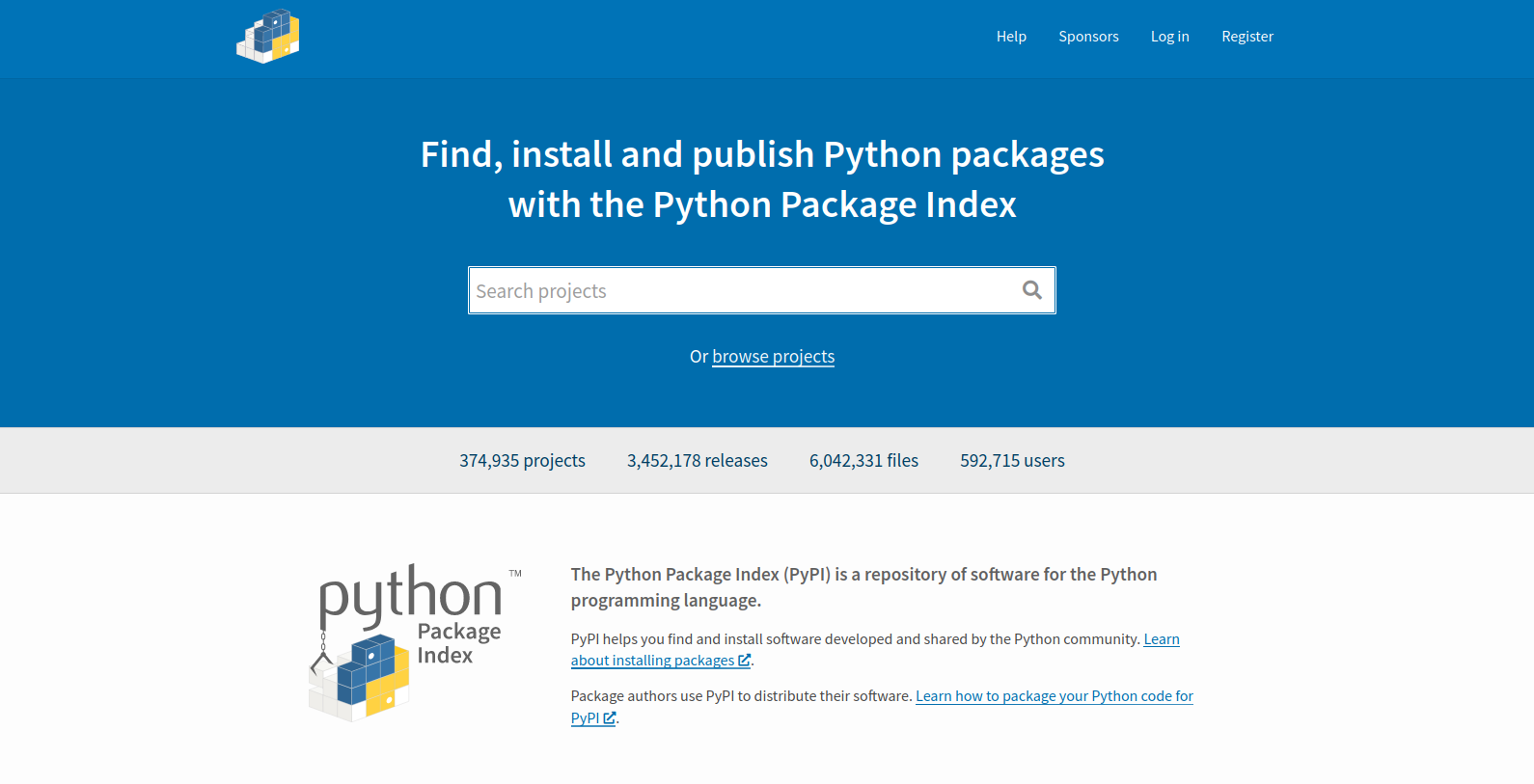
To begin, you will need to create an account on PyPI and its sister-site, TestPyPI – the latter is used to check that our packages have been uploaded and set up properly before committing to the standard repository.
To help people find our project after it’s uploaded, we should add
some keywords they can search for. We can also add ‘classifiers’ that
categorise our project and describe its intended audience. A list of
possible classifiers are available on the PyPI website. These should be
added to our pyproject.toml
:
TOML
# file: pyproject.toml
[project]
# keywords should contain search terms so users can
# find our project
keywords = [
"epimediology",
"virology",
"SIR",
"SEIR",
"Compartmental",
"Model",
]
# Classifiers are a standard set of categories for
# our project, including which level of development
# we're at, who our intended audience is, which
# license we're using, etc.
classifiers = [
"Development Status :: 3 - Alpha",
"Intended Audience :: Science/Research",
"License :: OSI Approved :: MIT License",
"Natural Language :: English",
"Operating System :: OS Independent",
"Programming Language :: Python :: 3.8",
"Topic :: Scientific/Engineering",
]
Before discussing how to get our project hosted on PyPI, we’ll quickly discuss ‘wheel’ files, which are the standard way for Python to distribute packages.
Wheel files
When we install packages using pip
, it first creates a
‘wheel’ file, with the file extension .whl
. For
example:
... installs dependencies ...
Building wheels for collected packages: epi-models
Building wheel for epi-models (pyproject.toml) ... done
Created wheel for epi-models: filename=epi_models-0.1.0-py3-none-any.whl size=5378 sha256=005bcad72e7f6cc773f4180960e402e3bdbdf0fcb6fb683a019d9521775edfc8
Stored in directory: /tmp/pip-ephem-wheel-cache-69r0h48p/wheels/d9/4d/ac/bbc1437fd83635787dd0fb8c3df8da61fc7b57f6eaa2b0d17b
Successfully built epi-models
Installing collected packages: epi-models
Successfully installed epi-models-0.1.0
The installation goes through several steps:
-
pip
analyses our package and creates a.whl
file. - The
.whl
file is stored in a temporary directory. - The package is installed to our system from the
.whl
file.
After installation, we can find our installed package at
/path/to/my/env/lib/python3.8/site-packages/epi_models
, and
within we’ll find that every file has been compiled to Python bytecode,
contained within __pycache__
directories. So what is a
wheel file, and how does it help with this process?
A wheel is a standard package distribution format, defined in PEP 427. It is essentially
a .zip
file with a descriptive name that contains our
package. When we installed our package above it created the following
wheel file:
epi_models-0.1.0-py3-none-any.whl
The filename has seveal components separated by dashes. In order, these are:
- The package name
- The package version
- Language implementation, such as
py3
,py27
, orpy2
. Packages that are compatible with any Python version will bepy2.py3
. Packages that are only compatible with a particular Python implementation, such as CPython, may be listedcpy38
. - The ABI tag, which stands for ‘Application Binary Interface’. This
specifies the binary compatibility of the CPython API, and may take a
value such as
abi3
. This won’t be relevant unless you’re distributing compiled extensions written in C, and will benone
if your package is pure Python. This is beyond the scope of these lessons. - Platform tag, which specifies which operating system your package is
compatible with.
any
means it will run on Windows, Mac or Linux, while it may say something likemacosx_10_9_x86_64
if it includes compiled C extensions for Mac OSX 10.9 on an x86_64 architecture. Themanylinux
tag encompasses many different popular Linux distros.
When we upload our package to PyPI, we will do so in the form of a
wheel file, which contains all Python files in our package along with
any compiled binaries for extensions written in C. When a wheel file is
installed, Python files are compiled to bytecode, and the package is
installed to the site-packages
of our environment.
As the wheel file contains compatibility information in its name,
pip
can quickly check to see whether any wheels uploaded to
PyPI are compatible with our system whenever we try to install
something. In order to meet the needs of as many users as possible, it’s
also possible for us to upload multiple wheels for each release – one
for each targeted Python version and operating system.
build
and twine
So how do we create a wheel file to upload? The standard tool used to
create wheel files is build
:
As we already have a pyproject.toml
, build
has everything it needs to create a wheel file. It can be called simply
using:
This will create a new directory ./dist
containing the
following:
📁 dist
|
|____📜
epi_models-0.1.0-py3-none-any.whl
|____📜
epi_models-0.1.0.tar.gz
We can see the contents of the .whl
file by unzipping
it:
We’ll see that it contains our package along with a second directory
epi_models-0.1.0.dist-info
, which contains package metadata
such as our license and README file. Note that we should remove the
unzipped directories from ./dist
before the upload
stage.
The second file build
created is a .tar.gz
file – a gzip-compressed tarball. This is a ‘source distribution’, which
is used as a backup by pip
if it can’t find a suitable
wheel file to install.
The tool for uploading our package to PyPI is twine
:
We can check that our package is well-formed by running
twine check
:
If this returns that the package is okay, we can test the distribution of our package by uploading to TestPyPI:
Note that there is a registration process for TestPyPI, and a
separate one for PyPI. Once uploaded to TestPyPI we can check that
everything looks correct using the web interface. If you choose to
pip
download from TestPyPI you may need to specify that the
dependencies are gotten from PyPI proper by using the
--extra-indec-url
flag. This is because the dependencies
may not be available through TestPyPI.
BASH
$. pip install -i https://test.pypi.org/pypi/ --extra-index-url https://pypi.org/simple epi_models==0.1.0
Once we are happy that all looks correct on TestPyPI we may proceed with installing our package to PyPI:
That’s all we need! Users will then be able to install our package to any machine by calling the following:
Similarly, it can be included as a dependency to other Python projects:
As we continue to develop our software, we should upload each new version to PyPI. Our users will then be able to request a particular version:
Extra: Automating Package Publishing with GitHub Actions
We saw earlier how to ensure that the version of our package was stored only in the Git tags. We can similarly set up our GitHub project to automatically publish our package whenever we make a new release. We can use GitHub Actions for this, which is a service used for continuous integration. It can achieve tasks such as:
- Automatically run tests on a variety of platforms each time a new commit is pushed or version is published.
- Enforce a code style automatically.
- Publish work on each release.
GitHub Actions are controlled using a YAML file. Though the full specification is beyond the scope of this course, the following file will cause GitHub to automatically upload our package to PyPI with each new release:
YAML
# Provide a name for the workflow
name: Upload Python Package
# Tell GitHub when to run the action
# This will run every time a new release is published
on:
release:
types: [published]
jobs:
deploy:
# Run the workflow on the latest Ubuntu version
runs-on: ubuntu-latest
steps:
# This will checkout our GitHub project and enter
# the directory
- uses: actions/checkout@v3
# This will set up a Python environment
- name: Set up Python
uses: actions/setup-python@v4
with:
python-version: '3.x'
# Here we update pip to the latest version and
# install 'build'. We won't need 'twine' here.
- name: Install dependencies
run: |
python -m pip install --upgrade pip
pip install build
# Here we run build to create a wheel and a
# .tar.gz source distribution.
- name: Build package
run: python -m build --sdist --wheel
# Finally, we use a pre-defined action to publish
# our package in place of twine.
- name: Publish package
uses: pypa/gh-action-pypi-publish@release/v1
with:
user: __token__
password: ${{ secrets.PYPI_API_TOKEN }}
This should be included in the file
.github/workflows/publish.yml
, where the
.github
directory is located at the top level of our
project (at the same level as pyproject.toml
). In order for
this to work, we’ll need to set up a PYPI_DEPLOYMENT_TOKEN
in the ‘secrets’ section of our project’s GitHub settings. A guide to
doing this may be found on the PyPI website.
With this set up, our projects will be automatically pushed to PyPI each time we make a new release.