Content from Introduction
Last updated on 2025-01-09 | Edit this page
Overview
Questions
- What is NLP?
- What are real-world applications of NLP?
- Which problems NLP solves best?
- What is language from a NLP perspective?
- How does NLP relates to Deep Learning and Machine Learning?
Objectives
- Define Natural Language Processing
- Detailing classic NLP tasks and applications in practice
- Describe the theoretical perspectives that the field of NLP draws upon, including linguistics (syntax, semantics, and pragmatics), Deep Learning and Machine Learning
What is NLP?
Natural language processing (NLP) is an area of research and application that focuses on making natural (i.e., human) language accessible to computers so that they can be used to perform useful tasks. Research in NLP is highly interdisciplinary, drawing on concepts from computer science, linguistics, logic, mathematics, psychology, etc. In the past decade, NLP has evolved significantly with advances in technology, especially in the field of deep learning, to the point that it has become embedded in our daily lives.
Let’s start by looking at some popular applications you use in everyday life that have some form of NLP component.
NLP in the real world
Name three to five tools/products that you use on a daily basis and that you think leverage NLP techniques. To solve this exercise you can get some help from the web.
These are some of the most popular NLP-based products that we use on a daily basis:
- Voice-based assistants (e.g., Alexa, Siri, Cortana)
- Machine translation (e.g., Google translate, Amazon translate)
- Search engines (e.g., Google, Bing, DuckDuckGo)
- Keyboard autocompletion on smartphones
- Spam filtering
- Spell and grammar checking apps
The exercise above tells us that a great deal of NLP techniques is embedded in our daily life. Indeed NLP is an important component in a wide range of software applications that we use in our daily lives.
Core applications
Email providers use NLP in several ways: automatically detect and filter-out spam emails, classify important emails (e.g., Priority inbox), recognise dates and events to automatically add them to your calendar and suggesting you phrases while you’re typing
Voice-based assistants use NLP to recognise speech, interpret requests (e.g., “Set alarm for 8 AM tomorrow”) and perform it accurately, translate spoken language in real time and store individual preferences and history to tailor responses based on the past activities with the user
Search engines use NLP to interpret the meaning behind user queries (e.g., “What’s the best restaurant near me?”), pull and highlight key information directly from a webpage to answer your query and personalise results based on user history
Other types of applications
Customer care services use NLP to summarise and understand user reviews to provide actionable insights to their companies
Spelling and grammar correction tools use NLP to highlight typos or errors and suggest the most valid alternative
The Historical Archives of the European Parliament have used NLP to instantly search, retrieve and understand decades of legislative documents and parliamentary proceedings in multiple languages
NLP tasks
Language modeling: Given a sequence of words, the model predicts the next word. For example, in the sentence “The cat is on the _____”, the model might predict “mat” based on the context. This task is useful for building solutions that require speech and optical character recognition (even handwriting), language translation and spelling correction
Text classification: Given a set of items (e.g., emails), assign a label (e.g., spam/not-spam). It is the task of assigning predefined categories or labels to a given text. Text classification is extremely popular in NLP applications, from spam filtering to movies ratings based on reviews.
Information extraction: This is the task of extracting relevant information from the text. “Eva Viviani, a Research Software Engineer at the eScience Center, attended the 17th Conference of the European Chapter of ACL on May 2nd, 2023”. Person: Eva Viviani, Job title: RSE, Event: 17th Conference of the European Chapter of ACL, Date: May 2nd, 2023, etc.
Information retrieval: This is the task of finding relevant information or documents from a large collection of unstructured data based on user’s query, e.g., “What’s the best restaurant near me?”.
Conversational agent (also known as ChatBot): Building a system that interacts with a user via natural language, e.g., “What’s the weather today, Siri?”. These agents are widely used to improve user experience in customer service, personal assistance and many other domains.
Topic modelling: Automatically identify abstract “topics” that occur in a set of documents, where each topic is represented as a cluster of words that frequently appear together. This task is used in a variety of domains, from literature to bioinformatics as a common text-mining tool.
Natural vs Artificial Language
Why does NLP have “natural” in its name? A Language is a structured system of communication that consists of grammar and vocabulary. Within this definition, in NLP we refer to human language as Natural to contrast it to artificial languages which are formal languages. The reason for this is that many experts believe that natural languages have emerged naturally tens of thousands of years ago and have evolved ever since. Formal languages, on the other hand, are languages that have been engineered by humans and have rigid and explicitly defined rules.
To understand this perspective, let’s consider for instance Python or R. These are programming languages that have explicit, clear grammatical and syntactic rules. This means that within the realm of those programming languages, there is no room for ambiguity, otherwise your code would allow for different behaviours depending on the situation, or the machine. This is not the case for human languages.
Ambiguity
Natural language is highly creative and often ambiguous, among many other complex traits. A sentence of the type “I saw a bat” may mean many things depending on who is hearing/saying it, where and when it is pronounced. The disambiguation of meaning is usually a by-product of the context in which sentences are pronounced and the historic accumulation of interactions which are transmitted across generations (think for instance to idioms – these are usually meaningless phrases that acquire meaning only if situated within their historical and societal context). These characteristics make NLP a particularly challenging field to work in.
We cannot expect a machine to process human language and simply understand it as it is. We need a systematic, scientific approach to deal with it. It’s within this premise that the field of NLP is born, primarily interested in converting the building blocks of human/natural language into something that a machine can understand. We’ll see what does this mean in the next episode.
The image below shows you the building blocks of language and a few NLP applications that leverage this type of information.
Each building block of human language carries a large amount of information, which we process quickly and effortlessly. Some of this information is still being studied by scientists because it’s unclear how to measure it, whether the human brain uses it at all to aid understanding, and, if so, to what extent. A lot of research effort is spent on this problem in academia, and it’s important to keep in mind that we are still far from solving it.
How do we make language then understandable for machines? How do we expose and exploit the statistical information within the human language? The field of NLP focuses exactly on these challenges. The ultimate goal is to make this information available to computers, so that they can use it to understand language as closely as possible to the way we (humans) do.
Discreteness
NLP is a subfield of Artificial Intelligence that intersects with Deep Learning and more broadly with Machine Learning. There are many concepts in NLP that indeed draw upon those fields. For instance the task of categorising text as positive or negative, is a classification problem that has been formulated and solved also in the Deep Learning realm. What’s the difference then between classifying which species a penguin belongs to (based on their pictures) and understand the difference between “cat” and “sat”?
If you take an image of a penguin and change a pixel it will still be recognised as the same penguin as before. This tiny change has resulted in a small change that did not affect the whole picture. If you change one letter of a word, however, as in cat vs sat, then even if the difference for the computer is a single bit, the two things in the human language are two separate, discrete concepts. They just happen (for historical reasons or just by chance) to have similar spellings.
The reason why NLP is a distinct field is that, unlike images and sounds (which are typically handled in Deep Learning and are continuous data), words are discrete units. This characteristic of human language demands a completely different approach because, while computers excel at processing continuous variables, they struggle with the discrete nature of language. In the next episode, we’ll explore how a solution to this challenge has only recently been developed.
Key Points
- NLP is embedded in numerous daily-use products
- Key tasks include language modeling, text classification, information extraction, information retrieval, conversational agents, and topic modeling, each supporting various real-world applications.
- NLP is a subfield of Artificial Intelligence (AI) that deals with approaches to process, understand and generate natural language
- Deep learning has significantly advanced NLP, but the challenge remains in processing the discrete and ambiguous nature of language
- The ultimate goal of NLP is to enable machines to understand and process language as humans do, but challenges in measuring and interpreting linguistic information still exist.
Content from Episode 1: From text to vectors
Last updated on 2025-01-09 | Edit this page
Overview
Questions
- Why do we need to prepare a text for training?
- How do I prepare a text to be used as input to a model?
- What different types of pre processing steps are there?
- How do I train a neural network to extract word embeddings?
- What properties word embeddings have?
- What is a word2vec model?
- How do we train a word2vec model?
- How do I get insights regarding my text, based on the word embeddings?
Objectives
After following this lesson, learners will be able to:
- Implement a full preprocessing pipeline on a text
- Use Word2Vec to train a model
- Inspect word embeddings
Introduction
In this episode, we’ll train a neural network to obtain word
embeddings. We will only briefly touch upon the concepts of
preprocessing
and word embedding
with
Word2vec.
The idea is to get you over a practical example first, without diving into the technical or mathematical intricacies of neural networks and word embeddings. The goal for this episode, in fact, is for you to get an intuition of how computers represent language. This is key to understand how NLP applications work and what are their limits.
In the later episodes we will build upon this knowledge to go deeper into all of these concepts and see how NLP tools have evolved more complex language representations.
In this episode, we will build a workflow following these steps:
- Formulate the problem
- Download the input data
- Prepare data to be ingested by the model (i.e. preprocessing step)
- Train the model
- Load the embeddings and inspect them
Note that for step 5 we will cover only briefly the code to train your own model, but then we will load the output of already pretrained models. That is because training requires a large amount of data and considerable computing resources/time which are not suitable for a local laptop/computer.
1. Formulate the problem
In this episode we will be using Dutch newspaper texts to train a Word2Vec model to investigate the notion of semantic shift.
Semantic shift
Semantic shift, as it is used here, refers to a pair of meanings A and B which are linked by some relation. Either diachronically (e.g., Latin caput “head” and Italian capo “chief”) or synchronically, e.g. as two meanings that co-exist in a word simoultaneously (English “head”, as in “I have covered my head with a hat” and as in “I am the head of the department”). Can we detect a semantic shift? – We’ll tackle this phenomenon in this episode.
Newspapers make an interesting dataset for investigating this phenomenon, as they contain information about current events and the language it uses is clear and reflective of its time. We will specifically look at the evolution of specific words in Dutch across a period of time from 1950 to 1990. In order to do that, we need to train a model to extract the meaning of every single word and track in which context they occur, over decades.
Goal
The goal is to analyze the semantic shift of specific Dutch words from 1950 to 1989 using newspapers as a dataset.
Conceptually, the task of discovery of semantic shifts in our newspaper data can be formulated as follows:
Given newspaper corpora [C1, C2, C3, …] containing texts created in time periods from 1950s to 1980s, considered as four decades [1: 50-60; 2: 60-70; 3: 70-80; 4: 80-90], the task is to detect how some words have shifted in meaning across those decades.
As a test-bed, we’re going to focus on three words:
mobiel
, televisie
and ijzeren
.
These words exemplify very well the notion of semantic evolution /
semantic shift, as their meaning has gained new nuances due to social,
technological, political and economic changes occurred in those key
years.
We’re going to use a model to solve this task. We’re going to see which one and how in a moment.
Our dataset is provided by Delpher (developed by the KB - the National Library of the Netherlands) which contains digitalised historic Dutch newspapers, books, and magazines. This online newspaper collection covers data spanning from 1618 up to 1995 and of many local, national and international publishers.
We will load only a page to go step-by-step through what it takes to train a model. This makes it easier to know what’s going on. However, in practice, when to successfully train a model you need larger quantities of data to allow the model to get more precise and accurate representations. In those cases you will simply condense each of the steps we cover next into one code, to do all these steps at once.
Dataset size in training
To obtain high-quality embeddings, the size/length of your training dataset plays a crucial role. Generally tens of thousands of documents are considered a reasonable amount of data for decent results.
Is there however a strict minimum? Not really. Things to keep in mind
is that vocabulary size
, document length
and
desired vector size
interacts with each other. The higher
the dimensional vectors (e.g. 200-300 dimensions) the more data is
required, and of high quality, i.e. that allows the learning of words in
a variety of contexts.
While word2vec models typically perform better with large datasets containing millions of words, using a single page is sufficient for demonstration and learning purposes. This smaller dataset allows us to train the model quickly and understand how word2vec works without the need for extensive computational resources.
For the purpose of this episode and to make training easy on our laptop, we’ll train our word2vec model using just one page. Subsequently, we’ll load pre-trained models for tackling our task.
Exploring Delpher
Before we move further with our problem, take your time to explore Delpher more in detail. Go to Delpher and pick a newspaper of a particular date. Did you find anything in the newspaper that is interesting or didn’t know yet? For example about your living area, sports club, or an historic event?
The 20th of July 1969 marks an important event. The First Moon landing! Look at what the Tubantia newspaper had to say about it only four days afterwards.
The Cuban Missile Crisis, also known as the October Crisis in Cuba, or the Caribbean Crisis, was a 13-day confrontation between the governments of the United States and the Soviet Union, when American deployments of nuclear missiles in Italy and Turkey were matched by Soviet deployments of nuclear missiles in Cuba. The crisis lasted from 16 to 28 October 1962. See what de Volkskrant published on the 24th of October, 1962. Can you see what they have organised in Den Haag related to this event?
2. Download the data
We download a page from the journal Algemeen
Dagblad of July 21, 1969 as txt
and save it as
ad.txt
. We then load this file and store it in a variable
called corpus
.
Callout
The txt
file provides the text without formatting and
images, and is the product of a technique called Optical Character
Recognition (OCR). This is a technique in which text from an image is
converted into text, and it’s a necessary step for any scanned image to
obtain plain text. Luckily for us, Delpher has already done this step
for us so that we can directly use the txt. However, take into
consideration that if you start from an image that contains text, you
may need an additional preprocessing step.
Inspect the data
We inspect the first line of the imported text:
'MENS OP MAAN\n„De Eagle is geland” Reisduur: 102 uur, Uitstappen binnen 20 iuli, 21.17 uur 45 min. en'
We can see that although the OCR applied to the original image has
given a pretty good result, there are mistakes in the recognized text.
For example, on the first line the word juli
(july) has
misinterpreted as iuli
.
Note also the size of the text:
There are 12354
characters inside the corpus. Note also
the type of file:
Python tells us that corpus
is a str
,
i.e. a string. This means that every single character in the text (even
blank spaces) is a unit for our computer. However, what’s really
important for us is that the machine gets the meaning of the
words contained in the text. That is, that is able to
understand which characters belong together to form a word, and what
instead is something else: Punctuation, conjunctions, articles, or
prepositions.
How do we teach our machine to segment the text and keep
only the relevant words? This is where data preprocessing
comes into play. It prepares the text for efficient processing by the
model, allowing it to focus on the important parts of the text that
contribute to understanding its meaning.
3. Prepare data to be ingested by the model (preprocessing)
NLP models work by learning the statistical regularities within the constituent parts of the language (i.e, letters, digits, words and sentences) in a text. However, text contains also other type of information that humans find useful to convey meaning. To signal pauses, give emphasis and convey tone, for instance, we use punctuation. Articles, conjunctions and prepositions also alter the meaning of a sentence. The machine does not know the difference among all of these linguistic units, as it treats them all as equal. Also, the decision to remove or retain these parts of text is quite crucial for training our model, as it affects the quality of generated word vectors.
Examples of preprocessing steps are:
- Cleaning the text: remove symbols/special characters, or other things that “sneaked” into the text while loading the original version.
- Lowercasing
- Removing punctuation
- Stop word removal, where you remove prepositions, conjuctions and articles
- Tokenization: this means segmenting the text by retaining groups of
characters. These groups are referred to as
tokens
and their size can vary from entire words to lemmas, or subword components (e.g. morphemes) - Part of speech tagging: the process of labelling the grammatical role of a word, e.g. nouns and verbs.
Callout
Preprocessing approaches affect significantly the quality of the training when working with word embeddings. For example, [Rahimi & Homayounpour (2022)] (https://link.springer.com/article/10.1007/s10579-022-09620-5) demonstrated that for text classification and sentiment analysis, the removal of punctuation and stopwords leads to higher performance.
You do not always need to do all the preprocessing steps, and which ones you should do depends on what you want to do. For example, if you want to extract entities from the text using named entity recognition, you explicitly do not want to lowercase the text, as capitals are a component in the identification process.
Preprocessing can be very diffent for different languages. This is both in terms of which steps to apply, but also which methods to use for a specific step.
Let’s apply a number of preprocessing steps to extract a list of words from the newspaper page.
1. Cleaning the text
We start by importing the spaCy
library that will help
us go through the preprocessing steps. SpaCy is a popular open-source
library for NLP in Python and it works with pre-trained languages models
that we can load and use to process and analyse the text
efficiently.
We need to install en_core_web_sm
because the text we’re
dealing with it’s in Dutch This is a small pre-trained language model from Spacy containing
essential components like vocabulary, syntax, and entities specifically
for the Dutch language.
We can then load the model into the pipeline function. This function connects the pretrained model to various preprocessing steps, including the tokenisation.
Next, we’ll eliminate the triple dashes that separate different news articles, as well as the vertical bars used to divide some columns.
PYTHON
# filter out triple dashes and vertical bars
filtered_tokens = [token.text for token in doc if token.text != "---" and token.text != "|"]
# join units back into a cleaned string
corpus_clean = ' '.join(filtered_tokens)
print(corpus_clean[:100])
MENS OP MAAN „ De Eagle is geland ” Reisduur : 102 uur , Uitstappen binnen 20 iuli , 21.17 uur 45
2. Lowercasing
Our next step is to lowercase the text. Our goal here is to generate a list of unique words from the text, so in order to not have words twice in the list - once normal and once capitalised when it is at the start of a sentence for example - we can lowercase the full text.
mens op maan \n „ de eagle is geland ” reisduur : 102 uur , uitstappen binnen 20 iuli , 21.17 uur 45 [...]
Callout
It is important to keep in mind that in doing this, some information is lost. As mentioned before, models that are trained to identify named entities use information on capitalisation. As another example, there are a lot of names and surnames that carry meaning. “Bakker” is a common Dutch surname, but is also a noun (baker). In lowercasing the text you loose the distinction between the two.
Next we move to tokenise our text.
4. Tokenisation
Tokenisation is essential in NLP, as it helps to create structure
from raw text. It involves the segmentation of the text into smaller
units referred as tokens
. Tokens can be sentences
(e.g. 'the happy cat'
), words
('the', 'happy', 'cat'
), subwords
('un', 'happiness'
) or characters
('c','a', 't'
). The choice of tokens depends by the
requirement of the model used for training, and the text. This step is
carried out by a pre-trained model (called tokeniser) that has been
fine-tuned for the target language. In our case, this is
en_core_web_sm
loaded before.
Callout
A good word tokeniser for example, does not simply break up a text based on spaces and punctuation, but it should be able to distinguish:
- abbreviations that include points (e.g.: e.g.)
- times (11:15) and dates written in various formats (01/01/2024 or 01-01-2024)
- word contractions such as don’t, these should be split into do and n’t
- URLs
Many older tokenisers are rule-based, meaning that they iterate over a number of predefined rules to split the text into tokens, which is useful for splitting text into word tokens for example. Modern large language models use subword tokenisation, which are more flexible.
PYTHON
spacy_corpus = nlp(corpus_clean)
# Get the tokens from the pipeline
tokens = [token.text for token in spacy_corpus]
tokens[:10]
['mens', 'op', 'maan', '\n ', '„', 'de', 'eagle', 'is', 'geland', '”']
As one can see the tokeniser has split each word in a token, however
it has considered also blank spaces \n
and also
punctuation.
5. Remove punctuation
The next step we will apply is to remove punctuation. We are interested in training our model to learn the meaning of the words. This task is highly influenced by the state of our text and punctuation would decrease the quality of the learning as it would add spurious information. We’ll see how the learning process works later in the episode.
The punctuation symbols are defined in:
We can loop over these symbols to remove them from the text:
PYTHON
# remove punctuation from set
tokens_no_punct = [token for token in tokens if token not in string.punctuation]
# remove also blank spaces
tokens_no_punct = [token for token in tokens_no_punct if token.strip() != '']
['mens', 'op', 'maan', 'de', 'eagle', 'is', 'geland', 'reisduur', '102', 'uur']
Visualise the tokens
This was the end of our preprocessing step. Let’s look at what tokens we have extracted and how frequently they occur in the text.
PYTHON
import matplotlib.pyplot as plt
from collections import Counter
# count the frequency of occurrence of each token
token_counts = Counter(tokens_no_punct)
# get the top n most common tokens (otherwise the plot would be too crowded) and their relative frequencies
most_common = token_counts.most_common(100)
tokens = [item[0] for item in most_common]
frequencies = [item[1] for item in most_common]
plt.figure(figsize=(12, 6))
plt.bar(tokens, frequencies)
plt.xlabel('Tokens')
plt.ylabel('Frequency')
plt.title('Token Frequencies')
plt.xticks(rotation=90)
plt.tight_layout()
plt.show()
As one can see, words in the text have a very specific skewed distribution, such that there are few very high-frequency words that account for most of the tokens in text (e.g., articles, conjunctions) and many low frequency words.
Challenge
Discuss with each other:
- For which NLP tasks can punctuation removal be applied?
- For which tasks is punctuation relevant and should punctuation not be removed?
- Laura left the solution here missing – could this be considered an exercise without a solution?
6. Stop word removal
For some NLP tasks only the important words in the text are needed. A
text however often contains many stop words
: common words
such as de
, het
, een
that add
little meaningful content compared to nouns and words. In those cases,
it is best to remove stop words from your corpus to reduce the number of
words to process.
Tasks where stop word removal is useful
NLP tasks for which stop word removal can be applied are for example
text classification
or topic modelling
. When
clustering words into topics, stop words are irrelevant. Having fewer
and more relevant words gives better results. For other tasks, such as
text generation
or question answering
, the
full structure and context are important, so stop words should
not be removed. This is also the case for
named entity recognition
, since named entities can contain
stop words themselves.
The Dutch spaCy model contains a list of stop words in the Dutch language.
['bijvoorbeeld', 'ikzelf', 'anderzijds', 'toch', 'jouwe', 'omtrent', 'geleden', 'een', 'met', 'voorts', 'pas', 'zal', 'meer', 'maar', 'wier', 'hen', 'hare', 'vervolgens', 'klaar', 'worden']
We proceed to remove it:
PYTHON
# remove stopwords
tokens_no_stopwords = tokens_no_punct
for stopword in stopwords:
tokens_no_stopwords = [token for token in tokens_no_stopwords if token != stopword]
print(tokens_no_stopwords[:20])
['mens', 'maan', 'eagle', 'geland', 'reisduur', '102', 'uur', 'uitstappen', '20', 'iuli', '21.17', 'uur', '45', 'min.', '40', 'sec.', 'vijf', 'uur', 'landing', 'armstrong']
Visualise tokens into a word cloud
PYTHON
from wordcloud import WordCloud
wordcloud = WordCloud().generate(' '.join(tokens_no_stopwords))
plt.imshow(wordcloud, interpolation='bilinear')
plt.axis("off")
plt.show()
Key Points
- Preprocessing involves a number of steps that one can apply to their text to prepare it for further processing.
- Preprocessing is important because it can improve your results
- You do not always need to do all preprocessing steps. It depends on the task at hand which preprocessing steps are important.
Tracing semantic shifts with word embeddings
Now we will train a model to to detect how the meaning of
ijzeren
, televisie
and mobiel
have shifted over the years, from the 50s to the 80s. This model will
return us distributional word representations
, also known
as embeddings
.
A number of publications (e.g., Turney et al., 2010; Baroni et al., 2014) have showed that embeddings provide an efficient way to track how meanings of words change across years. Let’s see what are those and how they manage to do that.
What are word embeddings?
A Word Embedding is a word representation type that maps words in a numerical manner (i.e., into vectors) in a multidimensional space, capturing their meaning based on characteristics or context. Since similar words occur in similar contexts, or have same characteristics, the system naturally learns to assign similar vectors to similar words.
Let’s illustrate this concept using animals. This example will show us an intuitive way of representing things into vectors.
Suppose we want to represent a cat
using measurable
characteristics:
- Furriness: Let’s assign a score of 70 to a cat
- Number of legs: A cat has 4 legs
So the vector representation of a cat becomes:
[70 (furriness), 4 (legs)]
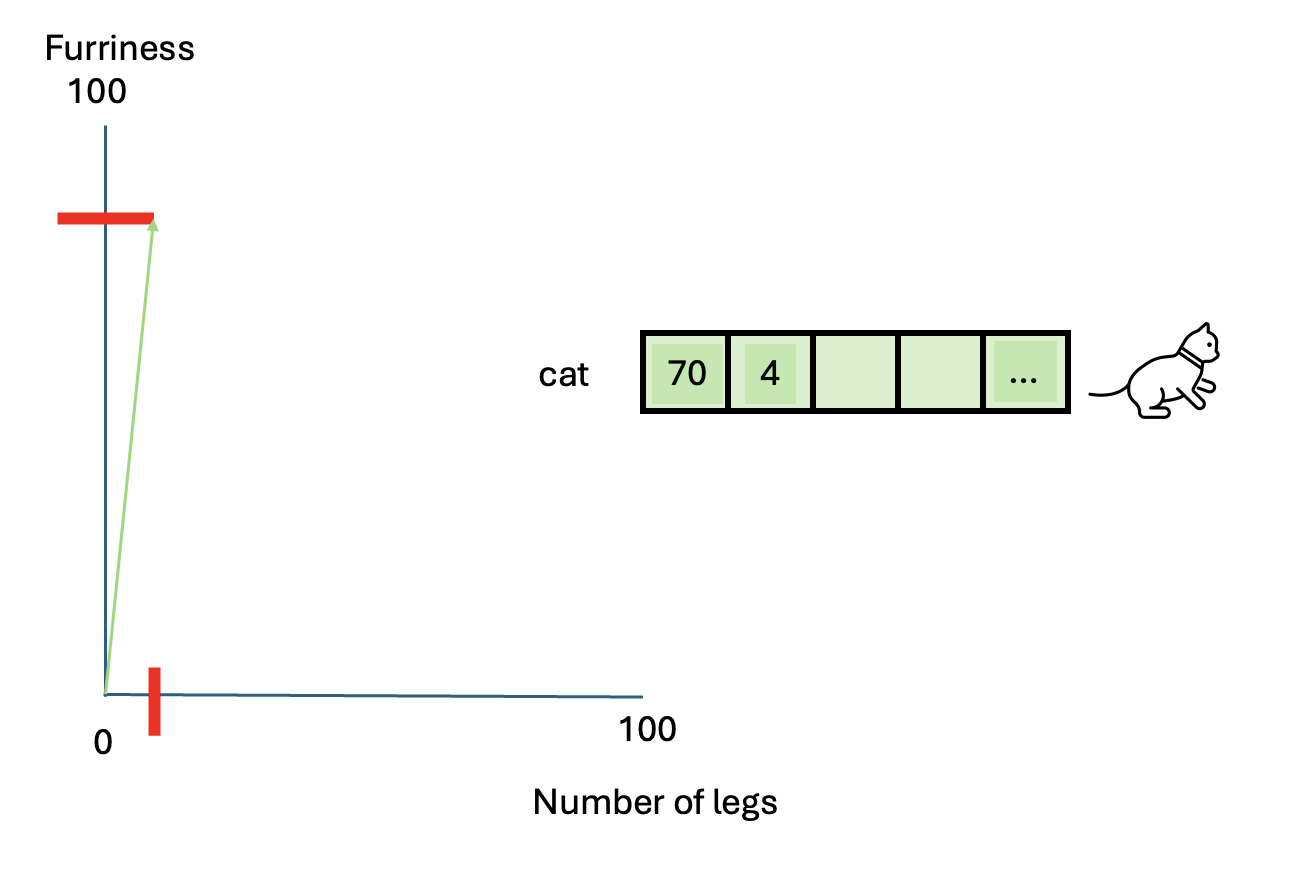
This vector doesn’t fully describe a cat but provides a basis for comparison with other animals.
Let’s add vectors for a dog and a caterpillar:
- Dog: [56, 4]
- Caterpillar: [70, 100]
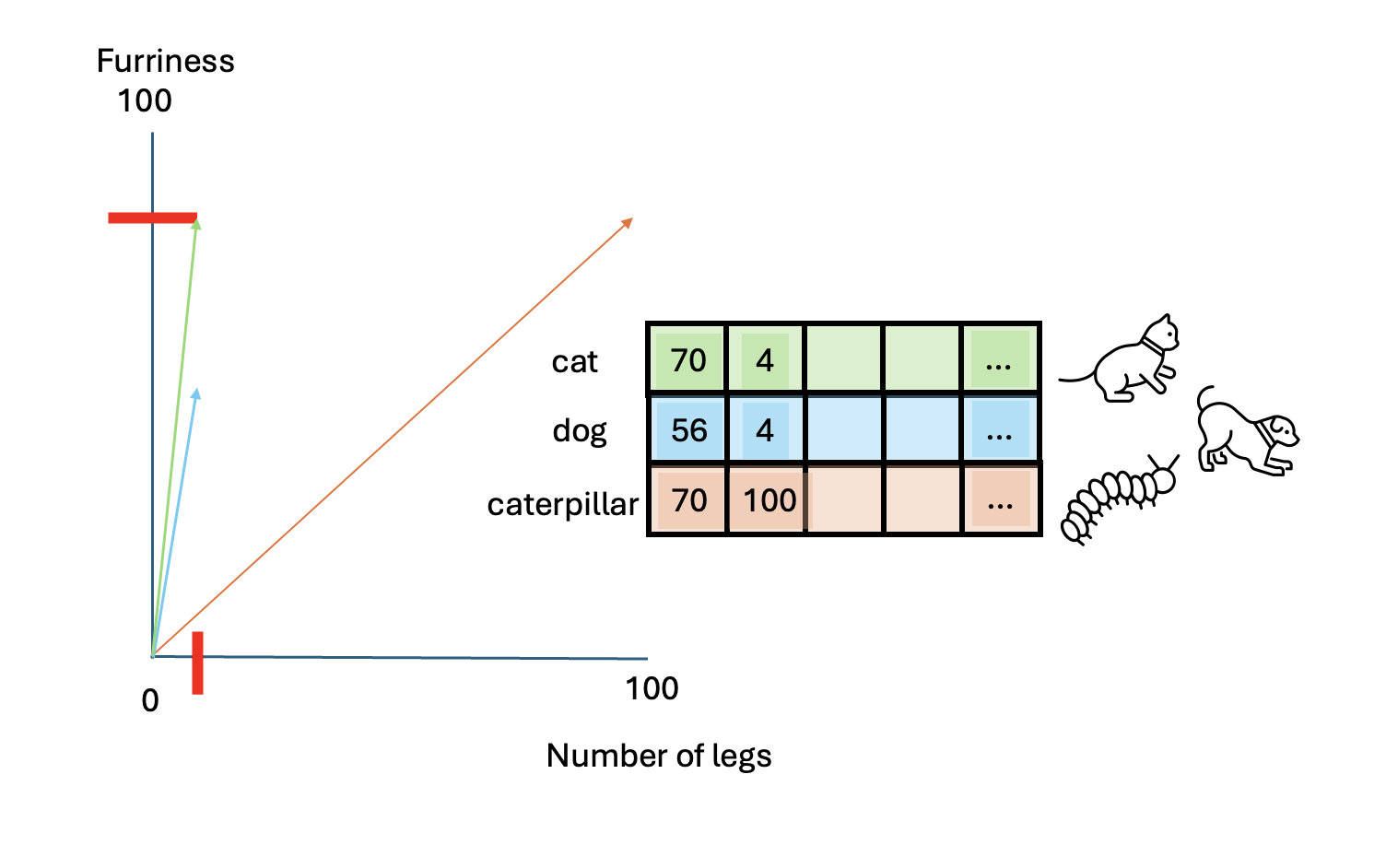
To determine which animal is more similar to a cat, we use
cosine similarity
, which measures the cosine of the angle
between two vectors.
Callout
cosine
similarity ranges between [-1
and 1
]. It
is the cosine of the angle between two vectors, divided by the product
of their length. It is a useful metric to measure how similar two
vectors are likely to be.
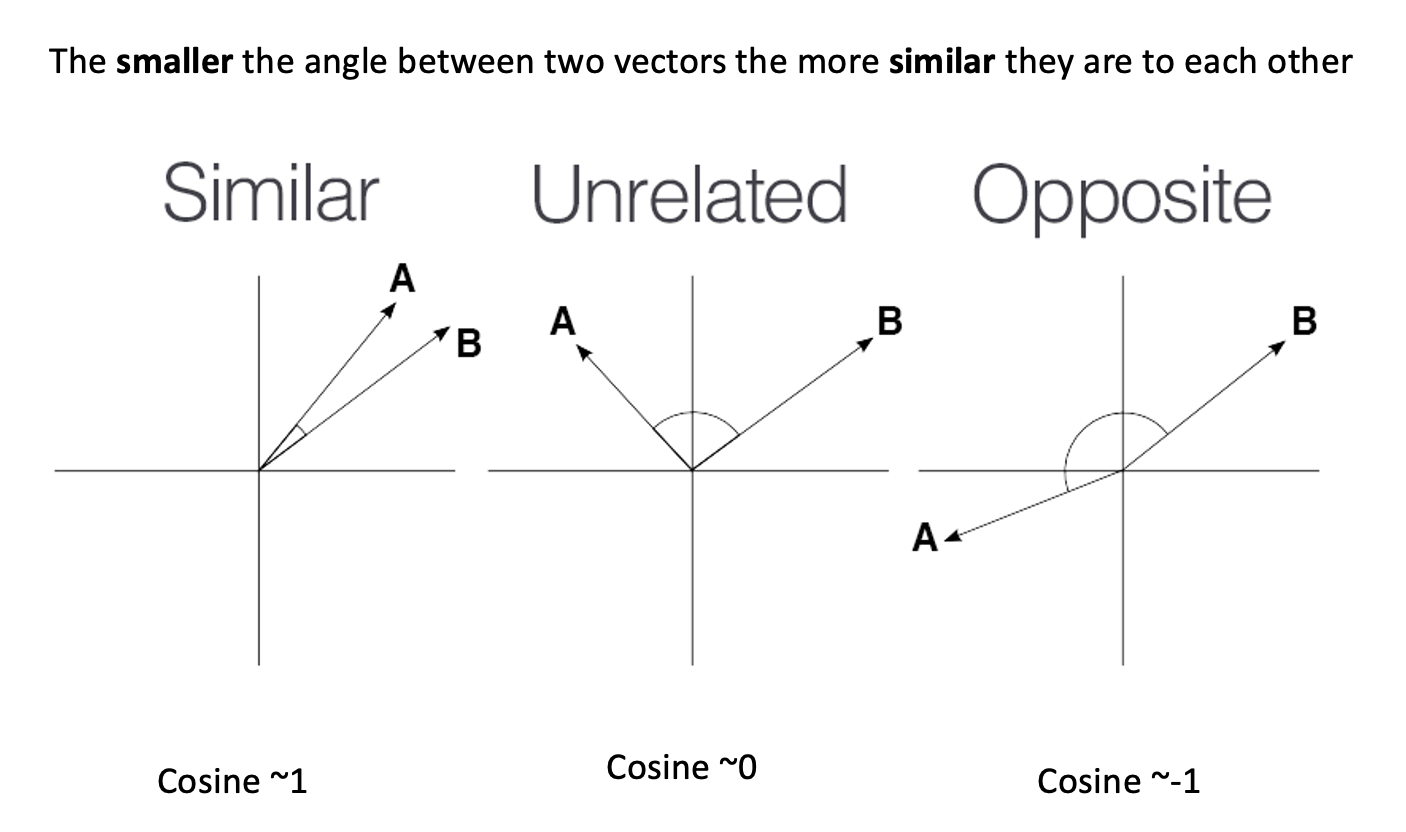
PYTHON
from sklearn.metrics.pairwise import cosine_similarity
similarity_cat_dog = cosine_similarity(cat, dog)[0][0]
similarity_cat_caterpillar = cosine_similarity(cat, caterpillar)[0][0]
print(f"Cosine similarity between cat and dog: {similarity_cat_dog}")
print(f"Cosine similarity between cat and caterpillar: {similarity_cat_caterpillar}")
PYTHON
Cosine similarity between cat and dog: 0.9998987965747193
Cosine similarity between cat and caterpillar: 0.6192653797321375
The higher similarity score between the cat and the dog indicates they are more similar based on these characteristics. Adding more characteristics can enrich our vectors, detecting more semantic nuances.
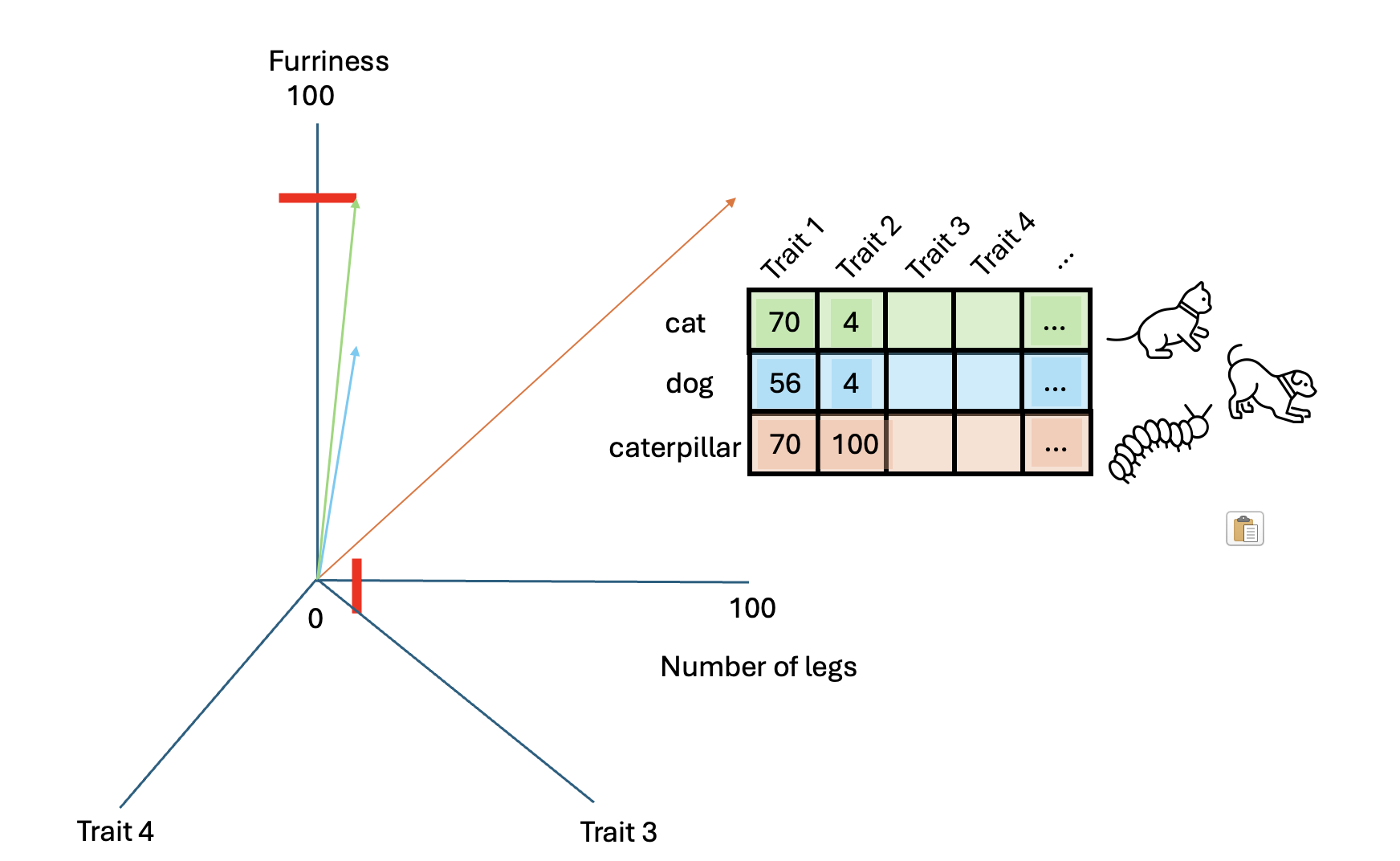
Challenge
- Add one of two other dimensions. What characteristics could they map?
- Add another animal and map their dimensions
- Compute again the cosine similarity among those animals and find the couple that is the least similar and the most similar
- Add one of two other dimensions
We could add the dimension of “velocity” or “speed” that goes from 0 to 100 meters/second.
- Caterpillar: 0.001 m/s
- Cat: 1.5 m/s
- Dog: 2.5 m/s
(just as an example, actual speeds may vary)
PYTHON
cat = np.asarray([[70, 4, 1.5]])
dog = np.asarray([[56, 4, 2.5]])
caterpillar = np.asarray([[70, 100, .001]])
Another dimension could be weight in Kg:
- Caterpillar: .05 Kg
- Cat: 4 Kg
- Dog: 15 Kg
(just as an example, actual weight may vary)
PYTHON
cat = np.asarray([[70, 4, 1.5, 4]])
dog = np.asarray([[56, 4, 2.5, 15]])
caterpillar = np.asarray([[70, 100, .001, .05]])
Then the cosine similarity would be:
Output:
- Add another animal and map their dimensions
Another animal that we could add is the Tarantula!
PYTHON
cat = np.asarray([[70, 4, 1.5, 4]])
dog = np.asarray([[56, 4, 2.5, 15]])
caterpillar = np.asarray([[70, 100, .001, .05]])
tarantula = np.asarray([[80, 6, .1, .3]])
- Compute again the cosine similarity among those animals - find out the most and least similar couple
Given the values above, the least similar couple is the dog and the
caterpillar, whose cosine similarity is
array([[0.60855407]])
.
The most similar couple is the cat and the tarantula:
array([[0.99822302]])
By representing words as vectors with multiple dimensions, we capture more nuances of their meanings or characteristics.
Key Points
- We can represent text as vectors of numbers (which makes it interpretable for machines)
- The most efficient and useful way is to use word embeddings
- We can easily compute how words are similar to each other with the cosine similarity
When semantic change occurs, words in their context also change. We can trace how a word evolves semantically over time through comparison of that word with other similar words. The idea is that the most similar words are not always fixed in each different year, if a word acquires a new meaning.
You shall know a word by the company it keeps - J. R. Firth, 1957
A word which holds the same meaning across time has stable contexts and similar words
A word that instead shifts meaning will be reflected by different contexts and words
So the changes of most similar words reflect the semantic change.
4. Train the Word2Vec model
Now we will train a two-layer neural network to transform our tokens
into word embeddings. We will be using the library gensim
and the model we will be using is called Word2Vec
,
developed by Tomas Mikolov et al. in 2013.
Import the necessary libraries:
PYTHON
import gensim
from gensim.models import Word2Vec
# import logging to monitor training
import logging
# set up logging
logging.basicConfig(format='%(asctime)s : %(levelname)s : %(message)s', level=logging.INFO)
There are two main architectures for training Word2Vec:
- Continuous Bag-of-Words (CBOW): Predicts a target word based on its surrounding context words.
- Continuous Skip-Gram: Predicts surrounding context words given a target word.
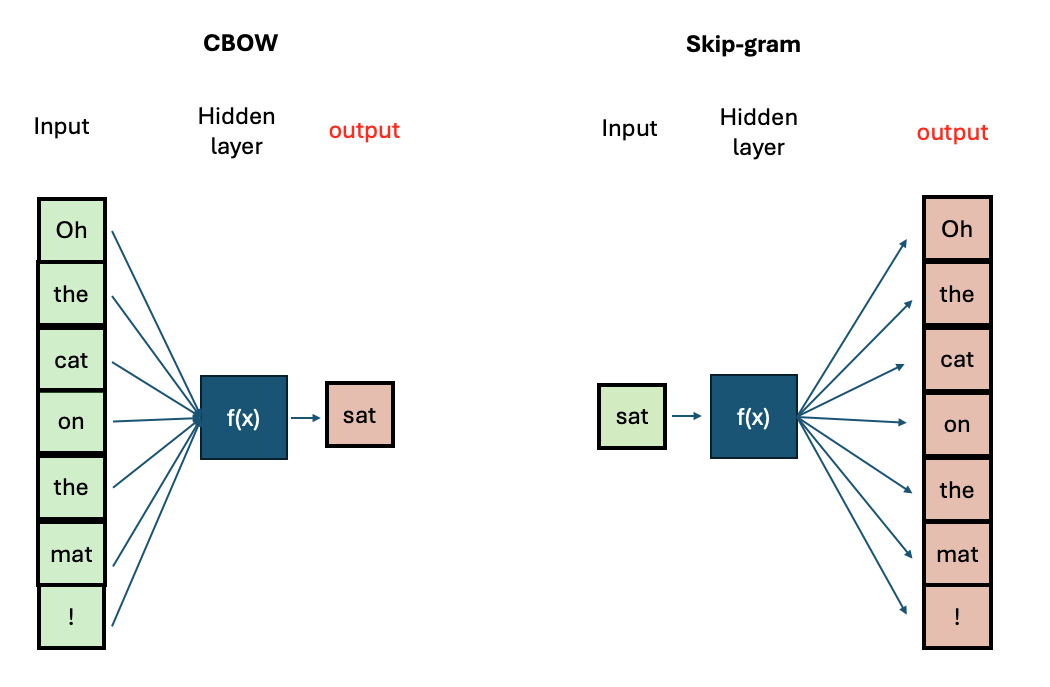
Callout
CBOW is faster to train, while Skip-Gram is more effective for infrequent words. Increasing context size improves embeddings but increases training time.
We will be using CBOW. We are interested in having vectors with 300 dimensions and a context size of 5 surrounding words. We include all words present in the corpora, regardless of their frequency of occurrence and use 4 CPU cores for training. All these specifics are translated in only one line of code.
Let’s train our model then:
PYTHON
model = Word2Vec([tokens_no_stopwords], vector_size=300, window=5, min_count=1, workers=4, sg=0)
We can inspect already what’s the output of this training, by checking the top 5 most similar words to “maan” (moon):
[('plek', 0.48467501997947693), ('ouders', 0.46935707330703735), ('supe|', 0.3929591178894043), ('rotterdam', 0.37788015604019165), ('verkeerden', 0.33672046661376953)]
We have trained our model on one page only of the newspaper and the
training was very quick. However, to approach our problem it’s best to
train our model on the entire dataset. We dont’ have the resources for
doing that on our local laptop, but luckily for us, Wevers, M (2019) did that
already for us and released it publicly. Let’s download this dataset on
our laptop and let’s save them in a folder called w2v
.
5. Load the embeddings and inspect them
We proceed to load our models. We will load all pre-trained model
files from the journal telegraaf
into a list. The library
gensim
contains a method called KeyedVectors
which allows us to load them.
PYTHON
from gensim.models import KeyedVectors
import os
filenames_by_decade = [
'telegraaf_1950_1959.w2v',
'telegraaf_1960_1969.w2v',
'telegraaf_1970_1979.w2v',
'telegraaf_1980_1989.w2v'
]
def load_w2v_models(filenames, folder_path):
loaded_models_by_decade = []
for file in filenames:
path = os.path.join(folder_path, file)
print(f'loading model {path}')
model = KeyedVectors.load_word2vec_format(path, binary=True)
loaded_models_by_decade.append(model)
return loaded_models_by_decade
# run the function to load the models
telegraaf_models = load_w2v_models(filenames_by_decade, folder_path)
We should see the following prints:
PYTHON
loading model data/w2v/telegraaf_1950_1959.w2v
loading model data/w2v/telegraaf_1960_1969.w2v
loading model data/w2v/telegraaf_1970_1979.w2v
loading model data/w2v/telegraaf_1980_1989.w2v
This means that we have loaded the models correctly.
Now let’s proceed to inspect the top 10 neighbours of the word
mobiel
(to start with) across the decades:
PYTHON
decades = ['50s', '60s', '70s', '80s']
for decade in range(len(decades)):
top_neighbours = telegraaf_models[decade].most_similar('mobiel', topn=10)
print(f'decade: {decades[decade]}')
for neighbour in top_neighbours:
print(neighbour[0])
print('\n')
This is what we should see:
PYTHON
decade: 50s
locatie
stationeren
landingsvaartuigen
stationering
toegangspoort
legeren
mustangs
imperialistisch
landmijnen
oprukt
decade: 60s
maagpijnen
ureum
bagagewagen
waterpartij
stillere
boormachine
achterportier
doorsnijden
stralingsgevaar
opgepast
decade: 70s
beweeglijk
beweeglijke
kunstrubriek
kleutert
klankbeeld
radiojournaal
knipperlicht
meisjeskoor
kinderkoor
volksverhalen
decade: 80s
communicatieapparatuur
parkeerterreinen
sonar
alarmsysteem
gasinstallaties
lichtnet
elektromotor
inentingen
sensoren
hulpbehoevenden
Let’s inspect together these results:
In the 50s, the neighbouring words predominantly point towards military and geopolitical terms (stationeren, landingsvaartuigen, stationering, legeren, and landmijnen). The presence of the word imperialistisch also suggests discussions about imperialism, possibly reflecting post WWII tensions (in the 50s Europe was entering Cold War period).
In the 60s, the term is associated to meanings related to health, safety and mechanical terms. Stralingsgevaar and opgepast suggest concerns about radiation and the need for caution, possibly reflecting the nuclear anxieties of the era.
In the 70s the word is associated to technological advancement and culture. While finally in the 80s we see a list of words that have solid grounds in technological and infrastracture terms. Words like communicatieapparatuur, sonar, alarmsysteem, elektromotor, and sensoren signals the push that technology has had in this period, with the advent of mobile phones (communicatieapparatuur).
All in all, the word’s meaning evolved from being a means of transport to a modern technology tool employed in urban infrastructure, societal well-being and communication.
Challenge
Reproduce the steps above for the other words: televisie
and ijzeren
. What do you expect from their historical
semantic evolution? Television was already present in the 50s, although
the technology around it has evolved up to the 1989. And what about the
term iron
? When do you expect this term to acquire a
meaning related to the Cold War e.g. Iron Curtain?
Content from Episode 2: BERT and Transformers
Last updated on 2025-01-09 | Edit this page
Overview
Questions
- What are Transformers?
- What is BERT and how does it work?
- How can I use BERT as a text classifier?
- How should I evaluate my classifiers?
Objectives
After following this lesson, learners will be able to:
- Understand how a Transformer works and recognize their different use cases.
- Use pre-trained transformers language models (e.g. BERT) to classify texts.
- Use a pre-trained transformer Named Entity Recognizer.
- Understand assumptions and basic evaluation for NLP outputs.
In the previous lesson we learned how Word2Vec can be used to represent words as vectors. Having these representations allows us to apply operations directly on the vectors that have numerical properties that can be mapped to some syntactic and semantic properties of words; such as the cases of analogies or finding synonyms. Once we transform words into vectors, these can also be used as features for classifiers that can be trained predict any supervised NLP task.
The main drawback of Word2Vec is that each word is represented in isolation, and unfortunately that is not how language works. Words get their meanings based on the specific context in which they are used (take for example polysemy, the cases where the same word can have very different meanings depending on the context); therefore, we would like to have richer vector representations of words that also integrate context into account in order to obtain more powerful representations.
In 2019, the BERT language model was introduced using a novel architecture called Transformer (2017), which allowed precisely to integrate words’ context into representations. To understand BERT, we will first look at what a transformer is and we will then directly use some code to make use of BERT.
Transformers
Every text can be seen as a sequence of sentences and likewise each sentence can be seen as a sequence of tokens (we use the term token instead of word because it is more general: tokens can be words, punctuation symbols, numbers, or even sub-words). Traditionally Recurrent Neural Networks (RNNs; and later their fancy version, LSTMs) were used to tackle token and sentence classification problems to account for the interdependencies inherent to sequences of symbols (i.e. sentences). RNNs were in theory powerful enough to capture these dependencies, something that is very valuable when dealing with language, but in practice they were resource consuming (both in training time and computational resources) and also the longer the sequences got, the harder it was to capture long-distance dependencies succesfully.
The Transformer is a neural network architecture proposed by Google researchers in 2017 to address these and other limitations of RNNs and LSTMs. In their paper, Attention is all you Need, they tackled specifically the problem of Machine Translation (MT), which in NLP terms is stated as: how to generate a sentence (sequence of words) in target language B given a sentence in source language A? In order to translate, first one neural network needs to encode the meaning of the source language A into vector representations, and then a second neural network needs to decode that representation into tokens that are understandable in language B. Therefore translation is modeling language B conditioned on what language A originally said.
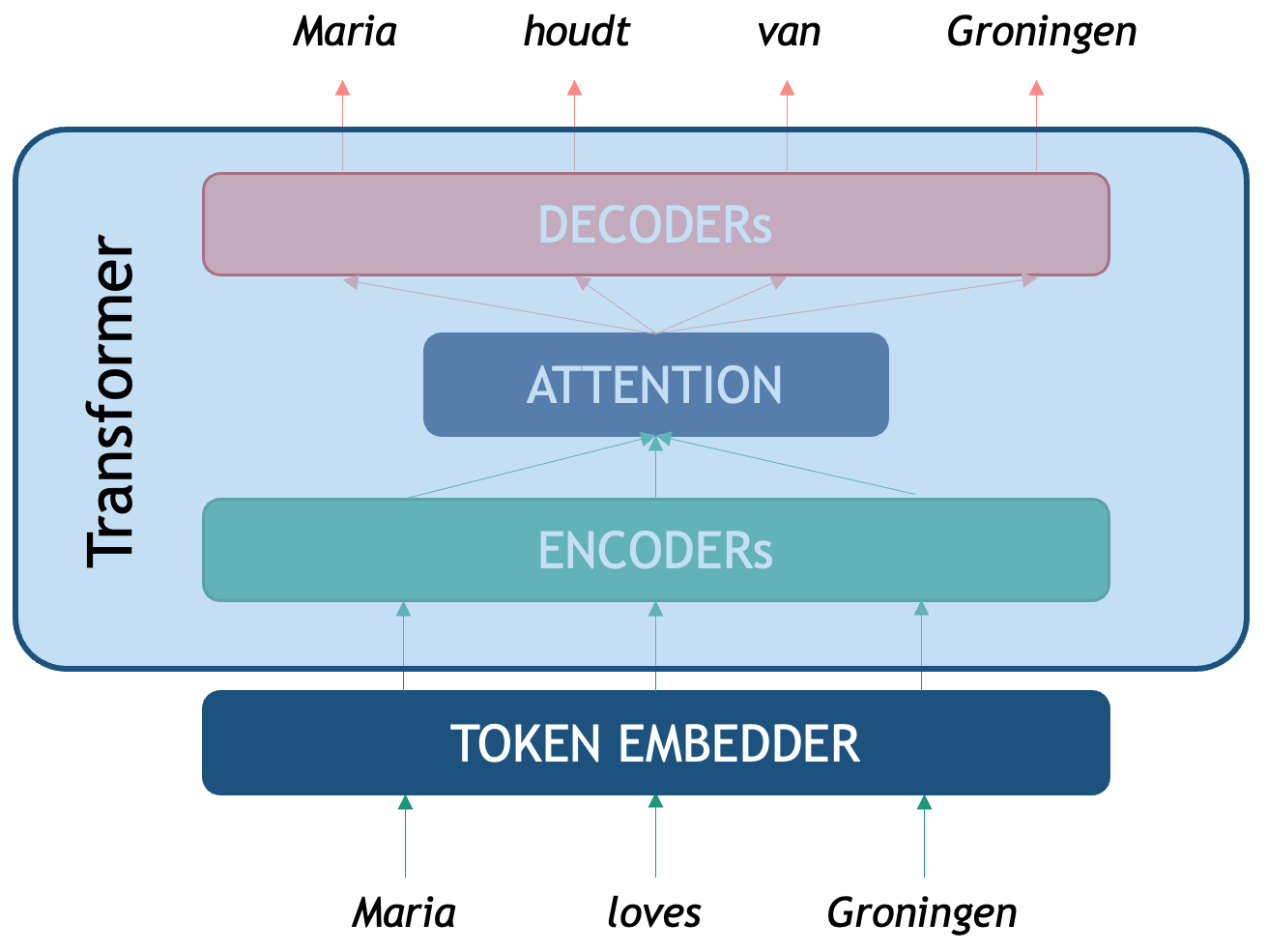
As seen in the picture, the original Transformer is an Encoder-Decoder network that tackles translation. We first need a token embedder which converts the string of words into a sequence of vectors that the Transformer network can process. The first component, the Encoder, is optimized for creating rich representations of the source sequence (in this case an English sentence) while the second one, the Decoder is a generative network that is conditioned on the encoded representation and, with the help of the attention mechanism, generates the most likely token in the target sequence (in this case Dutch words) based on both the tokens generated so far and the full initial English context.
Next, we will see how BERT exploits the idea of a Transformer Encoder to generate powerful word representations.
BERT
BERT is an acronym that stands for Bidirectional Encoder Representations from Transformers. The name describes it all: the idea is to use the power of the Encoder component of the Transformer architecture to create powerful token representations that preserve the contextual meaning of the whole input segment. The BERT vector representations of each token take into account both the left context (what comes before the word) and the right context (what comes after the word). Another advantage of the transformer Encoder is that it is parallelizable, which made it posible for the first time to train these networks on millions of datapoints, dramatically improving model generalization.
Pretraining BERT
To obtain the BERT vector representations the Encoder is pre-trained with two different tasks: - Masked Language Model: for each sentence, mask one token at a time and predict which token is missing based on the context from both sides. A training input example would be “Maria [MASK] Groningen” and the model should predict the word “loves”. - Next Sentence Prediction: the Encoder gets a linear binary classifier on top, which is trained to decide for each pair of sequences A and B, if sequence A precedes sequence B in a text. For the sentence pair: “Maria loves Groningen.” and “This is a city in the Netherlands.” the output of the classifier is “True” and for the pair “Maria loves Groningen.” and “It was a tasty cake.” the output should be “false” as there is no obvious continuation between the two sentences.
Already the second pre-training task gives us an idea of the power of BERT: after it has been pretrained on hundreds of thousands of texts, one can plug-in a classifier on top and re-use the linguistic knowledge previously acquired to fine-tune it for a specific task, without needing to learn the weights of the whole network from scratch all over again. In the next sections we will describe the components of BERT and show how to use it. This model and hundreds of related transformer-based pre-trained encoders can also be found on Hugging Face.
BERT Architecture
Now that we used the BERT language model component we can dive into the architecture of BERT to understand it better.
As in any basic NLP pipeline, the first step is to pre-process the raw text so it is ready to be fed into the Transformer. Tokenization in BERT does not happen at the word-level but rather splits texts into what they call WordPieces (the reason for this decision is complex, but in short, researchers found that splitting human words into subtokens exploits better the character sub-sequences inside words and helps the model converge faster). A word then sometimes is decomposed into one or several (sub) tokens.
- Tokenizer: splits text into tokens that the model recognizes
- Embedder: converts each token into a fixed-sized vector that represents it. These vectors are the actual input for the Encoder.
- Encoder several neural layers that model the token-level interactions of the input sequence to enhance meaning representation. The output of the encoder is a set of Hidden layers, the vector representation of the ingested sequence.
- Output Layer: the final encoder layer (which we depict as a sequence H’s in the figure) contains arguably the best token-level representations that encode syntactic and semantic properties of each token, but this time each vector is already contextualized with the specific sequence.
- OPTIONAL Classifier Layer: an additional classifier can be connected on top of the BERT token vectors which are used as features for performing a downstream task. This can be used to classify at the text level, for example sentiment analysis of a sentence, or at the token-level, for example Named Entity Recognition.
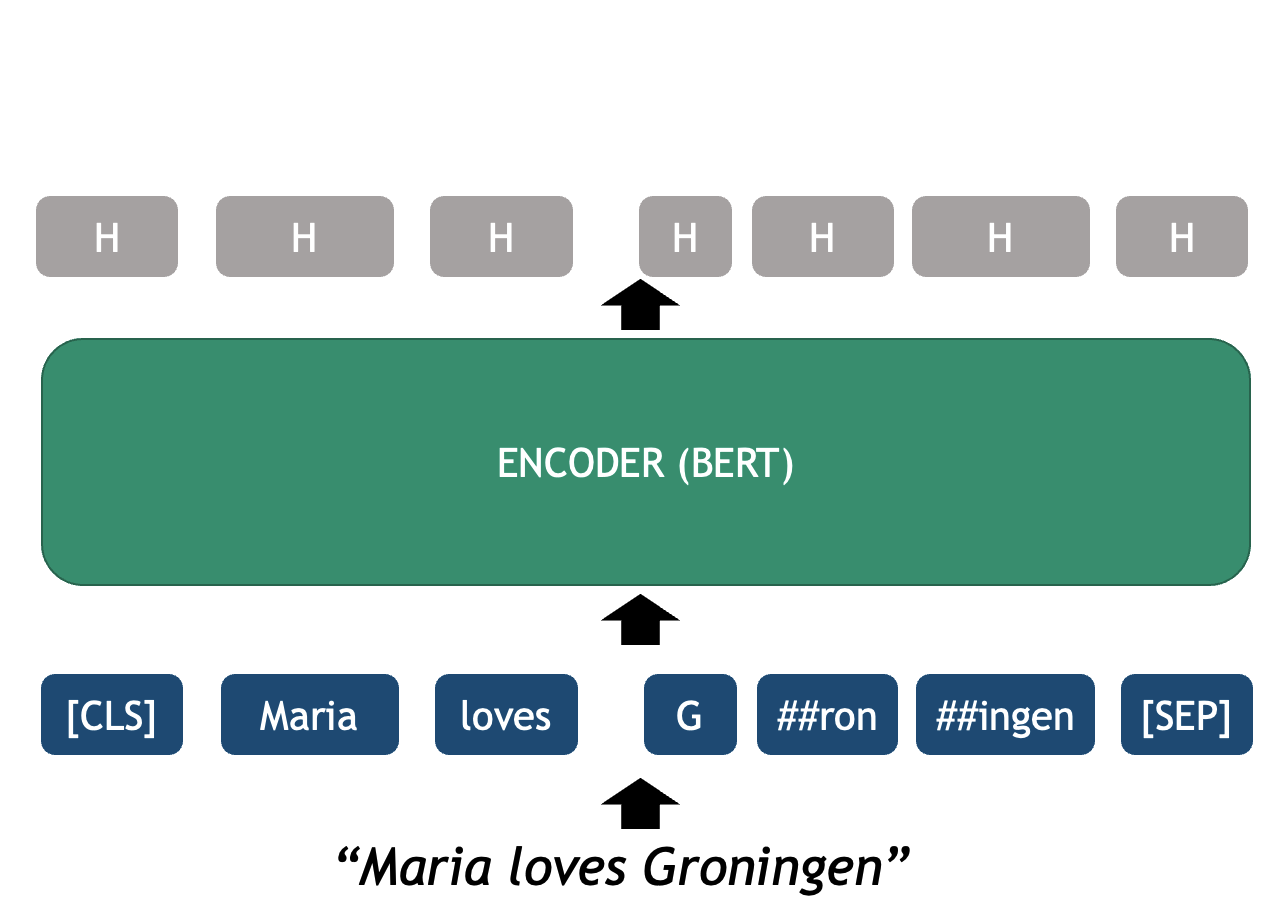
BERT Code
Let’s see how these components can be manipulated with code. For this we will be using the HugingFace’s transformers python library. We can install it with:
The first two main components we need to initialize are the model and tokenizer. The HuggingFace hub contains thousands of models based on a Transformer architecture for dozens of tasks, data domains and also hundreds of languages. Here we will explore the vanilla English BERT which was how everything started. We can initialize this model with the next lines:
BERT Tokenizer
We start with a string of text as written in any blog, book,
newspaper etcetera. The tokenizer
object is responsible of
splitting the string into recognizable tokens for the model and
embedding the tokens into their vector representations
PYTHON
text = "Maria loves Groningen"
encoded_input = tokenizer(text, return_tensors='pt')
print(encoded_input)
The print shows the encoded_input
object returned by the
tokenizer, with its attributes and values. The input_ids
are the most important output for now, as these are the token IDs
recognized by BERT
{
'input_ids': tensor([[ 101, 3406, 7871, 144, 3484, 15016, 102]]),
'token_type_ids': tensor([[0, 0, 0, 0, 0, 0, 0]]),
'attention_mask': tensor([[1, 1, 1, 1, 1, 1, 1]])
}
NOTE: the printing function shows transformers objects as dictionaries; however, to access the attributes, you must use the python object syntax, such as in the following example:
Output:
torch.Size([1, 7])
The output is a 2-dimensional tensor where the first dimention contains 1 element (this dimension represents the batch size), and the second dimension contains 7 elements which are equivalent to the 7 tokens that BERT generated with our string input.
Callout
You noticed in the previous outputs the tensor()
and
torch()
wrappers around the arrays of integers. This is
showing that the transformers
library uses
pytorch
underneath, one of the most popular libraries for
Deep Learning in Python. Pytorch’s basic unit is the Tensor.
A tensor is a generalization of a multidimentional array of data. By convention, a vector is a 1-dimensional sequence of scalar numbers (or a 1-dim tensor), a matrix is a 2-dimensional sequence (2-dim tensor) and for N-dimensions where N > 2 we use the concept of tensor.
In order to see what these Token IDs represent, we can translate them into human readable strings. This includes converting the tensors into numpy arrays and converting each ID into its string representation:
PYTHON
token_ids = list(encoded_input.input_ids[0].detach().numpy())
string_tokens = tokenizer.convert_ids_to_tokens(token_ids)
print("IDs:", token_ids)
print("TOKENS:", string_tokens)
IDs: [101, 3406, 7871, 144, 3484, 15016, 102]
TOKENS: ['[CLS]', 'Maria', 'loves', 'G', '##ron', '##ingen', '[SEP]']
These show us the WordPieces that the BERT Encoder will receive and process. We will look more in detail into the tokenization and special tokens later. For now, you just need to know that the encoder uses this token IDs to retrieve the corresponding embedding vector from its vocabulary, the string representations are just for the human reader.
BERT Output Object
To give a forward pass of the Encoder and obtain the vector
representations, we pass the encoded_input
object generated
by the tokenizer.
The output
variable in this case stores an ModelOutput
object, which contains a handful of values:
BaseModelOutputWithPoolingAndCrossAttentions(
last_hidden_state=tensor([[
[6.3959e-02, -4.8466e-03, -8.4682e-02, ..., -2.8042e-02, 4.3824e-01, 2.0693e-02],
[-3.7276e-04, -2.0076e-01, 2.5096e-01, ..., 9.9699e-01, -5.4226e-01, 1.7926e-01],
...
[ 7.1929e-01, -1.1457e-01, 1.4804e-01, ..., 5.3051e-01, 7.4839e-01, 7.8224e-02]
]]),
pooler_output=tensor([[-0.6889, 0.4869, 0.9998, -0.9888, 0.9296, 0.8637, ..., 1.0000, -0.7488, 0.9860]]),
hidden_states=None,
past_key_values=None,
attentions=None,
cross_attentions=None
)
We must focus for now on the last_hidden_state
field,
which contains the last layer vector of weights for each token, arguably
the best contextualized representation of the token.
torch.Size([1, 7, 768])
When we print the shape of this field, we obtain again a Pytorch
Tensor: torch.Size([1, 7, 768])
. This time, the first
dimension is the batch size, the second is the number of tokens (we have
7 tokens for this example as seen before), and the third, the
dimensionality of the vectors. In the case of BERT-base each token
vector always has a shape of 768. As opposed to the previous tensor,
each of the 7 tokens are not just one integer anymore, but a whole
vector of weights, hence the 3-dimensionality of the tensor.
Callout
When running examples in a BERT pre-trained model, it is advisable to
wrap your code inside a torch.no_grad():
context. This is
linked to the fact that BERT is a Neural Network that has been trained
(and can be further finetuned) with the Backpropagation algorithm.
Essentially, this wrapper tells the model that we are not in training
mode, and we are not interested in updating the weights (as it
would happen when training any neural network), because the weights are
already optimal enough. By using this wrapper, we make the model more
efficient as it does not need to calculate the gradients for an eventual
backpropagation step, since we are only interested in what comes
out of the Encoder. So the previous code can be made more efficient
like this:
BERT as a Language Model
Now that we know how to embedd and run the model to obtain the
representations, we can test the code for our first NLP Task: Language
Modelling (LM). As mentioned before, the main pre-training task of BERT
is LM: calculating the probability of a word based on the known
neighboring words (yes, Word2Vec was also a kind of LM). Obtaining
training data for this task is very cheap, as all we need is millions of
sentences from existing texts, without any labels. In this setting, BERT
encodes a sequence of words, and predicts from a set of English tokens,
what is the most likely token that could be inserted in the
[MASK]
position
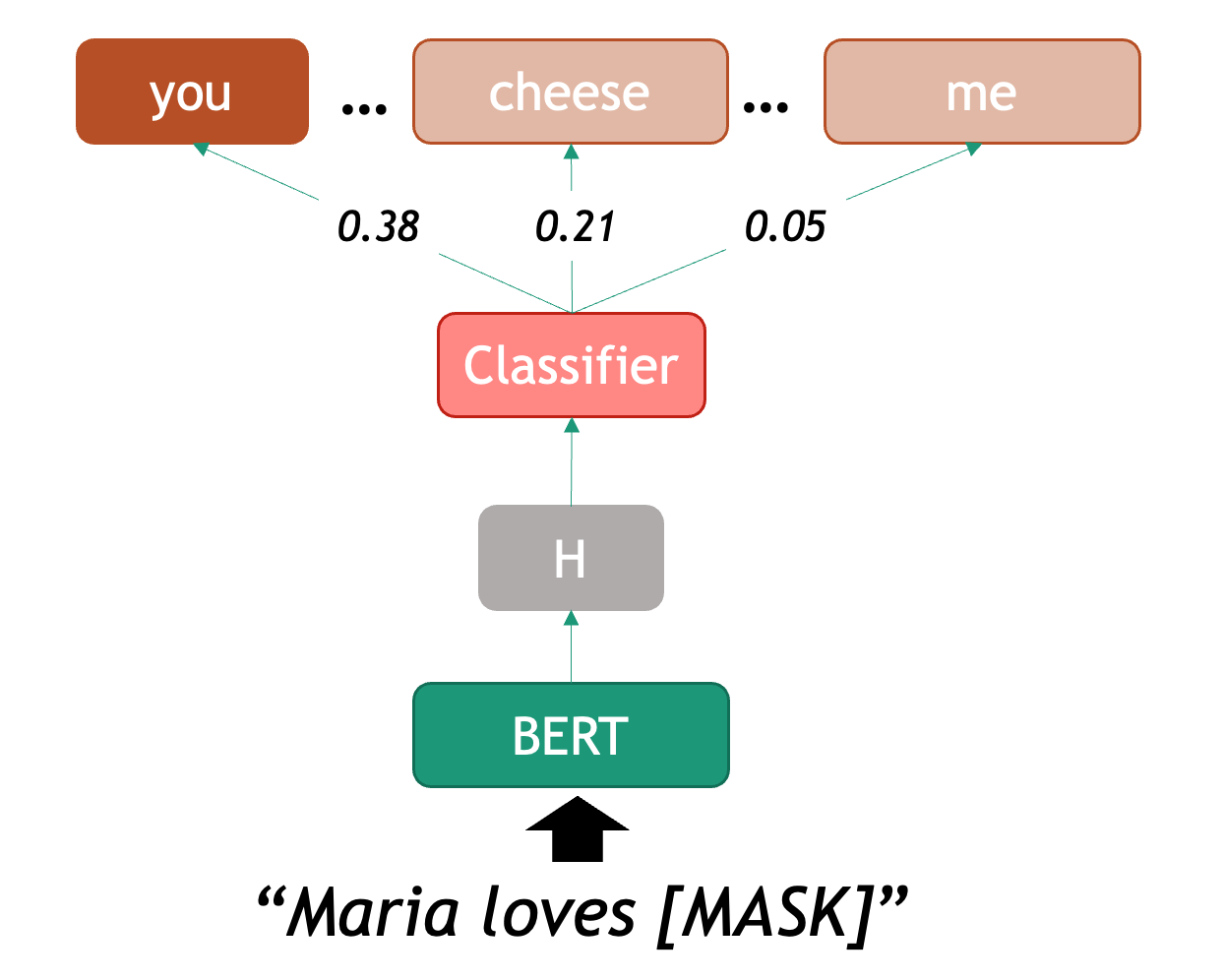
We can therefore start using BERT as a predictor for word completion,
and the word can be in any position inside the sentence. We will also
learn here how to use the pipeline
object, this is very
useful when we only want to use a pre-trained model for predictions (no
need to fine-tune). The pipeline
will internally initialize
both model and tokenizer for us. In this case again we use
bert-base-cased
, which refers to the vanilla BERT English
model. Once we declared a pipeline, we can feed it with sentences that
contain one masked token at a time (beware that BERT can only predict
one word at a time, since that was its training scheme). For
example:
PYTHON
from transformers import pipeline
def pretty_print_outputs(sentences, model_outputs):
for i, model_out in enumerate(model_outputs):
print("\n=====\t",sentences[i])
for label_scores in model_out:
print(label_scores)
nlp = pipeline(task="fill-mask", model="bert-base-cased", tokenizer="bert-base-cased")
sentences = ["Paris is the [MASK] of France", "I want to eat a cold [MASK] this afternoon", "Maria [MASK] Groningen"]
model_outputs = nlp(sentences, top_k=5)
pretty_print_outputs(sentences, model_outputs)
===== Paris is the [MASK] of France
{'score': 0.9807965755462646, 'token': 2364, 'token_str': 'capital', 'sequence': 'Paris is the capital of France'}
{'score': 0.004513159394264221, 'token': 6299, 'token_str': 'Capital', 'sequence': 'Paris is the Capital of France'}
{'score': 0.004281804896891117, 'token': 2057, 'token_str': 'center', 'sequence': 'Paris is the center of France'}
{'score': 0.002848200500011444, 'token': 2642, 'token_str': 'centre', 'sequence': 'Paris is the centre of France'}
{'score': 0.0022805952467024326, 'token': 1331, 'token_str': 'city', 'sequence': 'Paris is the city of France'}
===== I want to eat a cold [MASK] this afternoon
{'score': 0.19168031215667725, 'token': 13473, 'token_str': 'pizza', 'sequence': 'I want to eat a cold pizza this afternoon'}
{'score': 0.14800849556922913, 'token': 25138, 'token_str': 'turkey', 'sequence': 'I want to eat a cold turkey this afternoon'}
{'score': 0.14620967209339142, 'token': 14327, 'token_str': 'sandwich', 'sequence': 'I want to eat a cold sandwich this afternoon'}
{'score': 0.09997560828924179, 'token': 5953, 'token_str': 'lunch', 'sequence': 'I want to eat a cold lunch this afternoon'}
{'score': 0.06001955270767212, 'token': 4014, 'token_str': 'dinner', 'sequence': 'I want to eat a cold dinner this afternoon'}
===== Maria [MASK] Groningen
{'score': 0.24399833381175995, 'token': 117, 'token_str': ',', 'sequence': 'Maria, Groningen'}
{'score': 0.12300779670476913, 'token': 1104, 'token_str': 'of', 'sequence': 'Maria of Groningen'}
{'score': 0.11991506069898605, 'token': 1107, 'token_str': 'in', 'sequence': 'Maria in Groningen'}
{'score': 0.07722211629152298, 'token': 1306, 'token_str': '##m', 'sequence': 'Mariam Groningen'}
{'score': 0.0632941722869873, 'token': 118, 'token_str': '-', 'sequence': 'Maria - Groningen'}
When we call the nlp
pipeline, requesting to return the
top_k
most likely suggestions to complete the provided
sentences (in this case k=5
). The pipeline returns a list
of outputs as python dictionaries. Depending on the task, the fields of
the dictionary will differ. In this case, the fill-mask
task returns a score (between 0 and 1, the higher the score the more
likely the token is), a tokenId, and its corresponding string, as well
as the full “unmasked” sequence.
In the list of outputs we can observe: the first example shows correctly that the missing token in the first sentence is capital, the second example is a bit more ambiguous, but the model at least uses the context to correctly predict a series of items that can be eaten (unfortunately, none of its suggestions sound very tasty); finally, the third example gives almost no useful context so the model plays it safe and only suggests prepositions or punctuation. This already shows some of the weaknesses of the approach.
We will next see the case of combining BERT with a classifier on top.
BERT for Text Classification
The task of text classification is assigning a label to a whole
sequence of tokens, for example a sentence. With the parameter
task="text_classification"
the pipeline()
function will load the base model and automatically add a linear layer
with a softmax on top. This layer can be fine-tuned with our own labeled
data or we can also directly load the fully pre-trained text
classification models that are already available in HuggingFace.
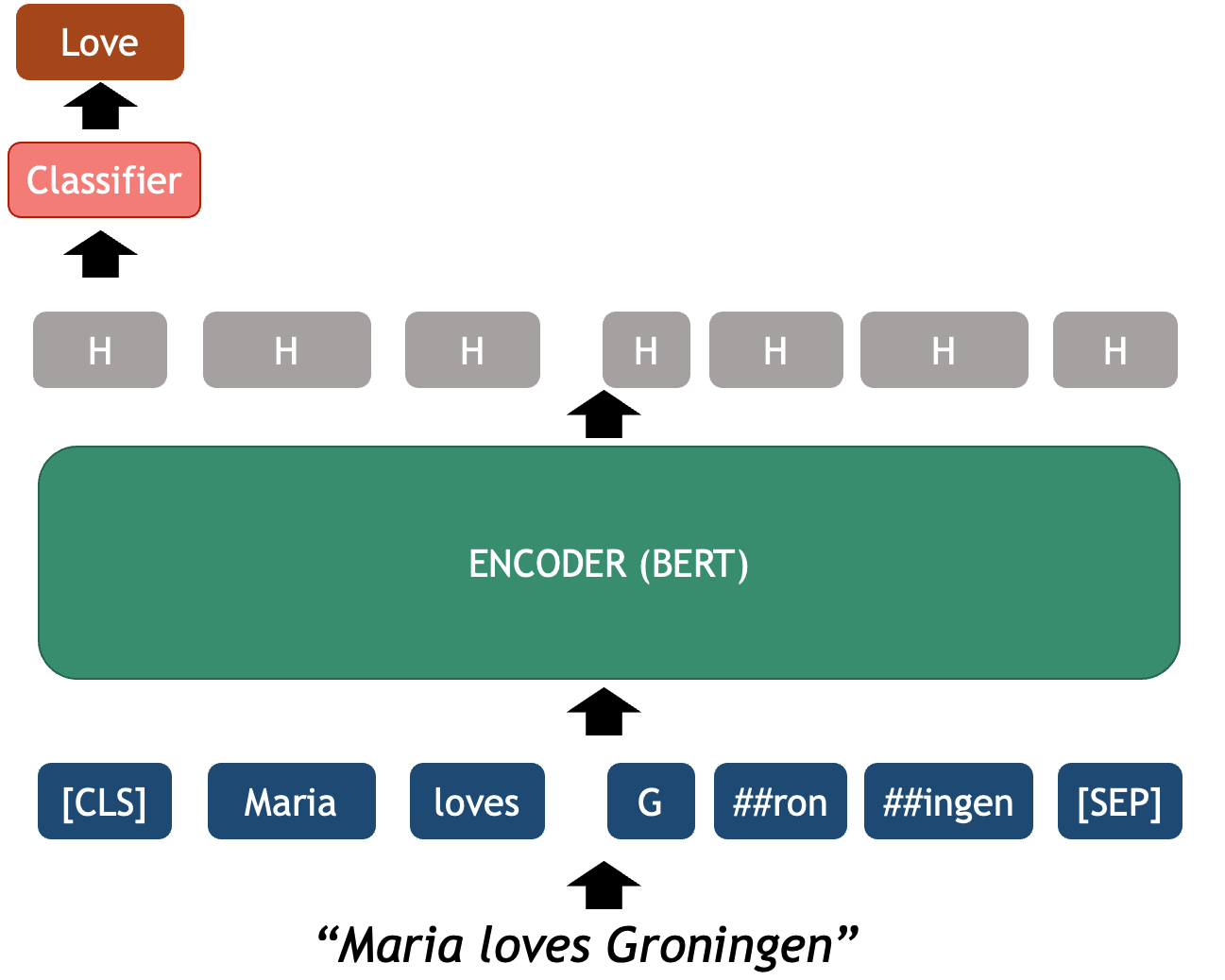
Let’s see the example of a ready pre-trained emotion classifier based
on RoBERTa
model. This model was fine-tuned in the Go
emotions dataset,
taken from English Reddit and labeled for 28 different emotions at the
sentence level. The fine-tuned model is called roberta-base-go_emotions.
This model takes a sentence as input and ouputs a probability
distribution over the 28 possible emotions that might be conveyed in the
text. For example:
PYTHON
classifier = pipeline(task="text-classification", model="SamLowe/roberta-base-go_emotions", top_k=3)
sentences = ["I am not having a great day", "This is a lovely and innocent sentence", "Maria loves Groningen"]
model_outputs = classifier(sentences)
pretty_print_outputs(sentences, model_outputs)
===== I am not having a great day
{'label': 'disappointment', 'score': 0.46669483184814453}
{'label': 'sadness', 'score': 0.39849498867988586}
{'label': 'annoyance', 'score': 0.06806594133377075}
===== This is a lovely and innocent sentence
{'label': 'admiration', 'score': 0.6457845568656921}
{'label': 'approval', 'score': 0.5112180113792419}
{'label': 'love', 'score': 0.09214121848344803}
===== Maria loves Groningen
{'label': 'love', 'score': 0.8922032117843628}
{'label': 'neutral', 'score': 0.10132959485054016}
{'label': 'approval', 'score': 0.02525361441075802}
This code outputs again a list of dictionaries with the
top-k
(k=3
) emotions that each of the two
sentences convey. In this case, the first sentence evokes (in order of
likelihood) dissapointment, sadness and
annoyance; whereas the second sentence evokes love,
neutral and approval. Note however that the likelihood
of each prediction decreases dramatically below the top choice, so
perhaps this specific classifier is only useful for the top emotion.
Callout
Finetunning BERT is very cheap, because we only need to train the classifier layer, a very small neural network, that can learn to choose between the classes (labels) for your custom classification problem, without needing a big amount of annotated data. This classifier is just a one-layer neural layer with a softmax that assigns a score that can be translated to the probability over a set of labels, given the input features provided by BERT, which encodes the meaning of the entire sequence in its hidden states.
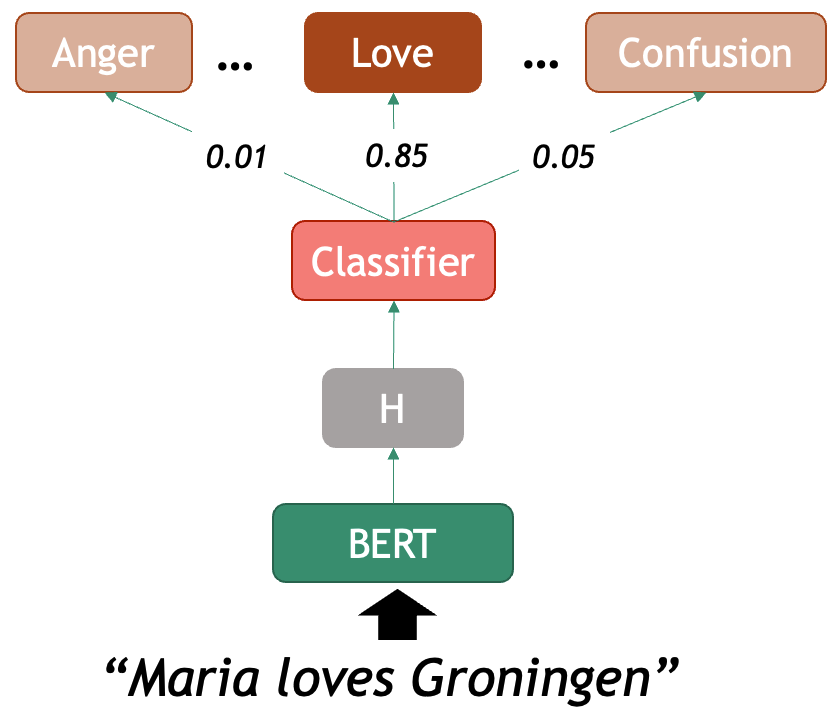
Understanding BERT Architecture
This will help to understand some of the strengths and weaknesses of using BERT-based classifiers.
Tokenizer and Embedder
Let’s revisit the tokenizer to better grasp how it is working. The tokenization step might seem trivial but in reality models’ tokenizers make a big difference in the final results of your classifiers, depending on the task you are trying to solve. Understanding the tokenizer of each model (as well as the model type!) can save us a lot of debugging when we work with our custom problem.
We will feed again a sentence into the tokenizer to observe how it outputs a sequence of vectors (also called a tensor: by convention, a vector is a sequence of scalar numbers, a matrix is a 2-dimensional sequence and a tensor is a N-dimensional sequence of numbers), each one of them representing a wordPiece:
PYTHON
# Feed text into the tokenizer
text = "Maria's passion for music is clearly heard in every note and every enchanting melody."
encoded_input = tokenizer(text, return_tensors='pt')
token_ids = list(encoded_input.input_ids[0].detach().numpy())
string_tokens = tokenizer.convert_ids_to_tokens(token_ids)
print(string_tokens)
['[CLS]', 'Maria', "'", 's', 'passion', 'for', 'music', 'is', 'clearly', 'heard', 'in', 'every', 'note', 'and', 'every', 'en', '##chan', '##ting', 'melody', '.', '[SEP]']
This shows a list of token IDs, as we saw with our first example, this time the list consists of 21 BERT tokens.
When inspecting the string tokens, we see that most “words” were
converted into a single token, however enchanting was splitted
into three sub-tokens: 'en', '##chan', '##ting'
the
hashtags indicate wether a sub-token was part of a bigger word or not,
this is useful to recover the human-readable strings later. The
[CLS]
token was added at a beginning and is intended to
represent the meaning of the whole sequence, likewise the
[SEP]
token was added to indicate that it is where the
sentence ends.
The next step is to give the sequence of tokens to the Encoder which processes it through the transformer layers and outputs a sequence of dense vectors:
PYTHON
with torch.no_grad():
output = model(**encoded_input)
print(output.last_hidden_state.shape)
print(output.last_hidden_state[0][0])
torch.Size([1, 21, 768])
tensor([-5.3755e-02, -1.1100e-01, -8.8204e-02, -1.1233e-01, 8.1979e-02,
-7.2656e-03, 2.5323e-01, -3.0361e-01, 1.7344e-01, -1.1212e+00, ...
We chose to print here the vector representation of
[CLS]
: by indexing the last_hidden_state[0]
we
access to the first batch (21 vectors of 768-dimensionality), and by
again indexing last_hidden_state[0][0]
we access the first
of the last_hidden_vectors, which as we saw in the token strings, belong
to [CLS]
and is there to represent the whole sequence. We
only see a lot of fine-tuned weights which are not very informative in
their own, but the full-vectors are meaningful within the embedding
space, which emulates some aspects of linguistic meaning.
Callout
In the case of wanting to obtain a single vector for enchanting, you can average the three vectors that belong to the token pieces that ultimately form that word. For example:
PYTHON
import numpy as np
tok_en = output.last_hidden_state[0][15].detach().numpy()
tok_chan = output.last_hidden_state[0][16].detach().numpy()
tok_ting = output.last_hidden_state[0][17].detach().numpy()
tok_enchanting = np.mean([tok_en, tok_chan, tok_ting], axis=0)
tok_enchanting.shape
We use the functions detach().numpy()
to bring the
values from the Pytorch execution environment (for example a GPU) into
the main python thread and treat it as a numpy vector for convenvience.
Then, since we are dealing with three numpy vectors we can average the
three of them and end op with a single enchanting
vector of
768-dimensions representing the average of
'en', '##chan', '##ting'
.
We can use the same method to encode two other sentences containing the word note to see how BERT actually handles polysemy (note means something very different in each sentence) thanks to the representation of each word now being contextualized instead of isolated as was the case with word2vec.
PYTHON
# Search for the index of 'note' and obtain its vector from the sequence
note_index_1 = string_tokens.index("note")
note_vector_1 = output.last_hidden_state[0][note_index_1].detach().numpy()
note_token_id_1 = token_ids[note_index_1]
print(note_index_1, note_token_id_1, string_tokens)
print(note_vector_1[:5])
We are basically printing the tokenized sentence from the previous
example and showing the index of the token note
in the list
of tokens. We are also printing the tokenID assigned to this token and
the list of tokens. Finally, the last print shows the first five
dimensions of the vector representing the token note
.
12 3805 ['[CLS]', 'Maria', "'", 's', 'passion', 'for', 'music', 'is', 'clearly', 'heard', 'in', 'every', 'note', 'and', 'every', 'en', '##chan', '##ting', 'melody', '.', '[SEP]']
[0.15780845 0.38866335 0.41498923 0.03389652 0.40278202]
Let’s encode now another sentence, also containing the word
note
, and confirm that the same token string, with the same
assigned tokenID holds a vector with different weights:
PYTHON
# Encode and then take the 'note' token from the second sentence
note_text_2 = "I could not buy milk in the supermarket because the bank note I wanted to use was fake."
encoded_note_2 = tokenizer(note_text_2, return_tensors="pt")
token_ids = list(encoded_note_2.input_ids[0].detach().numpy())
string_tokens_2 = tokenizer.convert_ids_to_tokens(token_ids)
note_index_2 = string_tokens_2.index("note")
note_vector_2 = model(**encoded_note_2).last_hidden_state[0][note_index_2].detach().numpy()
note_token_id_2 = token_ids[note_index_2]
print(note_index_2, note_token_id_2, string_tokens_2)
print(note_vector_2[:5])
12 3805 ['[CLS]', 'I', 'could', 'not', 'buy', 'milk', 'in', 'the', 'supermarket', 'because', 'the', 'bank', 'note', 'I', 'wanted', 'to', 'use', 'was', 'fake', '.', '[SEP]']
[ 0.5003222 0.653664 0.22919582 -0.32637975 0.52929205]
To be sure, we can compute the cosine similarity of the word note in the first sentence and the word note in the second sentence confirming that they are indeed two different representations, even when in both cases they have the same token-id and they are the 12th token of the sentence:
PYTHON
from sklearn.metrics.pairwise import cosine_similarity
vector1 = np.array(note_vector_1).reshape(1, -1)
vector2 = np.array(note_vector_2).reshape(1, -1)
similarity = cosine_similarity(vector1, vector2)
print(f"Cosine Similarity 'note' vs 'note': {similarity[0][0]}")
With this small experiment, we have confirmed that the Encoder produces context-dependent word representations, ass opposed to Word2Vec, where note would always have the same vector no matter where it appeared.
The Attention Mechanism
The original attention mechanism (remember this was developed for language translation) is a component in between the Encoder and the Decoder that helps the model to align the important information from the input sequence in order to generate a more accurate token in the output sequence:
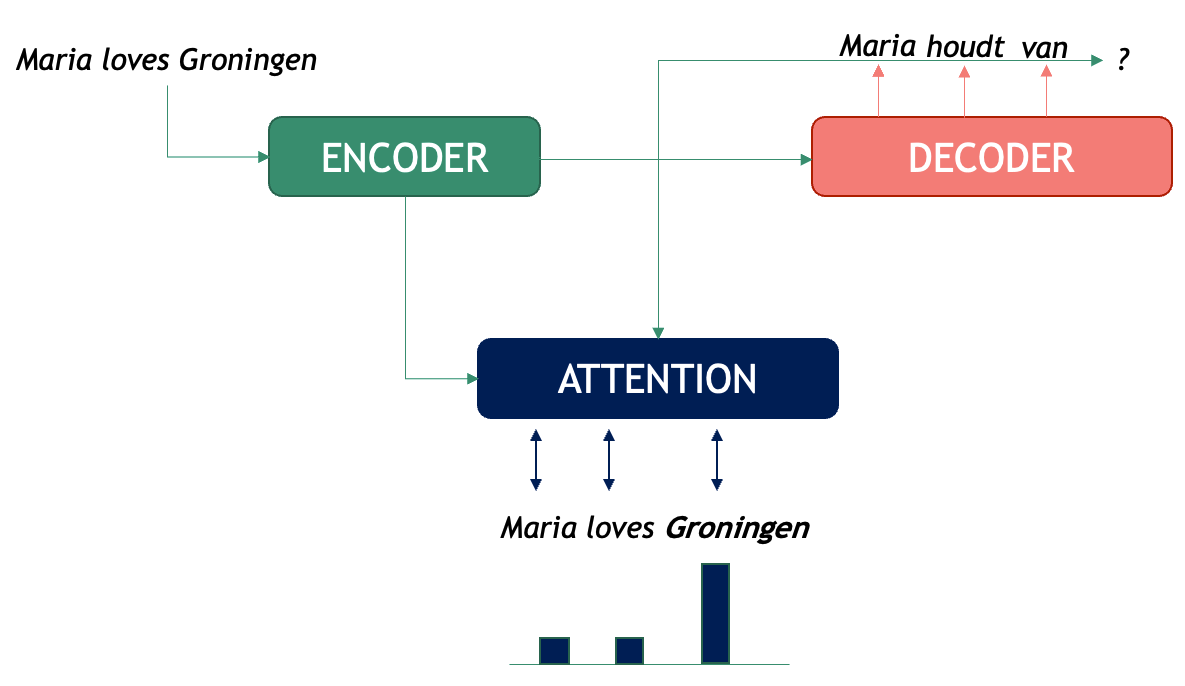
In the example above, the attention puts more weight in the input Groningen, so the decoder uses that information to know that is should generate Groningen. Note that if the decoder based it’s next word probability just on the sequence “Maria houdt van …”, it could basically generate any word and still sound natural. However, it is thanks to the attention mechanism that it preserves the meaning of the input sequence.
Attention is a neural layer, therefore it can also be plugged-in within the Encoder, this is called self-attention since the mechanism will look at the interactions between the input sequence itself (measure inportance between input sequence tokens vs input sequence tokens). This is how BERT uses (self-) attention, which is very useful to capture longer-range word dependencies such as correference, where, for example, a pronoun can be linked to the noun it refers to previously in the same sentence. See the following example:
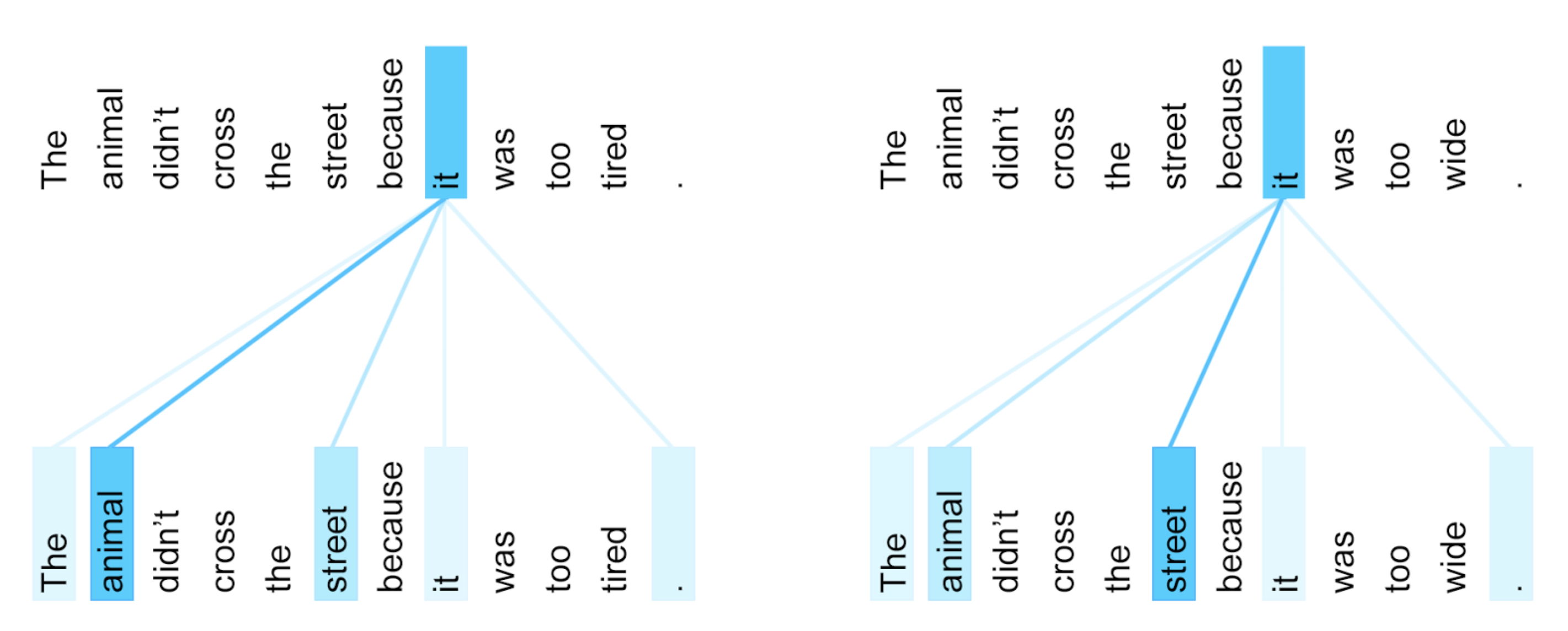
There are two sentences, in each one the pronoun “it” refers to a different noun, “animal” or “street”, and this is completely depending on the sentence context. Thanks to the self-attention BERT relates the pronoun to its relevant correferent.
For this reason BERT is not only useful as a text classifier but also for individual token classification tasks.
BERT for Token Classification
Just as we plugged in a trainable text classifier layer, we can add a token-level classifier that assigns a class to each of the tokens encoded by a transformer (as opposed to one label for the whole sequence). A specific example of this task is Named Entity Recognition, but you can basically define any task that requires to highlight sub-strings of text and classify them using this technique.
Named Entity Recognition
Named Entity Recognition (NER) is the task of recognizing mentions of real-world entities inside a text. The concept of Entity includes proper names that unequivocally identify a unique individual (PER), place (LOC), organization (ORG), or other object/name (MISC). Depending on the domain, the concept can expanded to recognize other unique (and more conceptual) entities such as DATE, MONEY, WORK_OF_ART, DISEASE, PROTEIN_TYPE, etcetera…
In terms of NLP, this boils down to classifying each token into a
series of labels (PER
, LOC
, ORG
,
O
[no-entity] ). Since a single entity can be expressed with
multiple words (e.g. New York) the usual notation used for labeling the
text is IOB (Inner Out
Beginnig of entity) notations which identifies the
limits of each entity tokens. For example:
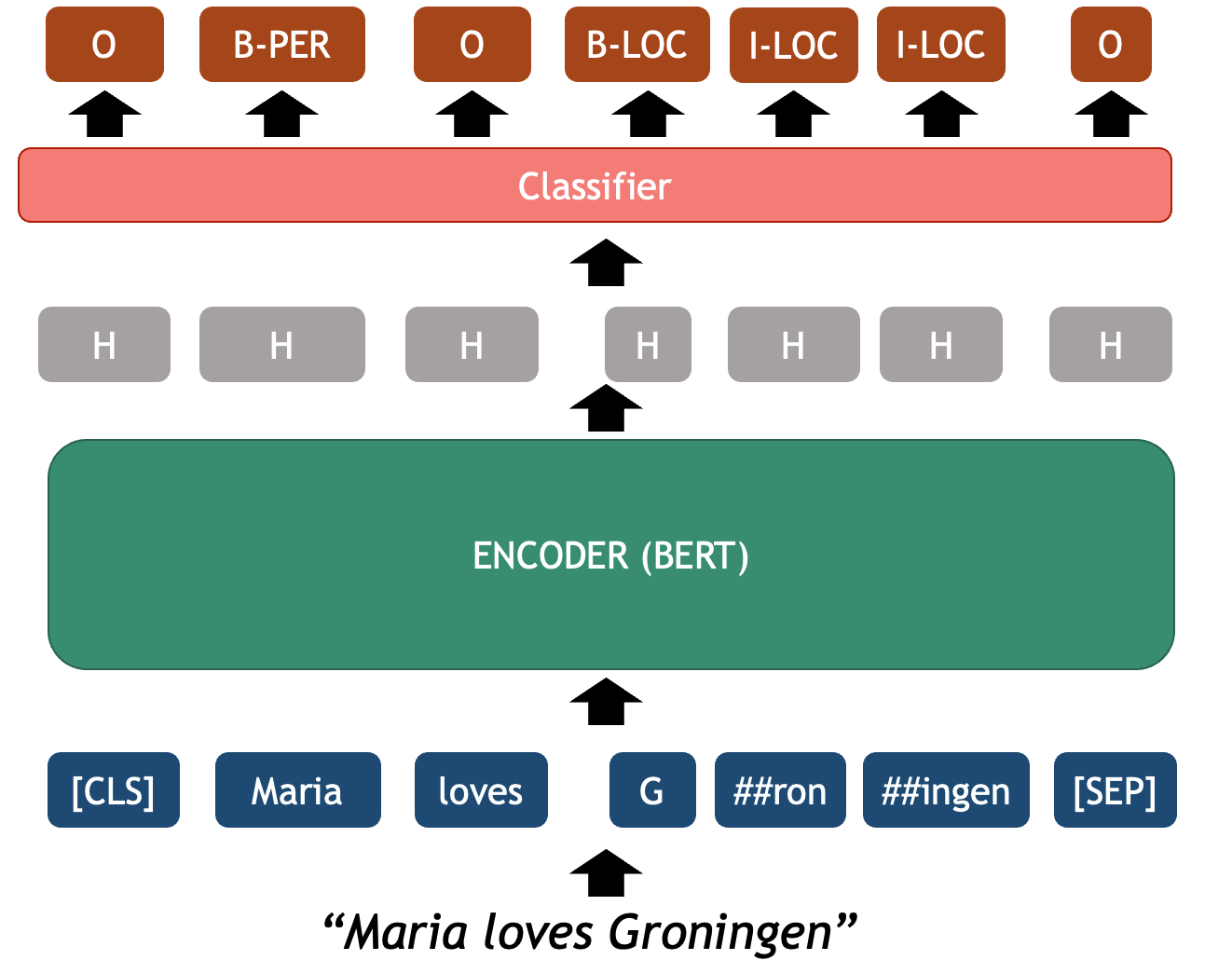
This is a typical sequence classification problem where an imput sequence must be fully mapped into an output sequence of labels with global constraints (for example, there can’t be an inner I-LOC label before a beginning B-LOC label). Since the labels of the tokens are context dependent, a language model with attention mechanism such as BERT is very beneficial for a task like NER.
Because this is one of the core tasks in NLP, there are dozens of
pre-trained NER classifiers in HuggingFace that you can use right away.
We use once again the pipeline()
to run the model for
predictions in your custom data, in this case with
task="ner"
. For example:
PYTHON
from transformers import AutoTokenizer, AutoModelForTokenClassification
from transformers import pipeline
tokenizer = AutoTokenizer.from_pretrained("dslim/bert-base-NER")
model = AutoModelForTokenClassification.from_pretrained("dslim/bert-base-NER")
ner_classifier = pipeline("token-classification", model=model, tokenizer=tokenizer)
example = "My name is Wolfgang Schmid and I live in Berlin"
ner_results = ner_classifier(example)
for nr in ner_results:
print(nr)
The code prints the following:
{'entity': 'B-PER', 'score': 0.9996068, 'index': 4, 'word': 'Wolfgang', 'start': 11, 'end': 19}
{'entity': 'I-PER', 'score': 0.999582, 'index': 5, 'word': 'Sc', 'start': 20, 'end': 22}
{'entity': 'I-PER', 'score': 0.9990482, 'index': 6, 'word': '##hm', 'start': 22, 'end': 24}
{'entity': 'I-PER', 'score': 0.9951691, 'index': 7, 'word': '##id', 'start': 24, 'end': 26}
{'entity': 'B-LOC', 'score': 0.99956733, 'index': 12, 'word': 'Berlin', 'start': 41, 'end': 47}
In this case the output of the pipeline is a list of dictionaries,
each one representing only entity IOB
labels at the BERT
token level. IMPORTANT: this list is per wordPiece and NOT per human
word even if the provided text is pre-tokenized. You can assume all
of the tokens that don’t appear in the output were labeled as no-entity,
that is "O"
. To recover the full-word entities you can
initialize the pipeline with
aggregation_strategy="first"
:
PYTHON
ner_classifier = pipeline("token-classification", model=model, tokenizer=tokenizer, aggregation_strategy="first")
example = "My name is Wolfgang Schmid and I live in Berlin"
ner_results = ner_classifier(example)
for nr in ner_results:
print(nr)
The code now prints the following:
{'entity_group': 'PER', 'score': 0.9995944, 'word': 'Wolfgang Schmid', 'start': 11, 'end': 26}
{'entity_group': 'LOC', 'score': 0.99956733, 'word': 'Berlin', 'start': 41, 'end': 47}
As you can see, entities aggregated at the Span Leven (instead of the
Token Level). Word pieces are merged back into human words and
also multiword entities are assigned a single entity label unifying the
IOB
labels into one. Depending on your use case you can
request the pipeline to give different
aggregation_strateg[ies]
. More info about the pipeline can
be found here.
The next step is crucial: evaluate how does the pre-trained model actually performs in your dataset. This is important since the fine-tuned model could be overfitted to other custom benchmarks that do not share the characteristics of your dataset.
To observe this, we can first see the performance on the test portion of the dataset in which this classifier was trained, and then evaluate the same pre-trained classifier on a NER dataset form a different domain.
———- END HERE ??? ———-
The rest is more advanced content (still I leave it here just in case for now).
Testing on CoNLL-03 Benchmark
This model was trained on the CoNLL-03 dataset, therefore we can
corroborate how it performs using the test portion of this dataset. To
get the data we can use the datasets
library which is also
part of theHuggingFace landscape
pip install datasets
PYTHON
from datasets import load_dataset
conll03_data = load_dataset("eriktks/conll2003", split="test", trust_remote_code=True)
conll03_data
This shows the features and number of records of the CoNLL-03 Dataset. Next we can observe which labels we have in the data
As expected, the labels are in IOB notation, where each label corresponds to one word in the dataset, however the dataset contains the labelIDs and we need to map them to their string representations. We can double check this by looking at one of the records of the dataset:
PYTHON
def labelid2str(label_int):
d = conll03_data.features['ner_tags'].feature._int2str
return d[label_int]
example_id = 10
print(conll03_data['tokens'][example_id])
print(conll03_data['ner_tags'][example_id])
print([labelid2str(tag) for tag in conll03_data['ner_tags'][example_id]])
These are the Gold Labels of the dataset. We can use our pre-trained BERT model to predict the labels for each example and compare the outputs to the gold labels provided in the data.
Predictions using Pipeline
This could be done using the pipeline as we have been doing so far, example by example:
PYTHON
from transformers import AutoTokenizer, AutoModelForTokenClassification
from transformers import pipeline
def get_gold_labels(label_ids):
return [labelid2str(tag) for tag in label_ids]
def token_to_spans(tokens):
token2spans = {}
char_start = 0
for i, tok in enumerate(tokens):
tok_end = char_start + len(tok)
token2spans[i] = (char_start, tok_end)
char_start = tok_end + 1
return token2spans
def get_iob_from_aggregated(tokenized_sentence, entities):
# Initialize all labels empty
iob_labels = ['O'] * len(tokenized_sentence)
# Get Token <-> Chars Mapping
tok2spans = token_to_spans(tokenized_sentence)
start2tok = {v[0]:k for k, v in tok2spans.items()}
end2tok = {v[1]:k for k, v in tok2spans.items()}
# Iterate over each entity to populate labels
for entity in entities:
label = entity['entity_group']
token_start = start2tok.get(entity['start'])
token_end = end2tok.get(entity['end'])
if token_start is not None:
iob_labels[token_start] = f'B-{label}'
if token_end is not None:
for i in range(token_start+1, token_end+1):
iob_labels[i] = f'I-{label}'
return iob_labels
tokenizer = AutoTokenizer.from_pretrained("dslim/bert-base-NER")
model = AutoModelForTokenClassification.from_pretrained("dslim/bert-base-NER")
example = conll03_data['tokens'][example_id]
example_str = " ".join(example)
ner_classifier = pipeline("ner", model=model, tokenizer=tokenizer, aggregation_strategy="first")
predictions = ner_classifier(example_str)
print("SENTENCE:", example_str)
print("PREDICTED:", get_iob_from_aggregated(example, predictions))
print("GOLD:", get_gold_labels(conll03_data['ner_tags'][example_id]))
Now that we understand how to get a list of Predicted labels for one example we can run the model for the whole test data:
PYTHON
all_predictions = []
for example in conll03_data['tokens']:
output = ner_classifier(" ".join(example))
predictions = get_iob_from_aggregated_simple(example, output)
all_predictions.append(predictions)
gold_labels = [get_gold_labels(lbl) for lbl in conll03_data['ner_tags']]
We can use the seqeval
package to directly evaluate the
outputs:
PYTHON
from seqeval.metrics import classification_report
report = classification_report(gold_labels, all_predictions)
print(report)
The three most basic metrics for NLP classifiers are traditionally Precision, Recall and F1 score. They come from the Information Extraction field and roughly they aim to measure the following: - Precision (P): From the predicted entities, how many of them are correct (i.e. match the gold labels)? - Recall (R): From the known gold entities, how many of them were predicted by the model? - F1 Score (F1): the harmonic mean of precison and recall, which aims to provide a balance between both metrics. It has two variants: the Micro-F1 which treats all errors equally, being the same as measuring Accuracy; and Macro-F1, which aims to show the model performance taking into account the label distribution, this is normally the score reported through main benchmarks as it shows better the model’s weaknesses across classes.
Using a Pre-trained Model on LitBank
We can of course also use the pre-trained NER classifier with any custom dataset, it will just need come pre- and post-processing steps to make it work. For this example, we will use the LitBank corpus, an annotated dataset of 100 works of English-language fiction to support tasks in natural language processing and the computational humanities. Specifically they have human annotations of entities on these books. We can measure how good is this pre-trained classifier by making the model predict the entities inside the text and them compare the outputs with the humam annotations. The NER portion of the dataset we will use is the tabulated data from here and one example looks like this:
Index | Token | IOB-1 | IOB-2 | IOB-3 | IOB-4 |
---|---|---|---|---|---|
1 | CHAPTER | O | O | O | O |
2 | I | O | O | O | O |
3 | In | O | O | O | O |
4 | Chancery | B-FAC | O | O | O |
5 | London | B-GPE | O | O | O |
6 | . | O | O | O | O |
It contains the information of 4 annotators, this is very useful interannotator agreement, a technique in computational linguistics for validating the correctness and consistency of the dataset. Yes! Humans are wrong too all the time when labeling! For simplicity, we will assume we only have the information from annotator 1 and take that as our ground truth.
The format of the dataset resembles the conll format, a widely used format in computational linguistics for token-based annotations. Another important aspect to observe is that they have other labels for entities. The pre-trained model we chose only labels PER, LOC, ORG and MISC. We can translate FAC and GPE to LOC label as they are only more fine-grained occurrences of locations which our model should recognize as such. To read the data we can use the following function:
PYTHON
def quick_conll_reader(filepath):
all_sentences, all_labels = [], []
sent_txt, sent_lbl = [], []
label_vocab = {}
gold_label_column = 1
label_translator = {
"B-FAC": "O",
"I-FAC": "O",
"B-GPE": "B-LOC",
"I-GPE": "I-LOC",
"B-VEH": "O",
"I-VEH": "O"
}
with open(filepath) as f:
for line in f.readlines():
row = line.strip().split("\t")
if len(row) > 1:
sent_txt.append(row[0])
label = row[gold_label_column]
if label in label_translator:
final_label = label_translator[label]
else:
final_label = label
sent_lbl.append(final_label)
if final_label not in label_vocab:
label_vocab[final_label] = len(label_vocab)
else:
all_sentences.append(" ".join(sent_txt))
all_labels.append(sent_lbl)
sent_txt, sent_lbl = [], []
return all_sentences, all_labels, label_vocab
sentences, gold_labels, label_vocab = quick_conll_reader("1023_bleak_house_brat.tsv")
print(sentences[0].split(' '))
print(gold_labels[0])
This code processes the Bleak House book and extracts a list of tokenized sentences (as strings) and a list of IOB Labels corresponding to each token in the sentence. You can see the first sentence and its corresponding list of gold labels on this example. Next, we load the NER pre-trained model again and process the sentences to obtain model predictions. The problem here is that the model predictions are lists of dictionaries and we need to post-process them so they are also on IOB-format. We use the get_iob_labels() function to do this conversion.
PYTHON
def token_to_spans(tokens):
token2spans = {}
char_start = 0
for i, tok in enumerate(tokens):
tok_end = char_start + len(tok)
token2spans[i] = (char_start, tok_end)
char_start = tok_end + 1
return token2spans
def get_litbank_labels(tokenized_sentence, entities):
# Initialize all labels empty
iob_labels = ['O'] * len(tokenized_sentence)
# Get Token <-> Chars Mapping
tok2spans = token_to_spans(tokenized_sentence)
start2tok = {v[0]:k for k, v in tok2spans.items()}
end2tok = {v[1]:k for k, v in tok2spans.items()}
# Iterate over each entity to populate labels
for entity in entities:
label = entity['entity_group']
if label == "MISC": # Design choice: Do NOT count MISC entities!
continue
token_start = start2tok.get(entity['start'])
token_end = end2tok.get(entity['end'])
if token_start is not None:
iob_labels[token_start] = f'B-{label}'
if token_end is not None:
for i in range(token_start+1, token_end+1):
iob_labels[i] = f'I-{label}'
return iob_labels
And we finally apply the model to the sentences that we previously read:
PYTHON
ner_results = ner_classifier(sentences)
model_predictions = []
for i, sentence_ner in enumerate(ner_results):
print(f"\n===== SENTENCE {i+1} =====")
print('Tokens:', sentences[i].split())
print('GOLD:', gold_labels[i])
# Get the IOB labels for the tokenized sentence
tokenized_sentence = sentences[i].split()
predicted_iob_labels = get_litbank_labels(tokenized_sentence, sentence_ner)
model_predictions.append(predicted_iob_labels)
print('MODEL:', predicted_iob_labels)
for nr in sentence_ner:
print(f'\t{nr}')
For each model prediction we are printing the sentence tokens, the IOB gold labels and the IOB predicitons. Now that the data is in this shape we can perform evaluation.
Model Evaluation
To perform evaluation in your data you can use again the
seqeval
package:
PYTHON
from seqeval.metrics import classification_report
print(classification_report(gold_labels, model_predictions))
Since we took a classifier that was not trained for the book domain, the performance is quite poor. But this example shows us that classifiers performing very well on their own domain most of the times transfer poorly to other apparently similar datasets.
The solution in this case is to use another of the great characteristics of BERT: fine-tuning for domain adaptation. It is possible to train your own classifier with relatively small data (given that a lot of linguistic knowledge was already provided during the language modeling pre-training). In the following section we will see how to train your own NER model and use it for predictions.