Content from Introduction to FAIR Data Management for Long-term Agriculture Experiments
Last updated on 2024-12-03 | Edit this page
Overview
Questions
- What will I learn from this lesson?
Objectives
- Understand the purpose of this lesson.
- Explain why good data management is important for long-term agricultural experiments.
Introduction
Who is this lesson for?
This lesson is aimed at long-term agricultural experiment (LTE) managers, and researchers interested in using long-term agricultural experiment data, who want to apply FAIR principles and good research data management practices to their LTE data. While the target audience for this lesson is therefore LTE managers and researchers, the lesson covers many data management and FAIR data topics relevant to a broader audience working on agricultural field trials.
The lesson is split into eight episodes:
- Introduction to FAIR Data Management for Long-term Agriculture Experiments
- Why should we share long-term experiment data
- FAIR Principles for long-term agricultural experiments data
- Metadata for long-term experiments
- Organising data for long-term experiments
- Making LTE data FAIR
- Using databases to manage Long-term experiment data
- Publishing LTE data
What are long-term agricultural experiments?
Long-term agricultural experiments are multi-year field experiments, testing a range of different treatments to assess the sustainability of crop production and soil health over time (Johnston & Poulton, 2018). There is no strict definition for when an experiment becomes long-term, but it is generally accepted that experiments can be long-term if they have between 10 to 20 years of continuous treatments; the longest running experiment is Broadbalk wheat, located at Rothamsted, UK, and has been running for 180 years. Globally there are many hundreds of LTEs. The Global Long-Term agricultural Experiments Network - GLTEN lists over 290 experiments world wide, while the German BonaRes repository contains information for over 450 LTEs in Europe.
LTEs consist of multiple experiment plots, each receiving a different combination of treatments over time. Each plot receives the same set of treatments and this can result in very different soil environments, with gradients of soil pH, nutrient availability, or soil organic matter arising over time.
Experimental treatments tested on LTEs are very diverse and can range from whole system comparisons, such as organic vs inorganic agriculture, cropping system comparisons such as monocrop vs different crop rotations, and individual management practices such as nutrient inputs, crop residue management, organic amendments, and tillage and cultivation practices.
Callout
Review some of experiments listed on the Global Long-Term agricultural Experiments Network - GLTEN metadata portal and have a look at the diversity of different treatments used.
Why are LTEs and their data important?
LTEs are important sources of long-term data on crop productivity and soil health which can be used to assess the sustainability of different agricultural practices and farming systems overtime. Often the trends and processes observed in LTEs can take many years, even decades to manifest, and this data cannot easily be reproduced. LTEs are also expensive to maintain, because it can take several years before useful data is generated, meaning research organisations and funders have to commit to long-term investments.
Today, as society attempts to address global challenges faced by climate change and food security, this data can provide critical insights and knowledge to help us adapt to and address these challenges.
Why do we need good data management for LTEs
Discussion
Working in groups, identify reasons why good research data management should be a critical activity for Long-term Agricultural Experiments.
Record your reasons to share with the other participants.
Very often LTEs will span several generations of researchers, therefore good data management is critically important to ensure the data created by one generation of researchers is usable by the next generation. Good research data management is necessary to maintain the continuity and the interpretation of LTE data over time. Data management for LTEs needs to think more broadly about the information necessary to understand an LTE. Data managers need to consider not only the data itself but how the data is created, for example are sampling and analytical methods the same over time, and if not does this affect the interpretation of the data. LTE data managers also need to consider the experiment history and the decisions made that affect the experiment, such as a change in management practices, crop varieties, or treatments. Together this information, or metadata, can be critical for the correct interpretation of the data.
LTE data managers must also ensure LTE data is both secure and remains accessible overtime. LTE data needs to be kept stored in accessible storage using accessible and open data formats. Accessible can also mean the how the data is described and this can change overtime. For example, Rothamsted’s Broadbalk experiment originally recorded grain yields as bushels, then lbs acre-1 and today as t ha-1. Each change necessitates a conversion which must be documented to ensure ongoing trust in the data provenance.
For a more detailed overview of some of the data management challenges facing long-term experiments, based on the Rothamsted Long-term experiments see Ostler et al, 2023.
Key Points
Good research data management for LTE data matters because
- LTE data is costly to produce
- LTE data is unique and cannot be reproduced
- LTE data takes many years to generate
- Good RDM is necessary to ensure the continuity of data over time
- Good RDM is necessary to ensure the accessibility of data over time
- Good RDM is necessary to ensure the usability and interpretation of data over time
Content from Why should we share long-term experiment data
Last updated on 2024-12-03 | Edit this page
Overview
Questions
- Why should you share data?
- What are the motivations for sharing LTE data?
Objectives
- Understand the wider context for data sharing.
- Explain how sharing data can increase impact of your data and research.
- Understand how sharing data can increase the return on investment to funders.
- Understand how sharing data supports scientific integrity.
What is open science?
Open science is the movement to make scientific research outputs accessible to all. Open science research outputs include articles, datasets, physical samples, protocols, and code. These outputs are the building blocks of the open science movement.
What is open data?
Open data is a key building block of the open science movement and key to supporting scientific integrity and reproducibility, but what does open data mean? The Open Knowledge Foundation’s Open Data Handbook defines Open Data as “data that can be freely used, re-used and redistributed by anyone - subject only, at most, to the requirement to attribute and sharealike”.
The full open data definition gives precise details as to what this means in practice. These are:
- Data must be freely available, and at no more than the cost of reproduction.
- Data must me available in a modifiable and convenient form.
- Data must be distributed with terms allowing for its reuse and redistribution.
- Everyone must be able to use the data, there must be no restrictions based on intended use or user.
Does data have to be open to be shareable?
No. Data can be shared even if it isn’t open. ‘Data available on request’ is still a common practice in research article data availability statements. sucha a statement means the data is not open, but the data can be shared. As we will see in the next episode on FAIR data, data can be FAIR but not open.
What are the motivations for open science and data sharing?
Discussion
Working in groups, identify reasons why many organisations, funders, and governments are promoting open science and data sharing.
- Think about the different motivations and who benefits.
- How might these motivations change for LTE data?
Return on Investment
A lot of research is funded by the public, either through charities or taxes. For example, Horizon Europe has a €95.5 billion budget for 2021-27, and the Bill and Melinda Gates Foundation aims to pay $9 billion by 2026. But, according to EU report “Cost-benefit analysis for FAIR research data”, €10.2bn is lost every year because of not accessible data. Funders, who are spending billions on research, have a self-interested motivation to have a return on their investments. One method for doing that is to ensure the data they pay to generate is reused as widely as possible, beyond its original purpose. Funders may be especially keen to see data which is either expensive to generate or difficult to replicate shared.
Many funders have therefore chosen to adopt policies actively encouraging and in some cases mandating open data sharing: For example, the following funders all promote open data sharing in their data policies:
- Bill and Melinda Gates Foundation
- National Science Foundation (US)
- CGIAR (International)
- USAID
- Horizon Europe
- Biodiversa
- UKRI (UK)
Moral Imperative
For publicly funded research there is a moral imperative to make data openly available. Public money should be invested for public good, namely the public should benefit by not having to pay to access research they already funded, and reducing duplication of effort.
Reproducbility, transparency, and accountability
Open Science by its nature helps to address reproducibility in science. Sharing data allows other researchers to replicate and results and validate their provenance. This helps to build transparency and trust in research findings.
By making data openly available researchers can more easily counteract narratives which seek to deliberately misinterpret the data by selectively analysing the data.
Personal reward
Open data works best when researchers are incentivised and rewarded for sharing their data. There is a growing body of evidence that sharing data can lead to increased citations. Allowing other researchers to reuse your data can increase the impact of your research, and if datasets are cited this impact can be measured and reported. Adding accessible data as a research output can therefore increase researcher reputation, and help to build communities around reuse of data.
Organisations are developing infrastructures to recognise open science practices. For example the Declaration on Research Assessment (DORA) aims to recognise datasets as important research outputs which should be considered by funders and institutions in their assessment processes.
Long-term data
Long-term experiments pull together several motivations for making data accessible. Long-term data is costly to produce, unique and irreplaceable and can be used to address a wide variety of research questions. The types of questions that can be asked from LTE increases if there are opportunities to combine and analyse their data using new methods. For example, Maclaren et al (2022) combined data from 30 LTEs in Africa and Europe to analyse the interactions between different management practices.
In the UK, the Biotechnology and Biological Sciences Research Council data sharing policy, who fund the Rothamsted Long-term experiments, identify the low throughput cumulative long times series data generated by long-term experiments as having an especially strong scientific case for data sharing.
Long-term experiments can directly benefit from sharing data by establishing new collaborations with researchers using the data. In our opinion, it is not necessary for an LTE manager to require co-authorship on every article authored by a researcher using LTE data, however, it is our experience from the Rothamsted LTEs that researchers will often seek support to interpret LTEs from experiment managers and data curators and this naturally leads to co-authorship.
Who uses LTE data
Use the Rothamsted Long-term Experiments Bibliography to see how many different research areas have used the Broadbalk experiment.
- Agronomy
- Weed ecology
- Soil science
- Soil metagenomics
- Plant nutrition
- Nitrogen Use efficiency
- Crop and soil model development
- Soil carbon dynamics
- Atmospheric sciences
- Disease and disease resistance
LTE data is therefore inherently valuable to a wide research community. By making LTE data openly available, LTE managers can increase the impact of the experiment through data reuse and use this to evidence ongoing impact to funders.
Key Points
- Open science aims to remove barriers to accessing research outputs.
- Open data is a key building block of Open Science.
- There are different motivations for sharing data including.
- LTE data is inherently valuable with relevance to multiple research areas.
Content from FAIR Principles for long-term agricultural experiments data
Last updated on 2024-12-03 | Edit this page
Overview
Questions
- What are the FAIR Principles?
- Why should we care about FAIR principles for LTE data?
- Are FAIR data and Open data the same?
Objectives
- Understand the FAIR Principles.
- Explain the advantages of adopting FAIR for LTE data.
- Explain the difference between FAIR and Open data.
Introduction
The FAIR Guiding Principles for scientific data management and stewardship were published in 2016 and comprise of 15 guiding principles which aim to: - Remove obstacles and improve the infrastructure to support data discovery and reuse. - Provide concise and measurable principles for enhancing reusability of data.
In the previous episode on data sharing we learned LTE data is a valuable resource with good potential for reuse, but simply putting data online is not enough. The FAIR guiding principles provide a valuable framework for describing how datasets can be provisioned to make them findable, accessible and reusable by other researchers, and supporting the long-term stewardship of LTE data. We can apply FAIR principles to LTE datasets to maximise their potential reach and impact, and safeguard them for future generations who will manage LTE data after us.
In this lesson we will explore in detail what the practical application of FAIR principles means for dataset metadata. In the following episodes we will explore application of FAIR principles to the data.
Findable data
Metadata and data should be easy to find for both humans and computers. Machine readable persistent identifiers and metadata allow data to be easily and reliably found.
- F1 (Meta)data are assigned a globally unique and persistent identifier
- F2 Data are described with rich metadata (defined by R1 below)
- F3 Metadata clearly and explicitly include the identifier of the data they describe
- F4 (Meta)data are registered or indexed in a searchable resource
Challenge 1: Findable (meta)data
In a new browser window, navigate to Google Dataset Search, and find the dataset “Replication Data for: Soil Biological Indices in Short and Long-Term Experiments in Kenya”. Which F principles in action.
F1: Assigned a DOI (document object identifier) https://doi.org/10.7910/DVN/4GVGAP.
F2: Has rich metadata to describe the dataset.
F3: Metadata includes the DOI.
F4: Metadata is registered in a dataset repository (Harvard Dataverse).
F4: Metadata can be indexed in searchable resource (Google dataset search).
Accessible (meta)data
Once the user finds the required data, she/he/they need to know how it can they be accessed, either openly or restrictively using authentication and authorisation.
- A1 (Meta)data are retrievable by their identifier using a standardised communications protocol
- A1.1 The protocol is open, free, and universally implementable
- A1.2 The protocol allows for an authentication and authorisation procedure, where necessary
- A2 Metadata are accessible, even when the data are no longer available
Challenge 2: Accessible (meta)data in action
Using the dataset “Replication Data for: Soil Biological Indices in Short and Long-Term Experiments in Kenya”, identify the A principles in action.
A1: Dataset can be retrieved by its DOI (identifier) over http (standardised communication protocol)
A1.1: http is an open, free and universally implementable protocol.
A1.2: although it is not used here, http supports authentication and authorisation.
A2: We cannot test this using this example as the dataset is available with the metadata.
Interoperable metadata
Interoperability is the process of making it easier to combine different datasets and allowing data and metadata to be accessed and used by applications or workflows for analysis, storage, and processing.
- I1 (Meta)data use a formal, accessible, shared, and broadly applicable language for knowledge representation.
- I2 (Meta)data use vocabularies that follow FAIR principles.
- I3 (Meta)data include qualified references to other (meta)data.
Challenge 3: Interoperable metadata in action
Using the dataset “Replication Data for: Soil Biological Indices in Short and Long-Term Experiments in Kenya”, identify the I principles in action.
View the page source and look for application/ld+json
I1: Metadata is represented as Dublin Core metadata tags and schema.org Dataset context (this is what Google looks for and uses).
I2: Metadata is annotated using AGROVOC keywords.
I3: Authors are identified by ORCID identifiers.
I3: Related publications are linked to.
Reusable metadata
The ultimate goal of FAIR is to enable the reuse of data. To achieve this, metadata and data should be well-described, following community data standards, and a clear and accessible licence, describing how the data can and cannot be reused, remixed or redistributed.
- R1 (Meta)data are richly described with a plurality of accurate and relevant attributes
- R1.1 (Meta)data are released with a clear and accessible data usage license
- R1.2 (Meta)data are associated with detailed provenance
- R1.3 (Meta)data meet domain-relevant community standards
Challenge 4: Reusable metadata in action
Using the dataset “Replication Data for: Soil Biological Indices in Short and Long-Term Experiments in Kenya”, identify the R principles in action.
View the page source and look for application/ld+json
R1 Data are described with rich metadata.
R1.1 The data is licenced under CC-BY-4.0 (Creative Commons), but the phrasing “Custom dataset terms” could cause confusion.
R1.2 There is information about provenance
R1.3 Metadata are available as inline JSON+LD following the schema.org @dataset context and as DublinCore meta elements in the html.
Following FAIR principles supports good research data management practice
Applying FAIR principles to your datasets encourages you to adopt good research data management practices.
Using FAIR Assessment Tools
As more researchers move to adopt FAIR principles, assessment tools are being developed to score how well datasets meet the FAIR principles. For example the [F-UJI Automated FAIR Data Assessment Tool] (https://www.f-uji.net/index.php), provides an automated assessment, while the CGIAR have developed a FAIR scoring system based on Netherlands Institute for Permanent Access to Digital Research Resources (DANS) metrics for FAIR compliance. These tools can be used to provide a basic assessment of the metadata quality and data repository infrastructure hosting the dataset.
Using FAIR assessment tools
Use the [F-UJI Automated FAIR Data Assessment Tool] (https://www.f-uji.net/index.php) to assess how FAIR the dataset “Replication Data for: Soil Biological Indices in Short and Long-Term Experiments in Kenya” is.
- Have a look at other datasets in common repositories such as Zenodo, and Figshare.
- For datasets published in Gardian, compare the Gardian FAIR Metrics score with the F-UJI score. Do the results surprise you?
FAIR does not equal Open Data
The FAIR guiding principles do not mandate data must also be open, and open data does not have to follow FAIR principles, but it will be easier to find, access and reuse if it does.
With principle R1.1, FAIR principles do mandate metadata must describe how data can be accessed. However, while FAIR does not require the data is open, principle A2, that metadata are accessible, even when the data are no longer available, means FAIR does require open metadata.
Challenge 5. How can FAIR data also be restricted access data?
List different ways datasets can be FAIR, but have their access to reuse them restricted?
Think about
- different options for licencing data
- timelines for publishing data
- Principle A1.2
- Data can be published using a restrictive licence, for example limiting commercial reuse of the data
- Data may be embargoed before publication of the results
- Data may be behind a registration wall requiring some authentication and authorisation process such as username and password. Registering may be an automatic process, while not strictly restricted it does create a barrier to access.
- Data may be freely available but not readily available, i.e ‘available on request’. While not strictly restricted it does create a barrier to access.
Publishing FAIR metadata for embargoed datasets
It is reasonable for researchers to expect a period of exclusive use over the data that they generate to give them time to analyse it and publish the results.
Discussion
The authors of this dataset on simulated maize and wheat data have embargoed the datasets until July 2023. Why might the authors have chosen to do this?
Reasons to publish FAIR metadata for embargoed datasets
Publishing FAIR metadata for embargoed datasets has several benefits including:
- Metadata can be published before the results, meaning there is a citable dataset that can be referenced in articles.
- Other researchers can find the dataset and request access. This may lead to new collaborations and earlier impact from secondary reuse.
- Some data repositories can automatically remove the embargo on a set date.
Reasons to go FAIR
why should you adopt FAIR Guiding Principles
Working in groups list reasons why you should adopt and follow FAIR Guiding Principles.
Think about what the benefits are, who benefits and what the costs.
Key Points
- Applying FAIR Principles make it easier to find research data.
- Following FAIR principles makes you start to follow best practices for research data management.
- FAIR data is not open data, but open data should be FAIR data.
- Good research data management through adopting FAIR principles has a cost.
Content from Metadata for long-term experiments
Last updated on 2024-12-03 | Edit this page
Overview
Questions
- What is metadata?
- Why do we need metadata for?
- What metadata should we collect for LTEs?
Objectives
- Recognise what metadata is.
- Distinguish different types of metadata.
- Understand what makes metadata interoperable.
- Know how to decide what to include in metadata.
What is metadata
Metadata is data about the data; metadata provides a description of our data. We need metadata to help us understand the data and how to interpret it. Therefore, metadata plays an important role in making our FAIR.
Types of metadata
We can distinguish three types of metadata:
Administrative metadata: Information about a project or resource that are relevant for managing it, for example: principle investigator, project collaborators, contacts, funders and grant details, project data management plan, project period, location, etc. This information is usually assigned to the data before you start collecting, but it can change and be added to, so it is important to keep it up to date!
Descriptive and citation metadata: Information about a dataset that allows other researchers to discover and identify it, for example, authors, abstract, keywords, persistent identifier such as a DOI, licence, related publications, and research outputs.
Structural metadata: Information about how the data was created and how it is structured. For example, experiment design and treatment factors, sampling protocols, analytical protocols, measured variables and observations. For an LTE structural metadata can also include information about the experiment management, for example sowing and harvest dates, pest management, tillage and cultivation practice, fertilizer inputs, and environmental information such as soil properties, and climate. Often the difference between metadata and data can be blurred. Structural metadata should be gathered according to best practices used in your research community.
Descriptive and structural metadata should be added continuously throughout the project.
Identifying Metadata
Review the lesson datasets.
What types of metadata do they collect?
Are they missing key metadata?
How consistent are the datasets in their metadata?
How do they do they differ?
Do you think the metadata is FAIR?
Metadata to describe a long-term experiment
Good metadata are crucial for ensuring LTE data is understandable and therefore reusable by other researchers. The type of metadata collected often depends on the context for the data. Administrative and citation metadata are normally common across different datasets and experiments, for example, a field experiment and a lab experiment will both have information about PIs, funding, abstract, keywords, and identifiers. The structural metadata will depend on the context. For example, a glass house experiment and a field experiment might share the same type of metadata to describe the experimental design and the different treatment factors, but different metadata to characterise their environment and management. Equally different types of data will have specific information about how that data originated. For example, soil chemistry data and yield data will have different metadata about their sampling and analytical methods, but will share metadata about the experiment and environment.
Challenge 2.1. Metadata to describe a long-term experiment
Make a list of the different metadata you should collect to describe your experiment (we’ll look at the data later).
GLTEN Metadata Schema
The GLTEN Metadata Schema has been developed by the Global Long-term Experiment Network to provide a consistent description for long term agricultural experiments.
The schema provides detailed metadata to describe a long-term experiment including: - Administration - Cropping and rotations - Experiment treatment factors - Soil and climate environmental properties - Links to related sources including datasets and publications
challenge 2.2. Review the GLTEN schema
Use the GLTEN metadata portal to review find the metadata records for our four experiments, how does the metadata compare with your list? You can view the full GLTEN metadata schema on Github.
Making Metadata FAIR
Collecting good metadata is essential, but we can make it more Findable and Interoperable by using structured metadata schemas and annotating by using and controlled vocabularies.
Callout
Schemas are machine processable specifications which define the structure and syntax of metadata specifications in a formal schema language. The schema defines terms to represent key items of information about the resource they describe. For example, a term spatial would describe the spatial coverage of a dataset resource.
Core metadata schemas
Core metadata schemas are used to aid resource discovery. Several schemas exist and it is useful to understand their different roles, and how they link to each other.
DublinCore DCMI Metadata Terms
This is an ISO standard schema defining 15 core elements for describing a resource. It is widely used by data repositories and can be found in web pages as meta tags with a DC. prefix, for example:
<meta name="DC.identifier" content="doi:10.7910/DVN/4GVGAP" />
<meta name="DC.type" content="Dataset" />
<meta name="DC.title" content="Replication Data for: Soil Biological Indices in Short and Long-Term Experiments in Kenya" />
<meta name="DC.date" content="2019-07-08" />
<meta name="DC.publisher" content="Harvard Dataverse" />
Webpages with DC metadata tags can be indexed by tools such as Google Dataset Search
Schema.org @dataset context
Schema.org is a collaborative effort founded by Google, Microsoft, Yahoo and Yandex to create structured schemas for the web. The @dataset type is sub set of schema.org used to describe a dataset. Like DCMI terms, it is widely used by data repositories and can be found in web pages, normally as inline JSON+LD, for example:
<script type="application/ld+json">
{
"@context":"http://schema.org",
"@type":"Dataset",
"@id":"https://doi.org/10.7910/DVN/4GVGAP",
"identifier":"https://doi.org/10.7910/DVN/4GVGAP",
"name":"Replication Data for: Soil Biological Indices in Short and Long-Term Experiments in Kenya"
</script>
Minimum Information Schemas
Minimum Information Schemas are specialised metadata schemas which aim to provide consistent and deeper description of a resource. We have already seen the GLTEN metadata schema which is really a minimum information schema to describe a long-term experiment. Another schema relevant to long-term experiments is MIAPPE.
MIAPPE
MIAPPE stands for minimum information about a plant phenotyping experiment and is designed to harmonise the description of experimental and computed data from field and glass house experiments.
Key Points
- Metadata provides essential context for understanding a dataset
- To be reusable, metadata should be consistent across datasets of the same type
Content from Organising data for long-term experiments
Last updated on 2024-12-03 | Edit this page
Overview
Questions
- What are the principles for using spreadsheets for good data organisation?
Objectives
- Learn to recognise good and bad practice for managing tabular data in spreadsheet programs.
- Understand how good table structure helps to re-use data.
Introduction
Tables are a great way of presenting data, and spreadsheet software such as Google Sheets and Microsoft Excel are useful programs for making tabular datasets. Most researchers are familiar with Excel, it is easy to use, flexible, and includes tools for analysing and visualising data. Excel’s flexibility gives us a lot of freedom to organise data, but this can lead to bad practices which make data difficult to reuse.
In the worst case Excel’s default behavior may corrupt our data. For example consider a value like 1/12, or SEPT1. In both examples Excel will, by default, convert these values to dates, even if they are not intended by the user to be dates. In the example of SEPT1, this was a recognised name for a human gene which has since been renamed to SEPTIN1 specifically to avoid this type of data handling error when using Excel (https://doi.org/10.1038/s41588-020-0669-3).
Bad tables in Excel
Using the data tables in the lesson Excel files, review them to identify any problems with that would make it difficult to reuse the data
- What do you find confusing about the data?
- Do you have all the information you need to use the data?
- Could you successfully combine the data?
- Plot names would be converted to dates in Excel.
- Treatments, FYM treatments are encoded using colour, and they key is not obvious.
- Yield does not provide units.
- Some yield values are coloured red, but not clear why.
- Data is split into multiple tables which would be difficult to combine and use.
Problems with the Sidada dataset
- Multi-row column headings.
- Matrix style layout using variables (year and season) as column headings.
- Just a table, no metadata to describe the dataset so not even sure what the data is.
Problems with the Chitedze dataset
- Pretty layout – try saving as CSV, mix of data and metadata on one sheet.
- Headings span multiple columns.
- Multiple tables in the same worksheet.
- Use of undefined codes.
- Missing data flagged with -9999.
Problems with the Embu dataset
- Data is split across multiple worksheet tabs
- Inconsistent data: First 3 years have a space between OM and N, but not in subsequent years
- Inconsistent data: First 3 years OM and N rates are blank when not applied, but after are 0.
- Inconsistent data: Yield has missing values which are flagged * years 1-3 and N/A years 4-10
- Inconsistent data: OM types are inconsistently named
C. calothyrsus cuttings
,Calliandra cuttings
, andCalliandra cuttings
- Inconsistent data: Yield combines units and value, should be separate
- Inconsistent data: Yield has different units – bushels/acre and t/ha
Common Spreadsheet Errors
There are several well known errors researchers make when using Excel. This section provides an overview of them.
1. Multiple tables in one spreadsheet
The Broadbalk example dataset has its data organised into separate tables for each year in one worksheet. While this view makes it visually easy for a human to view all the data on one screen, it makes it much harder for a computer to process the data. The computer does not recognise row 6 contains is for five different tears and column C includes both treatment codes, sowing and harvest dates for two differet years. If we wanted to calculate mean yields per treatment we would need to reorganise the data or manually reference cells in an Excel formula.
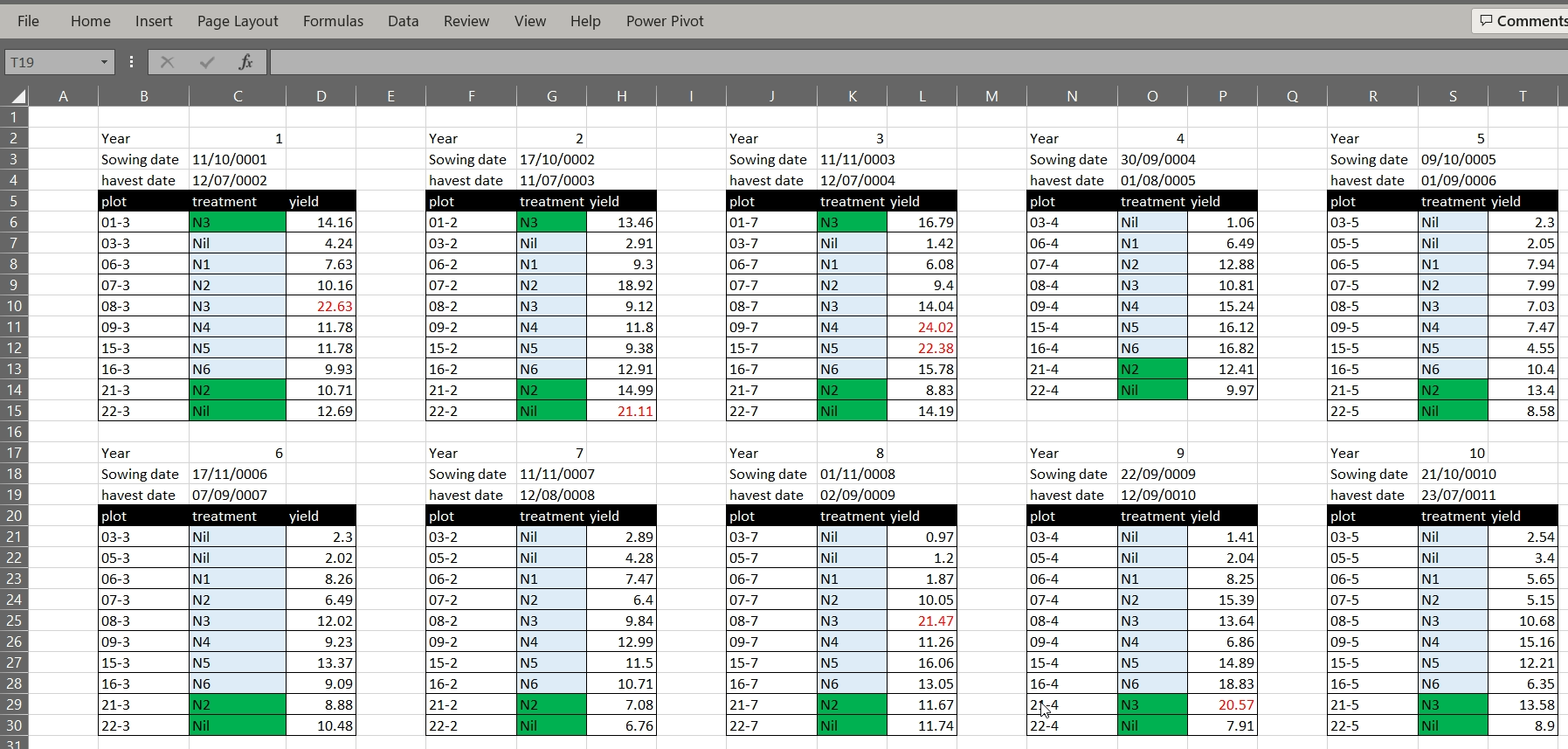
2. Using multiple tabs
Creating multiple tabs can improve data organisation by helping to separate different pieces of information. For example, in the Embu example dataset, the yield data is in a separate worksheet tab to the experiment information. However, with Excel, the computer will not be able to see connections which might exist between data in different tabs, for example, in the Embu example, the yield data is split into a separate worksheet per year, and while a human can see these data are related the computer cannot. Furthermore, key information, namely the year of the experiment is encoded in the worksheet name only. As with placing data in multiple tables in the same worksheet, placing data in multiple worksheets means you must either reorganise the data or write more data handling scripts before you can use the data.
Using multiple worksheet tab can introduce inconsistencies in the way tables are organised. It is always better to keep related together in one table. Keeping related data in one table may result in a large table, but Excel does provide tools to make it easier to navigate the data such as freezing column headings and column filters.
If our spreadsheet has more than a few worksheet tabs, and you need to scroll through multiple tabs, it can become difficult and unwieldy to keep track of all the information.
3. Zeros, blanks and null values
A cell with a value 0 is different to a blank cell. To Excel, a blank cell means no data, whereas zero is data. Zero means you looked for something but did not observe it while blank is an absence of data. Excel and statistical programs may misrepresent blanks that should be zeros in subsequent analyses
Very often we’ll encounter datasets where missing data is encoded by a specific value, for example, -999, 999, NA, or *. Using values to encode missing data can cause problems. For example a statistical program will not recognise a numeric code for missing data from real numeric data, and a non-numeric character may cause the values to be treated as text rather than numbers. Therefore it is generally better to leave missing values as blank, but if they are encoded, the table metadata must document this, and the code must be used consistently. One key exception to this rule is if you plan to use the statistical software R, where the value NA can be used to indicate missing values.
Sometimes we might know why a value is missing, and this might be for one of several reasons. For example, in an LTE, missing data might be because the crop failed to establish, it was destroyed by animal pests, or it was destroyed by disease. Rather than encoding this information in the column recording yield, it is better to add a separate column recording the reason why data is missing.
4. Using formatting to convey information
Formatting in Excel can include using different background colours for cells, using different colours for text, leaving blank rows or columns between data, or using border shading. For a human, formatting can be used to both make tables more readable and to convey information. Formatting to make a table more readable, for example, shading column headings, is acceptable, but using formatting to convey information must be avoided. Unlike a human, a computer cannot use formatting, and if the data is saved as a text format such as CSV or TAB, the information contained in the formatting is lost.
Rather than using formatting to convey information, the information should be encoded as a new field. For example, in the Broadbalk dataset, green is used to identify treatments with FYM, and red text to indicate a suspicious value, these pieced of information should be added as separate columns:
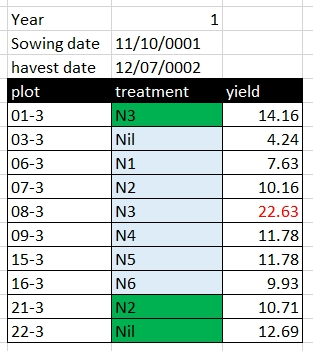
becomes
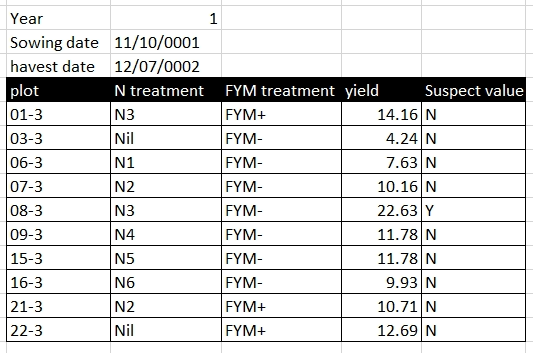
5. Storing more than one piece of information in a cell
Using formatting to convey information is an example of storing more than one piece of information in a cell, you have both the cell value and the information encoded by the formatting. In the Sidada example, we can see treatment seems to encode information about several different treatments. This makes it difficult, for example, to select records based on a single factor level, for example if we wanted to identify plots with the N+ treatment rather than filtering on an exact term we must use a fuzzy search and select plots containing or like N+. Equally we cannot easily order rows on this value.
In the Embu dataset, yield data for some years combines both the yield value and yield units. This problematic for analysis as a computer would treat the value as text instead of as a number. The unit information should either be stored in a separate column, or as metadata for the yield value column description.
6. Inconsistent values
Being inconsistent with value names can cause problems. For example,
in the Embu data, C. calothyrsus cuttings
,
Calliandra cuttings
, and Calliandra cuttings
are used interchangeably and, as humans we can see these terms, in the
context of the dataset, mean the same thing. A compuer, however, would
treat these as different values.
Being precise in how variables and values are named is essential for reliably interpreting the data.
7. Problematic field names
Column names should be descriptive of the data they hold. A brief descriptive name should be preferred as it removes ambiguity and better conveys the meaning of the data. You can provide more detail about the column data in a separate metadata file. Abbreviations may make sense now, but will they make sense in 6 months time or to other researchers unfamiliar with your naming convention? Abbreviations are best used only when they widely accepted within a research community.
When naming columns be careful to avoid white spaces, starting with numbers, or special characters. white space, tabs and commas can all be interpreted as column delimiters, and some software packages won’t accept column names with numbers. Underscores are a better alternative to white space. CamelCase (for example OrganicManureTreatment), can also be used, but may cause problems with software that treats column headings as case insensitive.
Good Name | Avoid Name |
---|---|
yield_tha | yield (t ha-1) |
organic_manure_type | OM type |
treatment_label | treatment label |
year_5 | 5th year |
8. multi-row column names and merge cells
Using multi-row column headings can be useful mechanism for grouping related columns together in a hierarchical naming structure. For example, in the Sidada dataset column headings are across two rows, with cells merged to illustrate the grouped columns. while this works for a human a computer will not recognise this structure, and interpret the second row as data. Merged cells may also be unmerged to create nameless columns, for example, we can see this behavior if we export the Sidada data as CSV:
Content from Making LTE data FAIR
Last updated on 2024-12-03 | Edit this page
Overview
Questions
- How do I make LTE data FAIR?
Objectives
- Understand how semantic annotation can improve data interoperability
- Learn to use online resources to identify appropriate resources to annotate data
Making LTE Data FAIR
In the previous two episodes we first learned how to improve the FAIRness of LTE metadata, then how to structure our data. In this section we will explore how to improve the FAIRness of our data by improving its interoperability and reusability.
Standardising the table structure using Tidy Data principles
Tidy data is an approach for organising tabular data intended to make it easier for an analyst or computer to extract data. In tidy data:
Each variable forms a column.
Each observation forms a row.
Each type of observational unit forms a table.
define a tidy data structure for the Broadbalk example dataset.
- What are the variables?
- What is the observation unit?
The variables are: plot, year, nitrogen treatment, organic manure treatment, sowing data, harvest date, and yield.
The observation unit is one plot in a given year.
Using open data formats
Open data formats are non proprietary text formats accessible to anyone. For tabular data, comma separated value (CSV) and tab delimited (TAB) are widely used formats.
Excel is a propriety format needing specialist software to open and use the data.
Describing the data table structure
Adding semantic annotation
Key Points
- Providing a well described table makes it easier for researchers to understand what data it contains.
- Standardising table structures and using open data formats make it easier to use the data in statistical packages.
- Semantic annotation allows different datasets to be combined on common concepts.
Content from databases-for-lte-data
Last updated on 2024-12-03 | Edit this page
Overview
Questions
- How are databases different from spreadsheets?
- Why are databases useful for managing LTE data
Objectives
- Understand the differences between databases and spreadsheets.
- Explain why databases may be a more useful tool for long-term LTE data management.
Excel is not a database
We commonly use Excel to manage data, but it is important to remember Excel is not a database management system. There are several key differences between how Excel, as a spreadsheet program, manages data compared to a database. How does data storage differ between spreadsheets and databases?
Unit of data
In a spreadsheet the unit of data is a cell, in a database it is a table record. If we create a table in Excel, the value in one column is independent from the value in the neighbouring cells. As a human we can see there is a relationship between cells in a row, but Excel does not share our concept of a table and will allow you to sort one column independently of other columns. Excel does not have a formal table structure, you can draw a table layout, but you can move the table around a worksheet, and place other information around it. In a database a table exists as a formal data structure and its unit of data is a row. A table can contain only data in the columns define for it and nothing else and columns cannot be sorted independently of each other.
Column data types
Database table columns are typed, in excel they are not. This means
in a database table you must define the data type for each column, for
example text
, integer
, numeric
,
date
, or datetime
(the exact datatypes will
vary between different database systems). Therefore a colmnn defined as
integer can only store integer values, it cannot store decimals, text or
dates. In Excel you can add any type of data into a cell. Databases
therefore provide very robust rules for quality controlling your data
compared to Excel.
Databases define formal relationships between tables
A database can define formal relationships between tables using key columns. This is a very powerful feature of relational databases which allow for complex data structures to be defined. An important use of relationships is to reduce data duplication and increase the integrity of the data. For example, the Chitedze dataset has eight different treatments. These treatments can be defined in one small table, and the unique treatment number copied into the yield data table to create a relationship. None of the information about treatments needs to be duplicated in the yield data table, and a rule known as referential integrity means only ID values from the treatments table can be used in the yield data table.
Databases live on database servers
Relational databases are usually hosted on a database server (SQLite and MS Access are two exceptions being file based programs). This brings several security features not available in Excel, including: - multi-user access - backup and recovery - permissioned access - multi-user access - audit logs
Databases are transactional
Every update to a database happens inside a transaction which has a start and an end. This means data is only saved into the database once the transaction successful completes, if something goes wrong, or you forget to finish, the transaction will rollback to the databases original state. This is a critical feature which helps to maintain the health of your data.
Databases are not easy to copy
Compared to Excel, it is very difficult to make multiple copies of a database. With Excel it is very easy to make multiple copies of a file which, unless a robust naming and versioning system is in place, can lead to confusion.
Databases are harder to use
While databases clearly have many advantages over Excel for managing data, this comes at a cost. To use a database effectively you need a good understanding of database design principles and SQL, the language used to interact with a database.
Key Points
- Use
.md
files for episodes when you want static content - Use
.Rmd
files for episodes when you need to generate output - Run
sandpaper::check_lesson()
to identify any issues with your lesson - Run
sandpaper::build_lesson()
to preview your lesson locally
Content from Publishing LTE data
Last updated on 2024-12-03 | Edit this page
Overview
Questions
- What do you need to do to publish data?
- Where should you publish data?
- How do I update published LTE data?
Objectives
- Explain what metadata needs to be collected before publishing data.
- Understand DataCite rules for publishing data.
Introduction
A data repository is a storage infrastructure designed to preserve research data to make it findable and accessible to other researchers.
What about project websites and cloud resources like Github or Dropbox
Basic project websites, without any data repository infrastructure are are not suitable for publishing datasets because dataset metadata cannot easily be indexed and searched.
Github is a version control platform original developed for code management, it is designed for uploaded content to be frequently updated, the opposite use of a repository.
Dropbox and similar services are cloud file hosting services.
None of these options have features such as PID allocation, versioning, or machine readable, indexed metadata, and therefore not suitable for publishing data.
Data Repository features
Features to support FAIR data
To support FAIR data, a data repository must provide a core set of features including: - Exposing machine readable metadata which can be indexed by services such as Google Dataset Search. - Infrastructure to capture rich and relevant metadata. - Ensuring access to metadata even if access to data is not available. - Allocation of persistent and citable identifiers (e.g. DOIs) to datasets.
Dataset DOIs
DOI stands for Domain Object Identifier and is a type of Persistent Identifier managed by DataCite. DataCite is a global non-profit organisation which manages the allocation of DOIs. A DOI consists of an identifier, a resolution URL and metadata. The resolution URL is the place on the internet where the data can be accessed. The two key advantages of a DOI compared to a simple web address are: 1. The DOI is permanent, DataCite guarantees it will resolve to a metadata landing page, even if the link to the data is broken. 2. Metadata to describe the dataset is always available, even if the dataset is not.
Review the DataCite Metadata Schema
Review the DataCite Metadata Schema to get a better understanding of the types of metadata it can record.
- How does the schema identify relationships between different research outputs?
Data versioning
Datasets published with a DOI are immutable, meaning you should not alter the data. For example, making a correction to a published dataset or appending new records means the data has changed and must therefore be republished with a new DataCite DOI. This is an important concept for supporting reproducible science as it guarantees all users of a dataset will use exactly the same data over time.
DataCite DOIs provide a mechanism for both versioning datasets and identifying relationships between two datasets, in this case stating Dataset X is a newer version of Dataset Y.
Versioning LTE data
LTEs accumulate new data overtime, meaning the data we publish now will be updated repeatedly in the future. There are three strategies for publishing data.
- Defined period publishing cycle.
- New published dataset.
- Dynamic citation publishing.
What about sites like Github or Dropbox
Github is a version control platform original developed for code management. Dropbox and similar services are cloud file hosting services. Neither type of service provides repository features such as PID, and indexed and findable metadata.
Features to improve research experience
Data repositories often provide other features to improve researcher experience including: - Embargo periods, enabling dataset discovery, but not immediate access. - Reporting metrics such as views, downloads, citations and other interactions. - Analytical pipelines. Normally only found in specialist data repositories which have rigorous submission pipelines checking data quality and host similar data types.
Specialist vs Generalist repositories
In several domains specialist data repositories have been developed to better serve particular research communities and the types of data they generate. Many research institutes will have their own data repositories. Institute repositories are normally generalist but may have local policies and support to improve data submission quality. The following table compares specialist, generalist and institute repositories:
Feature | Specialist | Generalist | Institute |
---|---|---|---|
Identifier | Unique repository accession number, DataCite DOI. | DataCite DOI | DataCite DOI |
Metadata | Detailed metadata to describe the type of data and or domain. | Administrative metadata using DataCite schema. | Administrative metadata using DataCite schema, but may have local policies to improve metadata quality. |
Data format | Proscribed data format using an open community standard. | No special rules or quality controls, but may make recommendations. | May have local policies on data formats. |
Support | May provide data curation and submission support. | Basic. | May have Research Data Stewards to support. |
Data Quality Control | Often have strict requirements. | Minimal to none. | Depends on local policy. |
Relationship to repository data | Good | None | Aligned to institute research areas. |
Tools integration | May feed directly into analytical pipelines for processing the repository data types. | None | Some open source repositories (CKAN, Dataverse) support cross dataset querying for similarly structured tabular data. |
Key Points
- Data repositories provide features supporting FAIR data.
- Published datasets are immutable - the data must not be altered.
- Published datasets are versioned.